Motion Rectification Network for Unsupervised Learning of Monocular Depth and Camera Motion
2020 IEEE INTERNATIONAL CONFERENCE ON IMAGE PROCESSING (ICIP)(2020)
摘要
Although unsupervised methods of monocular depth and camera motion estimation have made significant progress, most of them are based on the static scene assumption and may perform poorly in dynamic scenes. In this paper, we propose a novel framework for unsupervised learning of monocular depth and camera motion estimation, which is applicable to dynamic scenes. Firstly, the framework is trained to obtain initial inference results by assuming the scene is static, through minimizing a photometric consistency loss and a 3D transformation consistency loss. Then, the framework is fine-tuned by jointly learning with a motion rectification network (RecNet). Specifically, RecNet is designed to rectify the individual motion of moving objects and generate motion rectified images, enabling the framework to learn accurately in dynamic scenes. Extensive experiments have been done on the KITTI dataset. Results show that our method achieves state-of-the-art performance on both depth prediction and camera motion estimation tasks.
更多查看译文
关键词
Depth prediction, camera motion estimation, motion rectification, unsupervised learning
AI 理解论文
溯源树
样例
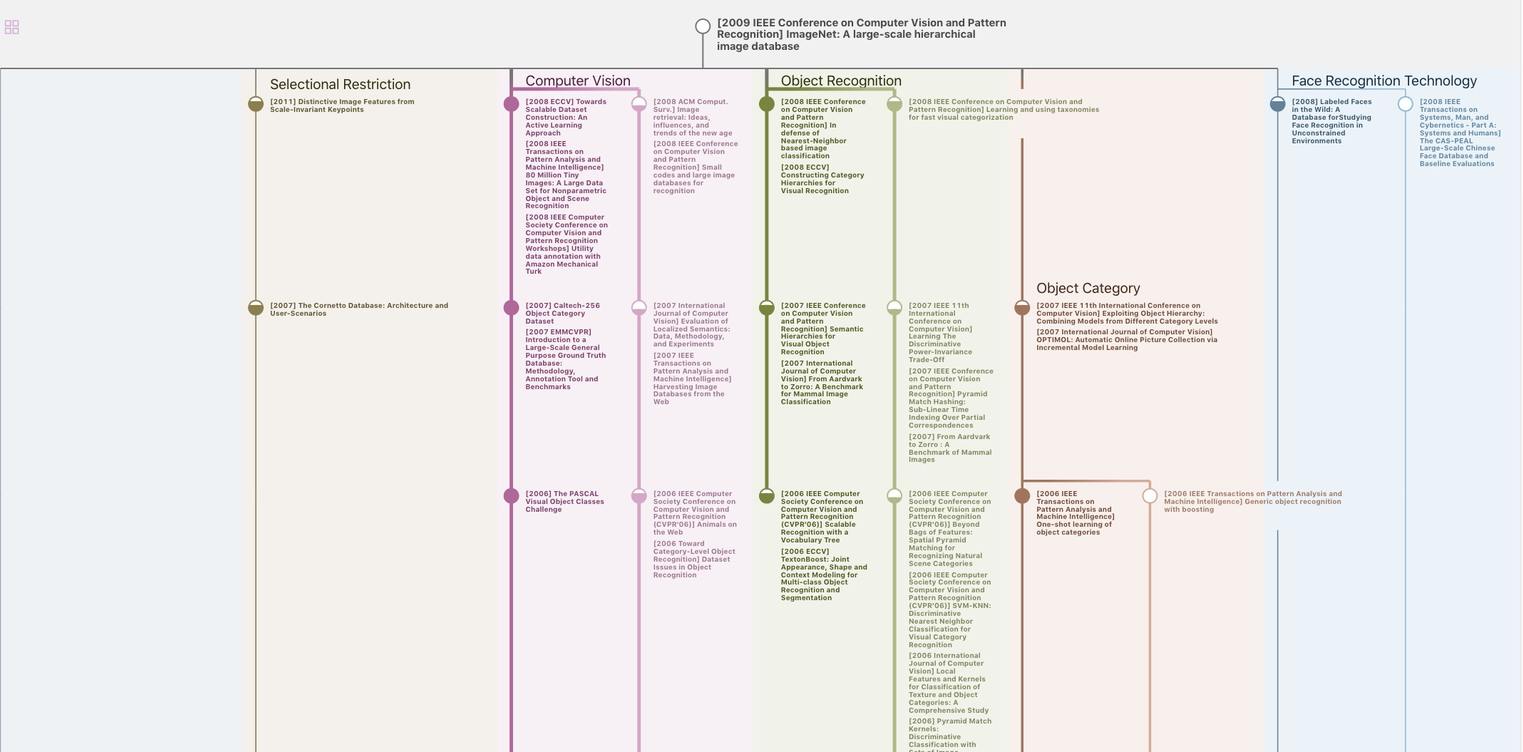
生成溯源树,研究论文发展脉络
Chat Paper
正在生成论文摘要