HHAR-net: Hierarchical Human Activity Recognition using Neural Networks
international conference on interaction design & international development(2020)
摘要
Activity recognition using built-in sensors in smart and wearable devices provides great opportunities to understand and detect human behavior in the wild and gives a more holistic view of individuals\u0027 health and well being. Numerous computational methods have been applied to sensor streams to recognize different daily activities. However, most methods are unable to capture different layers of activities concealed in human behavior. Also, the performance of the models starts to decrease with increasing the number of activities. This research aims at building a hierarchical classification with Neural Networks to recognize human activities based on different levels of abstraction. We evaluate our model on the Extrasensory dataset; a dataset collected in the wild and containing data from smartphones and smartwatches. We use a two-level hierarchy with a total of six mutually exclusive labels namely, \"lying down\", \"sitting\", \"standing in place\", \"walking\", \"running\", and \"bicycling\" divided into \"stationary\" and \"non-stationary\". The results show that our model can recognize low-level activities (stationary/non-stationary) with 95.8% accuracy and overall accuracy of 92.8% over six labels. This is 3% above our best performing baseline.
更多查看译文
关键词
Deep learning,Human Activity Recognition,Hierarchical classification
AI 理解论文
溯源树
样例
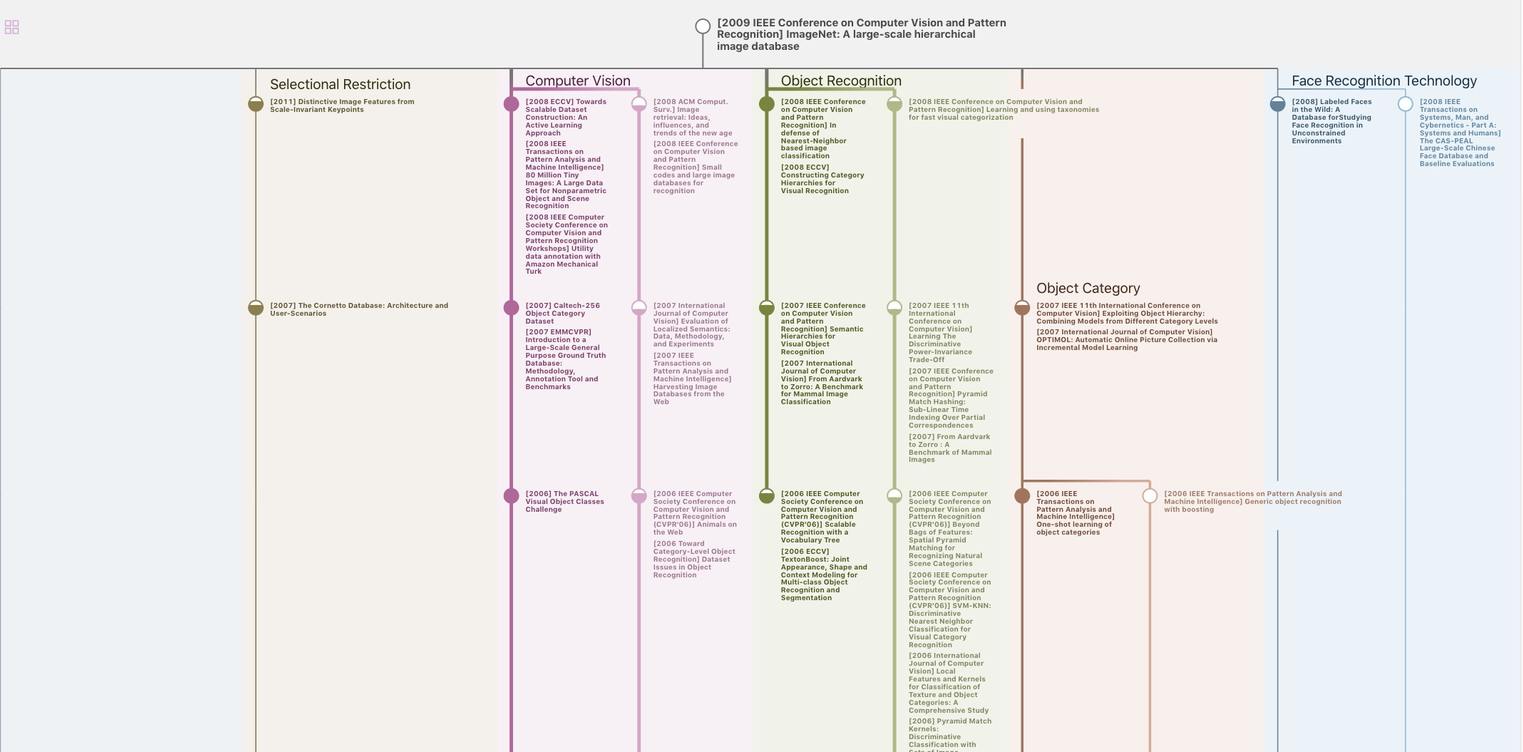
生成溯源树,研究论文发展脉络
Chat Paper
正在生成论文摘要