An Evolutionary Orthogonal Component Analysis Method for Incremental Dimensionality Reduction
IEEE Transactions on Neural Networks and Learning Systems(2022)
摘要
In order to quickly discover the low-dimensional representation of high-dimensional noisy data in online environments, we transform the linear dimensionality reduction problem into the problem of learning the bases of linear feature subspaces. Based on that, we propose a fast and robust dimensionality reduction framework for incremental subspace learning named evolutionary orthogonal component analysis (EOCA). By setting adaptive thresholds to automatically determine the target dimensionality, the proposed method extracts the orthogonal subspace bases of data incrementally to realize dimensionality reduction and avoids complex computations. Besides, EOCA can merge two learned subspaces that are represented by their orthonormal bases to a new one to eliminate the outlier effects, and the new subspace is proved to be unique. Extensive experiments and analysis demonstrate that EOCA is fast and achieves competitive results, especially for noisy data.
更多查看译文
关键词
Dimensionality reduction,incremental learning,orthogonal component (OC),subspace learning
AI 理解论文
溯源树
样例
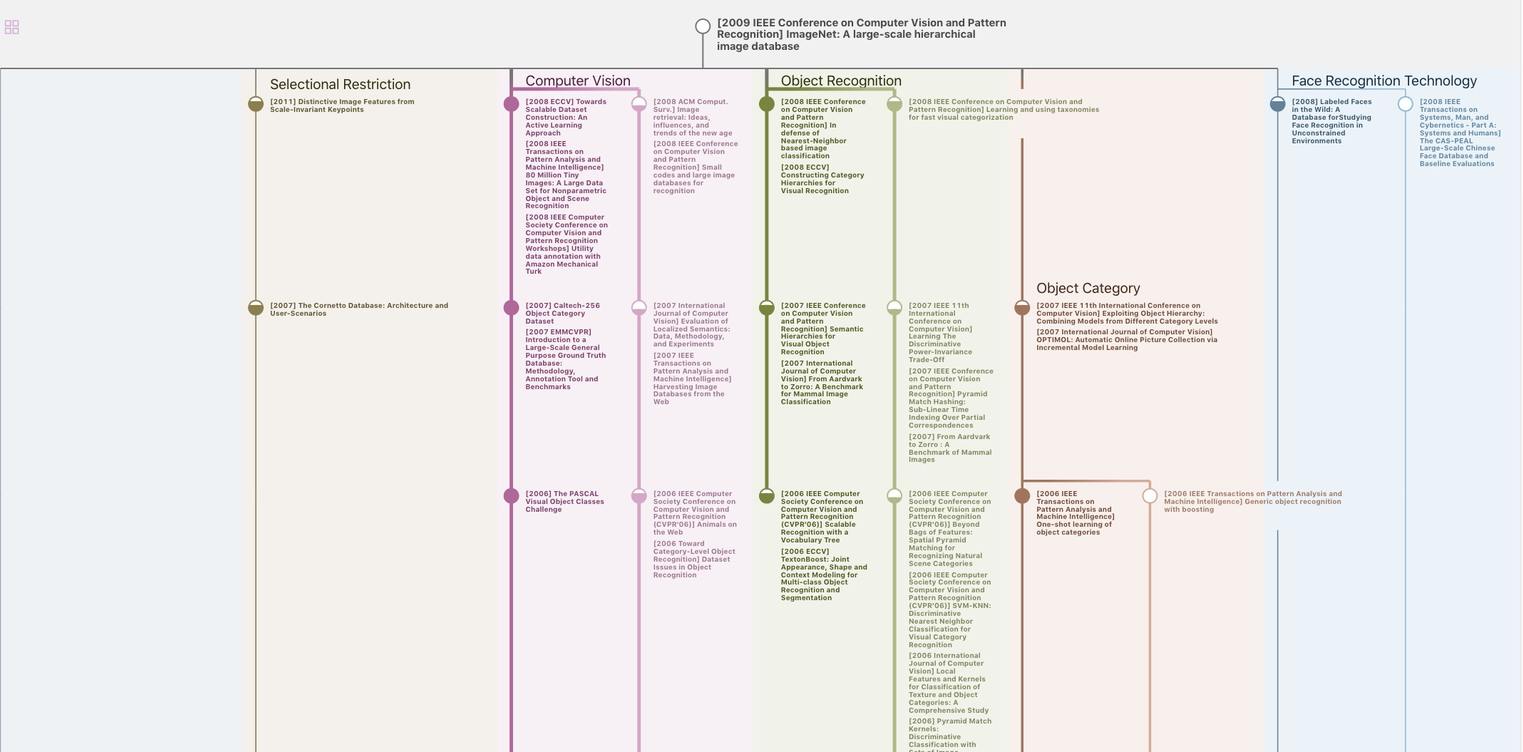
生成溯源树,研究论文发展脉络
Chat Paper
正在生成论文摘要