PHONEME BASED NEURAL TRANSDUCER FOR LARGE VOCABULARY SPEECH RECOGNITION
2021 IEEE INTERNATIONAL CONFERENCE ON ACOUSTICS, SPEECH AND SIGNAL PROCESSING (ICASSP 2021)(2021)
Abstract
To join the advantages of classical and end-to-end approaches for speech recognition, we present a simple, novel and competitive approach for phoneme-based neural transducer modeling. Different alignment label topologies are compared and word-end-based phoneme label augmentation is proposed to improve performance. Utilizing the local dependency of phonemes, we adopt a simplified neural network structure and a straightforward integration with the external word-level language model to preserve the consistency of seq-to-seq modeling. We also present a simple, stable and efficient training procedure using frame-wise cross-entropy loss. A phonetic context size of one is shown to be sufficient for the best performance. A simplified scheduled sampling approach is applied for further improvement and different decoding approaches are briefly compared. The overall performance of our best model is comparable to state-of-the-art (SOTA) results for the TED-LIUM Release 2 and Switchboard corpora.
MoreTranslated text
Key words
phoneme, neural transducer, speech recognition
AI Read Science
Must-Reading Tree
Example
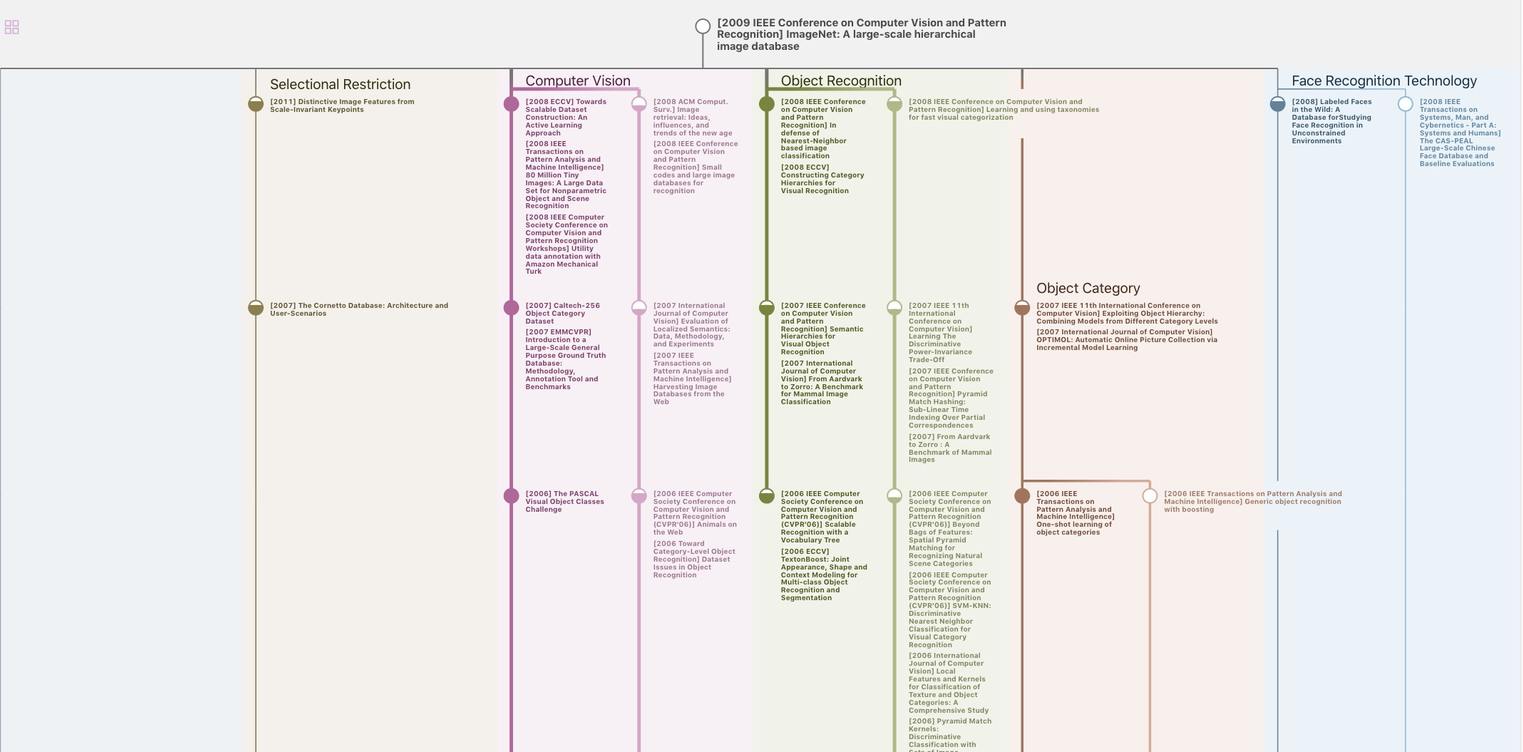
Generate MRT to find the research sequence of this paper
Chat Paper
Summary is being generated by the instructions you defined