Penetrating RF Fingerprinting-based Authentication with a Generative Adversarial Attack
IEEE INTERNATIONAL CONFERENCE ON COMMUNICATIONS (ICC 2021)(2021)
摘要
Physical layer authentication relies on detecting unique imperfections in signals transmitted by radio devices to isolate their fingerprint. Recently, deep learning-based authenticators have increasingly been proposed to classify devices using these fingerprints, as they achieve higher accuracies compared to traditional approaches. However, it has been shown in domains such as computer vision that adding carefully crafted perturbations to legitimate inputs can fool such classifiers. This can undermine the security provided by the authenticator. Unlike adversarial attacks applied in other domains, an adversary has no control over the propagation environment. Therefore, to investigate the severity of this type of attack in wireless communications, we consider an unauthorized transmitter attempting to have its signals classified as authorized by a deep learning-based authenticator. We demonstrate a reinforcement learning-based attack where the impersonator-using only the authenticator's binary authentication decision-distorts its signals in order to penetrate the system. Extensive simulations and experiments on a software-defined radio testbed indicate that at appropriate channel conditions and bounded by a maximum distortion level, it is possible to fool the authenticator reliably at a success rate of more than 90%.
更多查看译文
关键词
Transmitter Identification, Deep Learning, Open set recognition, authorization, physical layer authentication
AI 理解论文
溯源树
样例
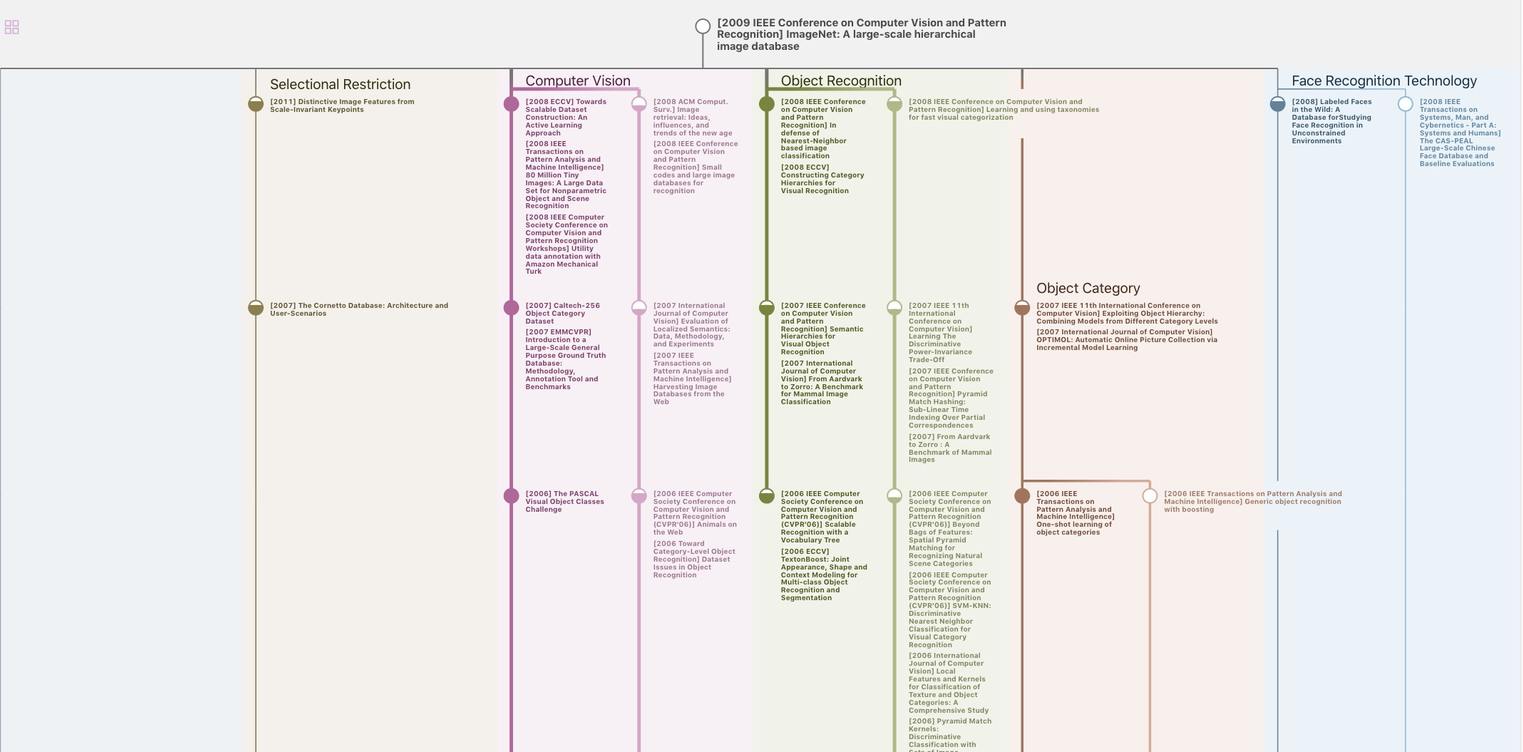
生成溯源树,研究论文发展脉络
Chat Paper
正在生成论文摘要