From PIace2Vec to Multi-Scale Built-Environment Representation: A General-Purpose Distributional Embedding for Urban Data Analysis
SIGSPATIAL '20: 28th International Conference on Advances in Geographic Information Systems Seattle WA USA November, 2020(2020)
摘要
Built environments like cities, roads, communities are rich sources of urban data. Many downstream applications require comprehensive analysis like geographic information retrieval, recommender systems, geographic knowledge graphs, and in general, understanding urban spaces [28]. Points of Interests (POI), as one of the most researched aspects of urban data, has been successfully modeled using concepts borrowed from Machine Learning (ML) and Natural Language Processing (NLP). In the work of Place2Vec [28], a Word2Vec-like statistical model is proposed to represent spatial adjacency with a continuous embedding space. This method successfully models the functional semantics of POIs with regard to several human-assessment based evaluations. However, though the Place2Vec model addresses the distributional heterogeneity within a given spatial context with ITDL augmentation, it does not address the spatial heterogeneity among different regions. To solve this problem, we propose to introduce a hierarchical, density-based, self-adjusting clustering mechanism. The boundary of relatedness and unrelatedness is learned from the given context, where denser areas have tighter bounds while sparser areas have looser ones. We train our model on both the baseline Yelp hierarchical dataset [28] and our OpenStreetMap dataset. We demonstrate that 1) our model significantly improves the performance on 2 of the 3 baseline tasks and the stability of training, and 2) our model generalizes excellently across 112 cities of radically different scales (minimum 1725 POIs, maximum 2694070 POIs), regions (North America, Europe, Asia, Africa) and types (commercial, touristy, industrial, etc.) without the need of adjusting or tuning any hyperparameters. We also demonstrate that our model can be used to discover interesting facts about cities like inter-city semantic analogy and intra-city connectivity, which can be very useful in urban planning, social computing and public policy making.
更多查看译文
AI 理解论文
溯源树
样例
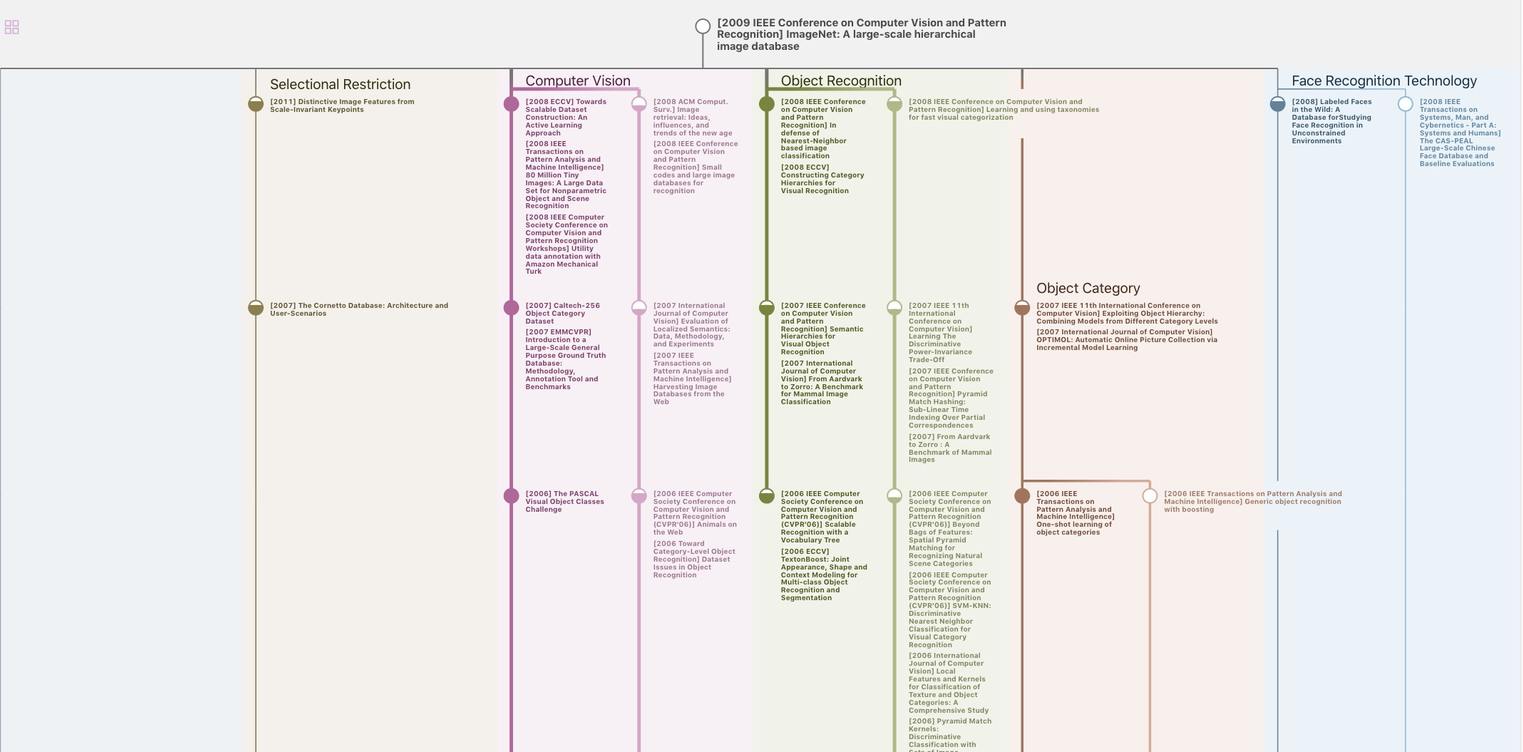
生成溯源树,研究论文发展脉络
Chat Paper
正在生成论文摘要