Development of a Clinical Prediction Model for In-hospital Mortality from the South African Cohort of the African Surgical Outcomes Study
WORLD JOURNAL OF SURGERY(2020)
Abstract
Background Data on the factors that influence mortality after surgery in South Africa are scarce, and neither these data nor data on risk-adjusted in-hospital mortality after surgery are routinely collected. Predictors related to the context or setting of surgical care delivery may also provide insight into variation in practice. Variation must be addressed when planning for improvement of risk-adjusted outcomes. Our objective was to identify the factors predicting in-hospital mortality after surgery in South Africa from available data. Methods A multivariable logistic regression model was developed to identify predictors of 30-day in-hospital mortality in surgical patients in South Africa. Data from the South African contribution to the African Surgical Outcomes Study were used and included 3800 cases from 51 hospitals. A forward stepwise regression technique was then employed to select for possible predictors prior to model specification. Model performance was evaluated by assessing calibration and discrimination. The South African Surgical Outcomes Study cohort was used to validate the model. Results Variables found to predict 30-day in-hospital mortality were age, American Society of Anesthesiologists Physical Status category, urgent or emergent surgery, major surgery, and gastrointestinal-, head and neck-, thoracic- and neurosurgery. The area under the receiver operating curve or c-statistic was 0.859 (95% confidence interval: 0.827–0.892) for the full model. Calibration, as assessed using a calibration plot, was acceptable. Performance was similar in the validation cohort as compared to the derivation cohort. Conclusion The prediction model did not include factors that can explain how the context of care influences post-operative mortality in South Africa. It does, however, provide a basis for reporting risk-adjusted perioperative mortality rate in the future, and identifies the types of surgery to be prioritised in quality improvement projects at a local or national level.
MoreTranslated text
Key words
clinical prediction model,south african cohort,mortality,in-hospital
AI Read Science
Must-Reading Tree
Example
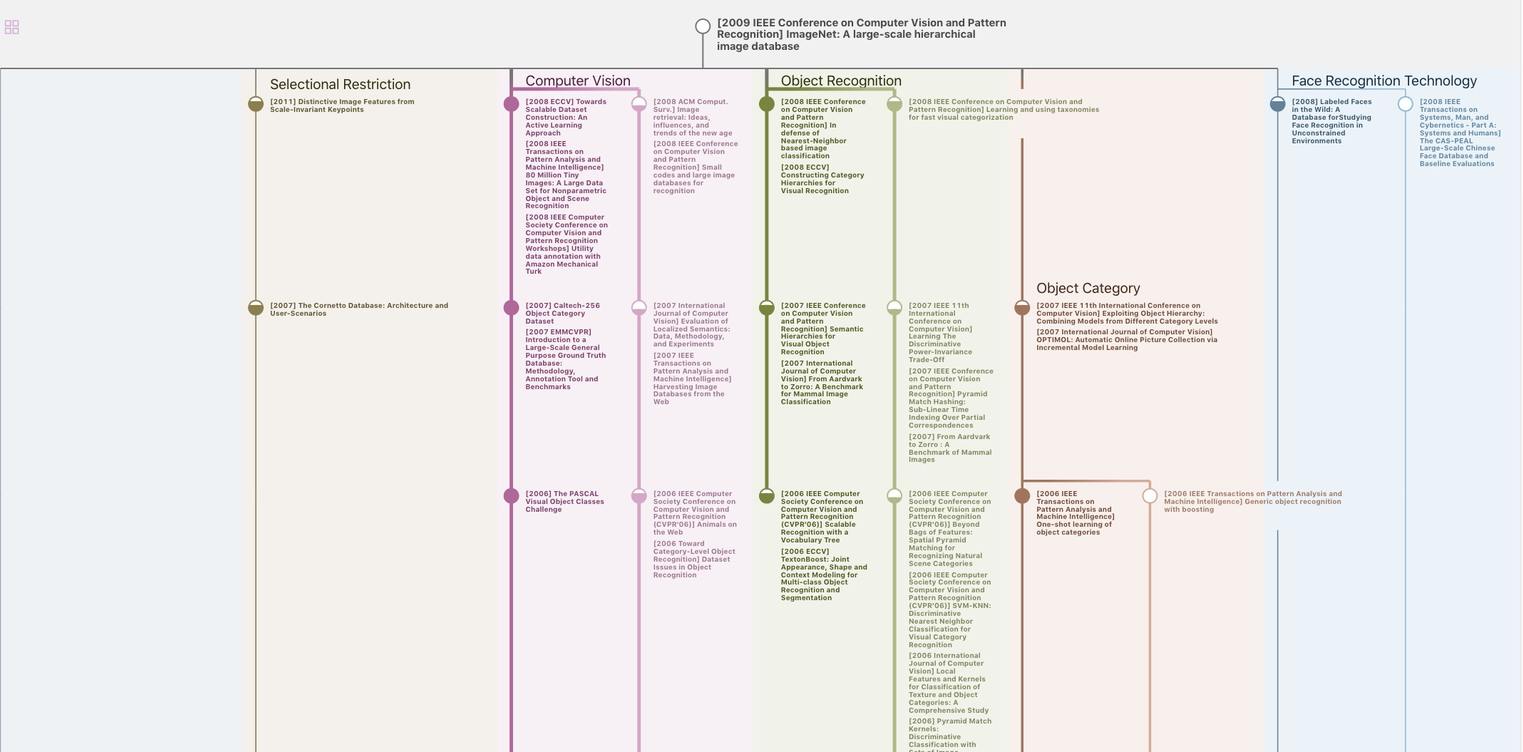
Generate MRT to find the research sequence of this paper
Chat Paper
Summary is being generated by the instructions you defined