RRScell method for automated learning immune cell phenotypes with immunofluorescence cancer tissue
arXiv (Cornell University)(2020)
摘要
Multiplexed immunofluorescence tissue imaging enables precise spatial assessment of protein expression in medical resection specimens. However, tissue sections are stained with a mixture of antibodies, DNA and RNA markers, the detection of weak or broken edges due to fluorescent membrane staining artifacts between touching or overlapping cells is a long term studied problem, and is an active research topic in biomedical image analysis. Sometimes detecting these kinds of edges which are even lacking discrimination or judgment by human visual intelligence. We have built a GPU client-server and have developed a hybrid system combining the stochastic random-reaction-seed (RRS) method and deep neural learning U-net to identify cell-membrane accurately and automatically. Furthermore, we have designed a high performance machine-learning AI-pipeline in quantifying spatial distribution of cell phenotypes from tissue images with various complexities, and extract single-cell or even subcellular resolution profiling-map of protein and RNA expression over a million cells tissue section.
更多查看译文
关键词
immune rrscell phenotypes,cancer,learning
AI 理解论文
溯源树
样例
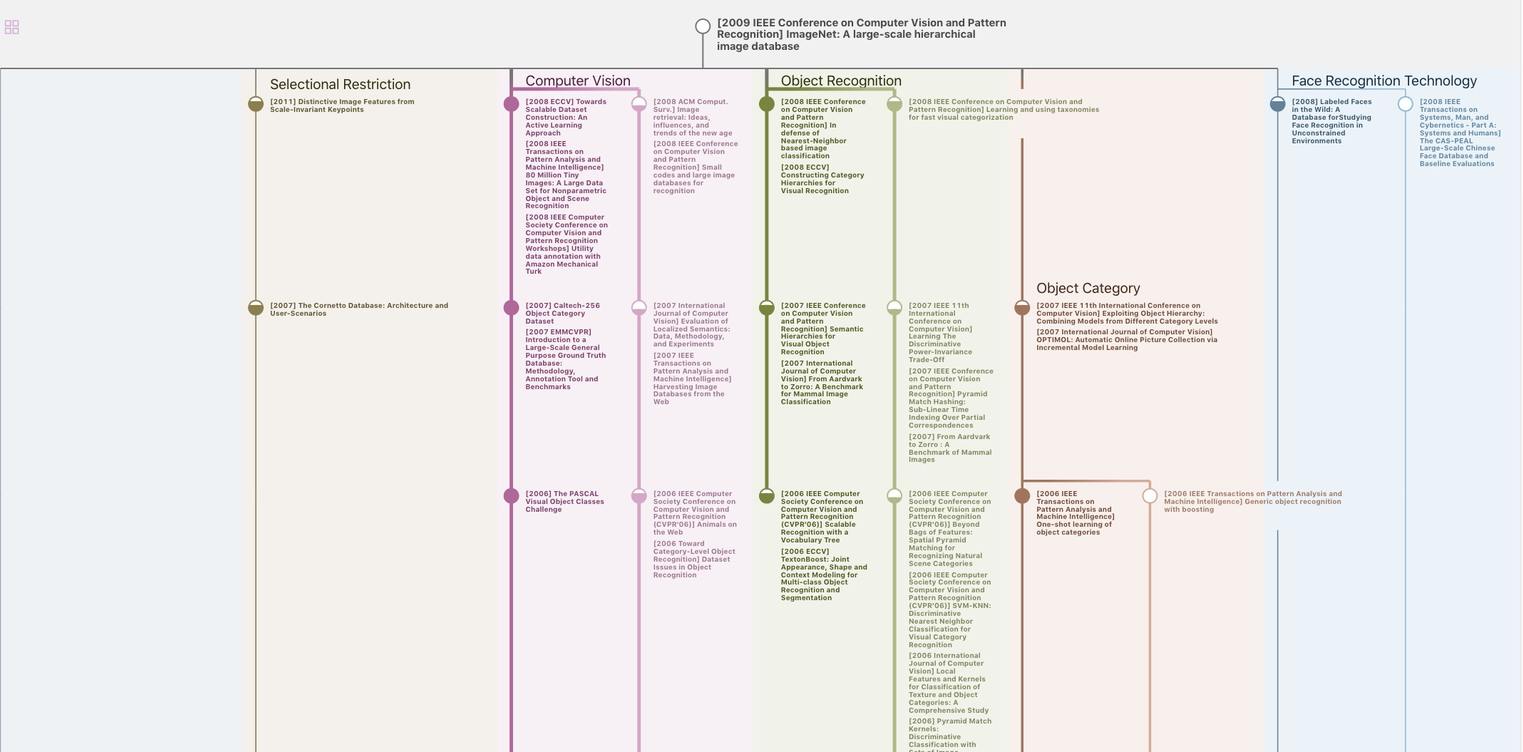
生成溯源树,研究论文发展脉络
Chat Paper
正在生成论文摘要