Frequency-compensated PINNs for Fluid-dynamic Design Problems
arxiv(2020)
摘要
Incompressible fluid flow around a cylinder is one of the classical problems in fluid-dynamics with strong relevance with many real-world engineering problems, for example, design of offshore structures or design of a pin-fin heat exchanger. Thus learning a high-accuracy surrogate for this problem can demonstrate the efficacy of a novel machine learning approach. In this work, we propose a physics-informed neural network (PINN) architecture for learning the relationship between simulation output and the underlying geometry and boundary conditions. In addition to using a physics-based regularization term, the proposed approach also exploits the underlying physics to learn a set of Fourier features, i.e. frequency and phase offset parameters, and then use them for predicting flow velocity and pressure over the spatio-temporal domain. We demonstrate this approach by predicting simulation results over out of range time interval and for novel design conditions. Our results show that incorporation of Fourier features improves the generalization performance over both temporal domain and design space.
更多查看译文
关键词
pinns,frequency-compensated,fluid-dynamic
AI 理解论文
溯源树
样例
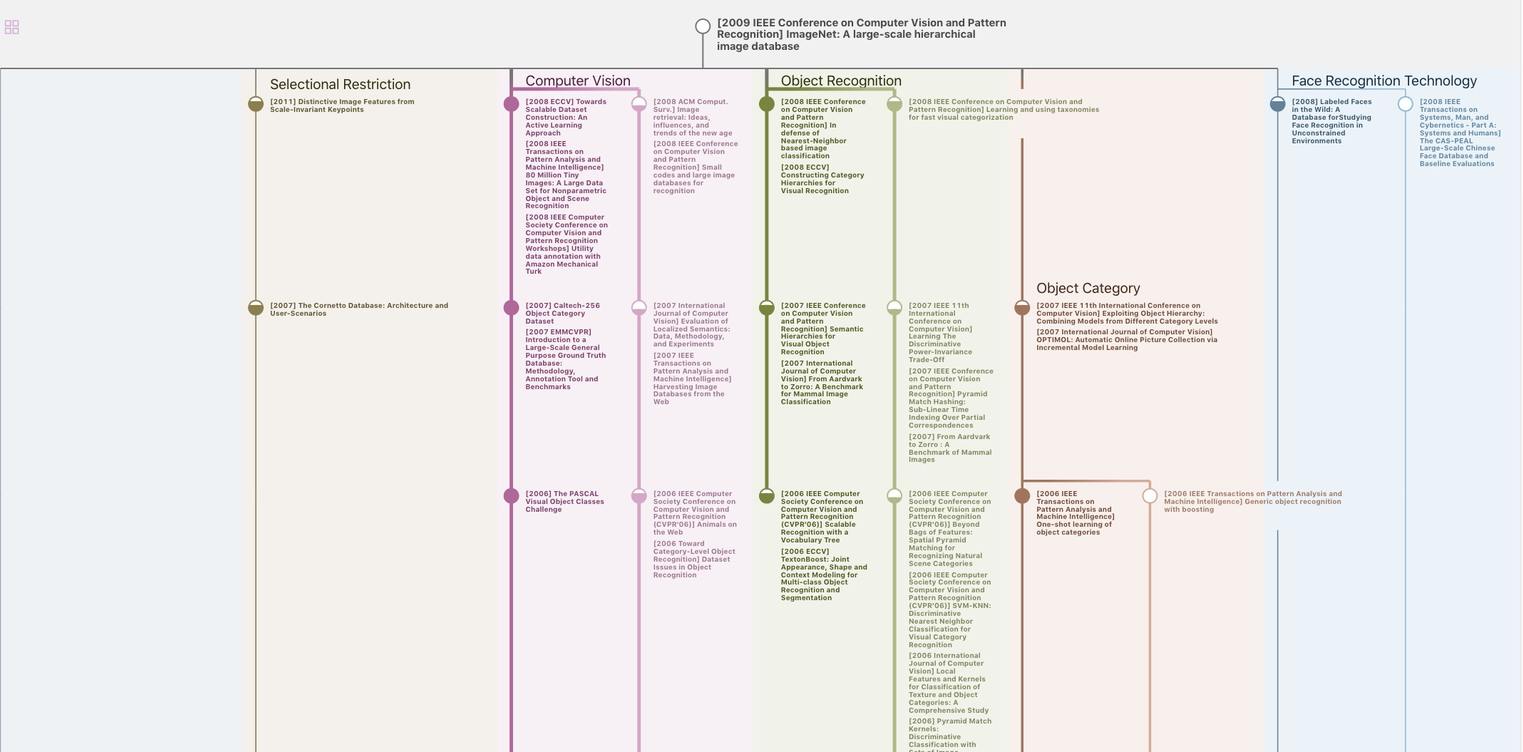
生成溯源树,研究论文发展脉络
Chat Paper
正在生成论文摘要