CTNN: A Convolutional Tensor-Train Neural Network for Multi-Task Brainprint Recognition
IEEE Transactions on Neural Systems and Rehabilitation Engineering(2021)
摘要
Brainprint is a new type of biometric in the form of EEG, directly linking to intrinsic identity. Currently, most methods for brainprint recognition are based on traditional machine learning and only focus on a single brain cognition task. Due to the ability to extract high-level features and latent dependencies, deep learning can effectively overcome the limitation of specific tasks, but numerous samples are required for model training. Therefore, brainprint recognition in realistic scenes with multiple individuals and small amounts of samples in each class is challenging for deep learning. This article proposes a Convolutional Tensor-Train Neural Network (CTNN) for the multi-task brainprint recognition with small number of training samples. Firstly, local temporal and spatial features of the brainprint are extracted by the convolutional neural network (CNN) with depthwise separable convolution mechanism. Afterwards, we implement the TensorNet (TN) via low-rank representation to capture the multilinear intercorrelations, which integrates the local information into a global one with very limited parameters. The experimental results indicate that CTNN has high recognition accuracy over 99% on all four datasets, and it exploits brainprint under multi-task efficiently and scales well on training samples. Additionally, our method can also provide an interpretable biomarker, which shows specific seven channels are dominated for the recognition tasks.
更多查看译文
关键词
Brain,Humans,Machine Learning,Neural Networks, Computer
AI 理解论文
溯源树
样例
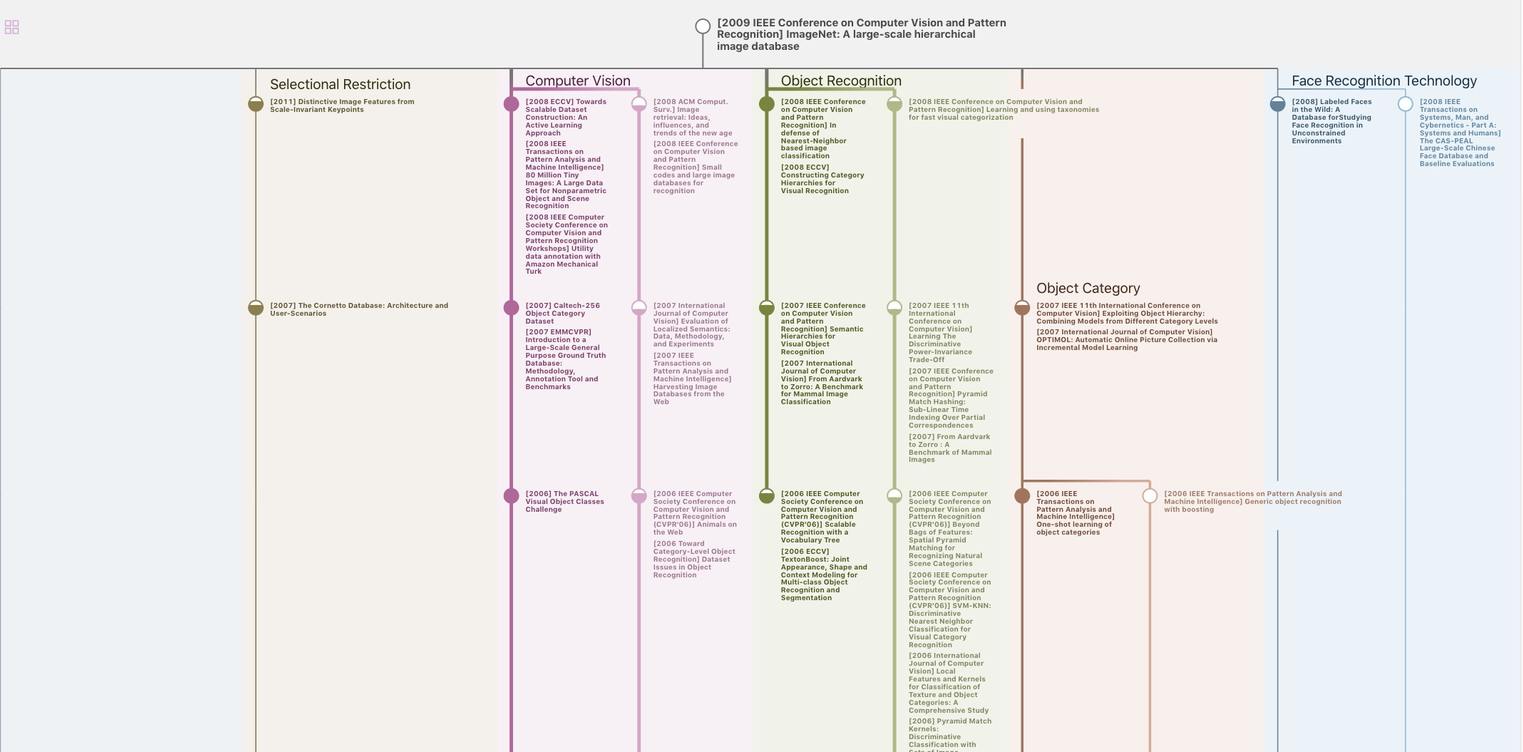
生成溯源树,研究论文发展脉络
Chat Paper
正在生成论文摘要