SMALL FOOTPRINT TEXT-INDEPENDENT SPEAKER VERIFICATION FOR EMBEDDED SYSTEMS
2021 IEEE INTERNATIONAL CONFERENCE ON ACOUSTICS, SPEECH AND SIGNAL PROCESSING (ICASSP 2021)(2021)
摘要
Deep neural network approaches to speaker verification have proven successful, but typical computational requirements of State-Of-The-Art (SOTA) systems make them unsuited for embedded applications. In this work, we present a two-stage model architecture orders of magnitude smaller than common solutions (237.5K learning parameters, 11.5MFLOPS) reaching a competitive result of 3.31% Equal Error Rate (EER) on the well established VoxCeleb1 verification test set. We demonstrate the possibility of running our solution on small devices typical of IoT systems such as the Raspberry Pi 3B with a latency smaller than 200ms on a 5s long utterance. Additionally, we evaluate our model on the acoustically challenging VOiCES corpus. We report a limited increase in EER of 2.6 percentage points with respect to the best scoring model of the 2019 VOiCES from a Distance Challenge, against a reduction of 25.6 times in the number of learning parameters.
更多查看译文
关键词
speaker verification, neural networks, text independent, small footprint
AI 理解论文
溯源树
样例
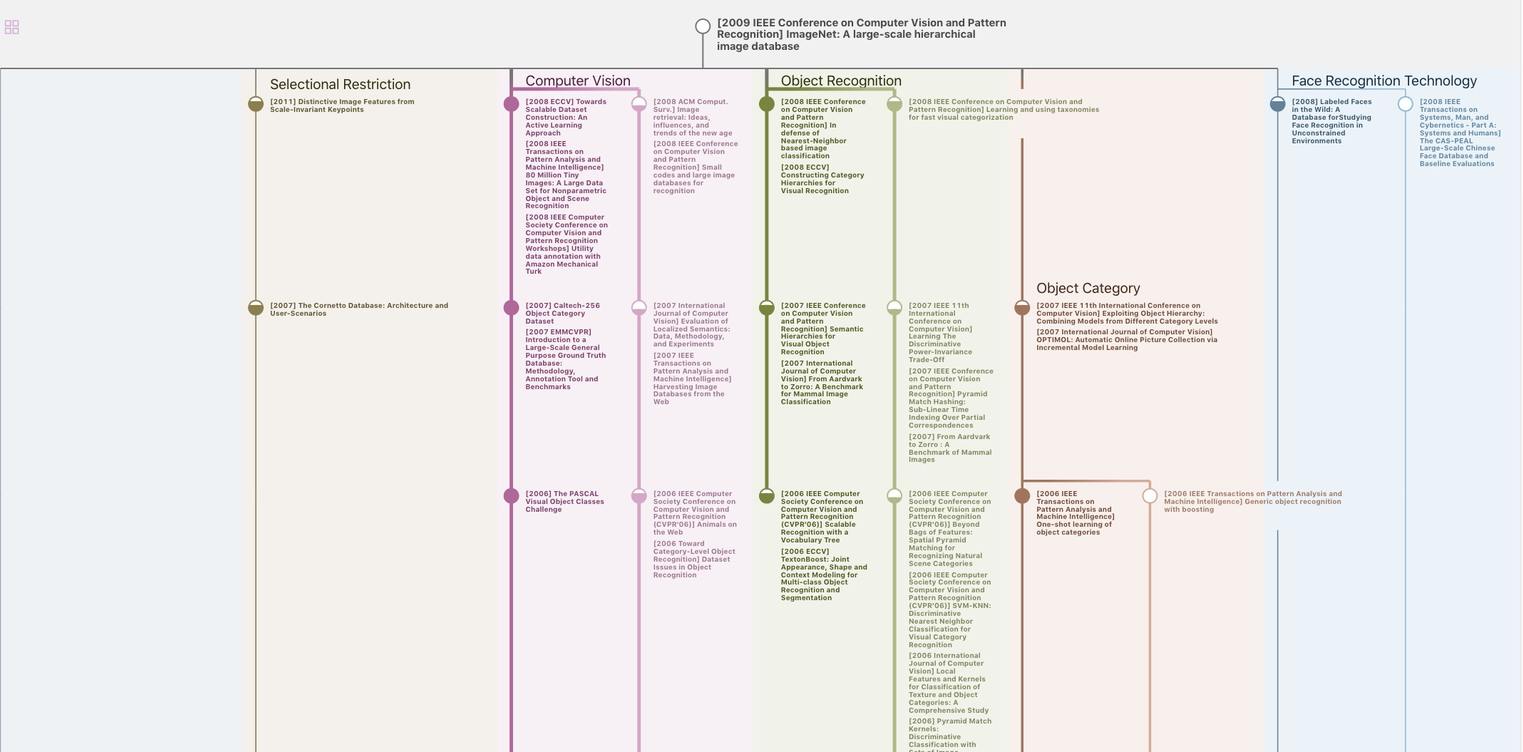
生成溯源树,研究论文发展脉络
Chat Paper
正在生成论文摘要