Spatiotemporal expansion of human brucellosis in Shaanxi Province, Northwestern China and model for risk prediction.
PEERJ(2020)
摘要
Background. Human brucellosis imposes a heavy burden on the health and economy of endemic regions. Since 2011, China has reported at least 35,000 human brucellosis cases annually, with more than 90% of these cases reported in the northern. Given the alarmingly high incidence and variation in the geographical distribution of human brucellosis cases, there is an urgent need to decipher the causes of such variation in geographical distribution. Method. We conducted a retrospective epidemiological study in Shaanxi Province from January 1, 2005 to December 31, 2018 to investigate the association between meteorological factors and transmission of human brucellosis according to differences in geographical distribution and seasonal fluctuation in northwestern China for the first time. Results. Human brucellosis cases were mainly distributed in the Shaanbei upland plateau before 2008 and then slowly extended towards the southern region with significant seasonal fluctuation. The results of quasi-Poisson generalized additive mixed model (GAMM) indicated that air temperature, sunshine duration, rainfall, relative humidity, and evaporation with maximum lag time within 7 months played crucial roles in the transmission of human brucellosis with seasonal fluctuation. Compared with the Shaanbei upland plateau, Guanzhong basin had more obvious fluctuations in the occurrence of human brucellosis due to changes in meteorological factors. Additionally, the established GAMM model showed high accuracy in predicting the occurrence of human brucellosis based on the meteorological factors. Conclusion. These findings may be used to predict the seasonal fluctuations of human brucellosis and to develop reliable and cost-effective prevention strategies in Shaanxi Province and other areas with similar environmental conditions.
更多查看译文
关键词
Human brucellosis,Spatiotemporal expansion,Generalized additive mixed model,Regional differences,Meteorological factors
AI 理解论文
溯源树
样例
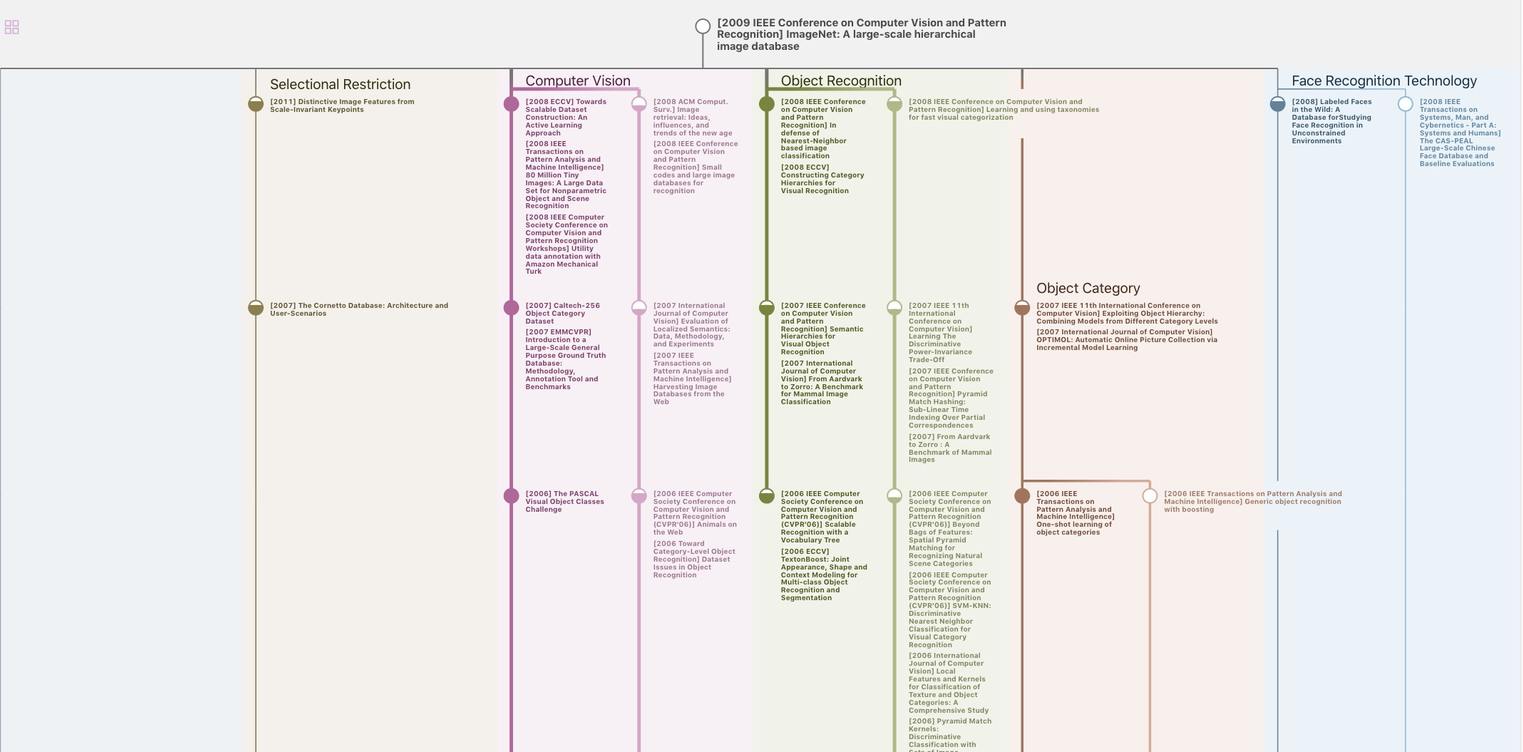
生成溯源树,研究论文发展脉络
Chat Paper
正在生成论文摘要