Robust Approximation of the Stochastic Koopman Operator\ast
arxiv(2022)
摘要
We analyze the performance of dynamic mode decomposition (DMD)-based approximations of the stochastic Koopman operator for random dynamical systems where either the dynamics or observ-ables are affected by noise. For many DMD algorithms, the presence of noise can introduce a bias in the DMD operator, leading to poor approximations of the dynamics. In particular, methods using time delayed observables, such as Hankel DMD, are biased when the dynamics are random. We introduce a new, robust DMD algorithm that can approximate the stochastic Koopman operator de-spite the presence of noise. We then demonstrate how this algorithm can be applied to time delayed observables, which allows us to generate a Krylov subspace from a single observable. This allows us to compute a realization of the stochastic Koopman operator using a single observable measured over a single trajectory. We test the performance of the algorithms over several examples.
更多查看译文
关键词
Koopman operator theory, random dynamical systems, dynamic mode decomposition
AI 理解论文
溯源树
样例
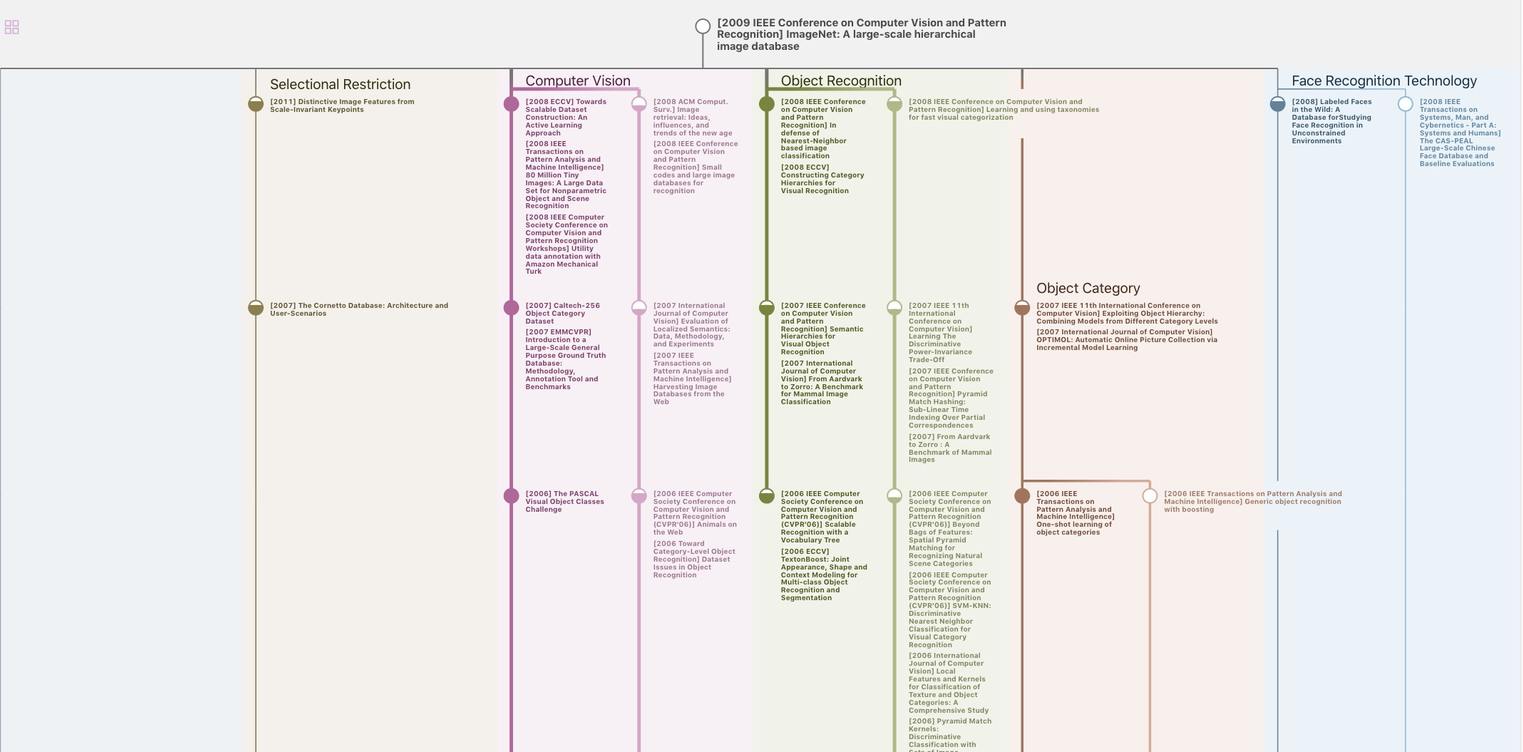
生成溯源树,研究论文发展脉络
Chat Paper
正在生成论文摘要