How do Offline Measures for Exploration in Reinforcement Learning behave?
arxiv(2020)
摘要
Sufficient exploration is paramount for the success of a reinforcement learning agent. Yet, exploration is rarely assessed in an algorithm-independent way. We compare the behavior of three data-based, offline exploration metrics described in the literature on intuitive simple distributions and highlight problems to be aware of when using them. We propose a fourth metric,uniform relative entropy, and implement it using either a k-nearest-neighbor or a nearest-neighbor-ratio estimator, highlighting that the implementation choices have a profound impact on these measures.
更多查看译文
关键词
offline measures,exploration,reinforcement learning
AI 理解论文
溯源树
样例
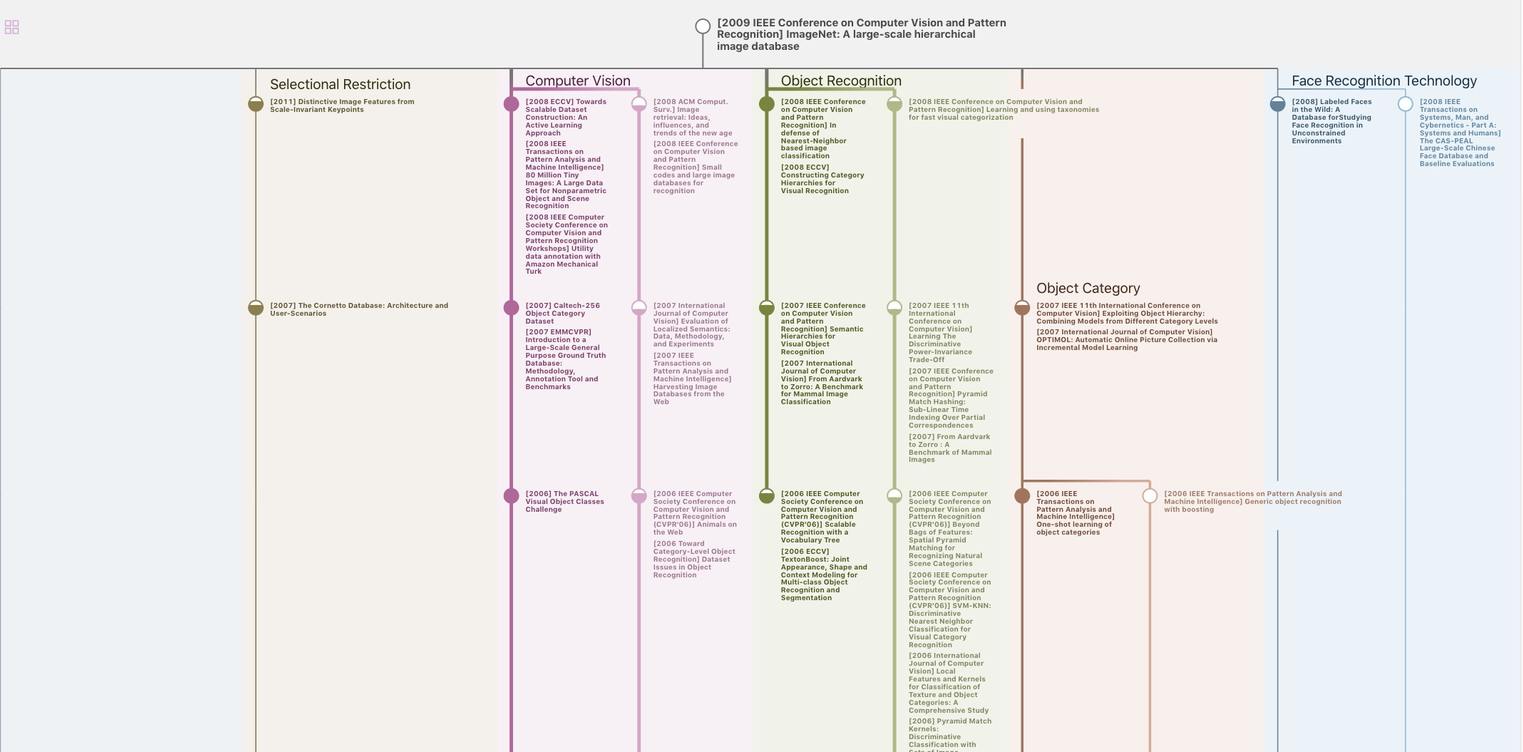
生成溯源树,研究论文发展脉络
Chat Paper
正在生成论文摘要