Maximum Likelihood Estimation in Data-Driven Modeling and Control
IEEE Transactions on Automatic Control(2023)
摘要
Recently, various algorithms for data-driven simulation and control have been proposed based on the Willems’ fundamental lemma. However, when collected data are noisy, these methods lead to ill-conditioned data-driven model structures. In this article, we present a maximum likelihood framework to obtain an optimal data-driven model, the signal matrix model, in the presence of output noise. Data compression and noise-level estimation schemes are also proposed to apply the algorithm efficiently to large datasets and unknown noise-level scenarios. Two approaches in system identification and receding horizon control are developed based on the derived optimal estimator. The first one identifies a finite impulse response model. This approach improves the least-squares estimator with less restrictive assumptions. The second one applies the signal matrix model as the predictor in predictive control. The control performance is shown to be better than existing data-driven predictive control algorithms, especially under high noise levels. Both approaches demonstrate that the derived estimator provides a promising framework to apply data-driven algorithms to noisy data.
更多查看译文
关键词
Data-driven modeling,maximum likelihood estimation (MLE),model-predictive control (MPC),system identification
AI 理解论文
溯源树
样例
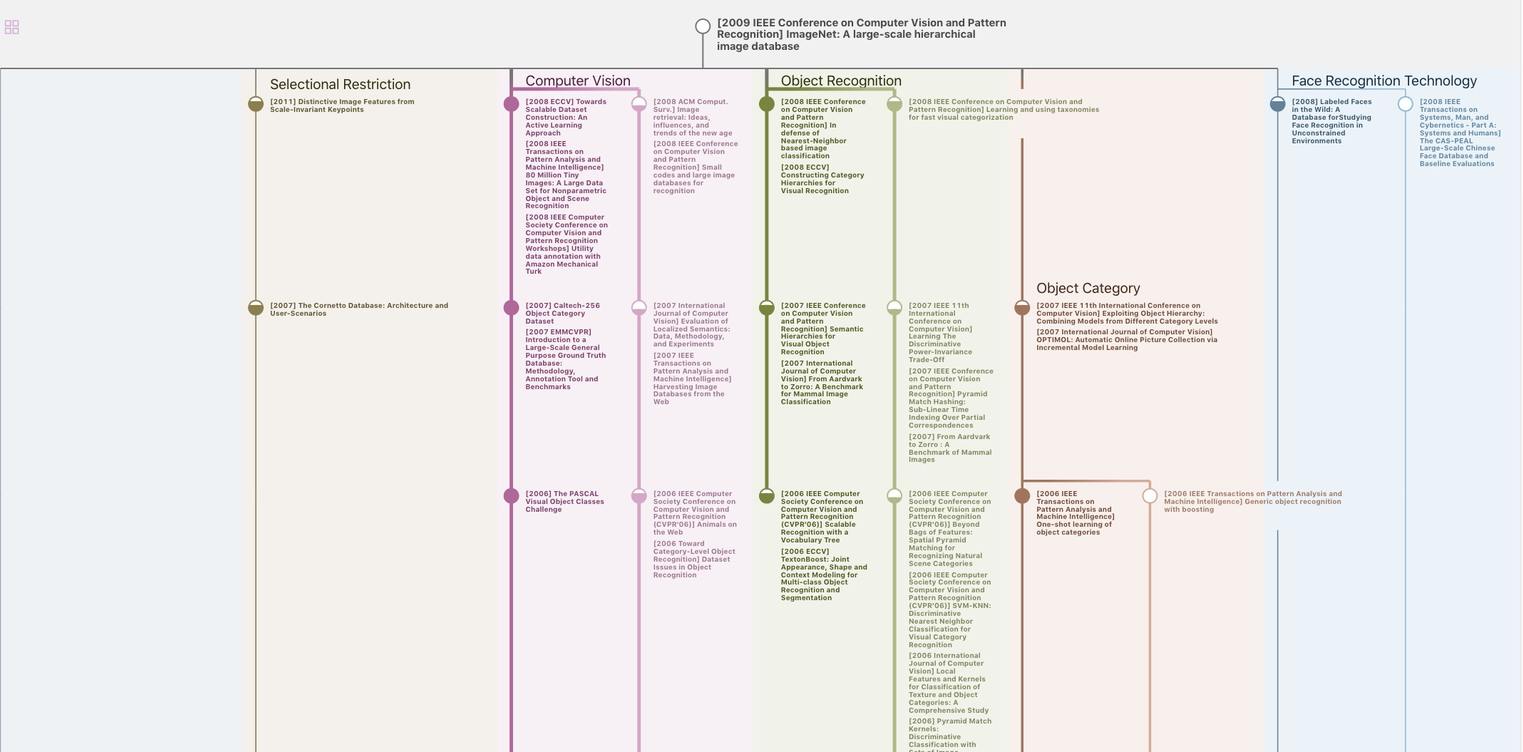
生成溯源树,研究论文发展脉络
Chat Paper
正在生成论文摘要