NON-AUTOREGRESSIVE TRANSFORMER ASR WITH CTC-ENHANCED DECODER INPUT
2021 IEEE INTERNATIONAL CONFERENCE ON ACOUSTICS, SPEECH AND SIGNAL PROCESSING (ICASSP 2021)(2021)
摘要
Non-autoregressive (NAR) transformer models have achieved significantly inference speedup but at the cost of inferior accuracy compared to autoregressive (AR) models in automatic speech recognition (ASR). Most of the NAR transformers take a fixed-length sequence filled with MASK tokens or a redundant sequence copied from encoder states as decoder input, they cannot provide efficient target-side information thus leading to accuracy degradation. To address this problem, we propose a CTC-enhanced NAR transformer, which generates target sequence by refining predictions of the CTC module. Experimental results show that our method outperforms all previous NAR counterparts and achieves 50x faster decoding speed than a strong AR baseline with only 0.0 similar to 0.3 absolute CER degradation on Aishell-1 and Aishell-2 datasets.
更多查看译文
关键词
non-autoregressive, transformer, ctc
AI 理解论文
溯源树
样例
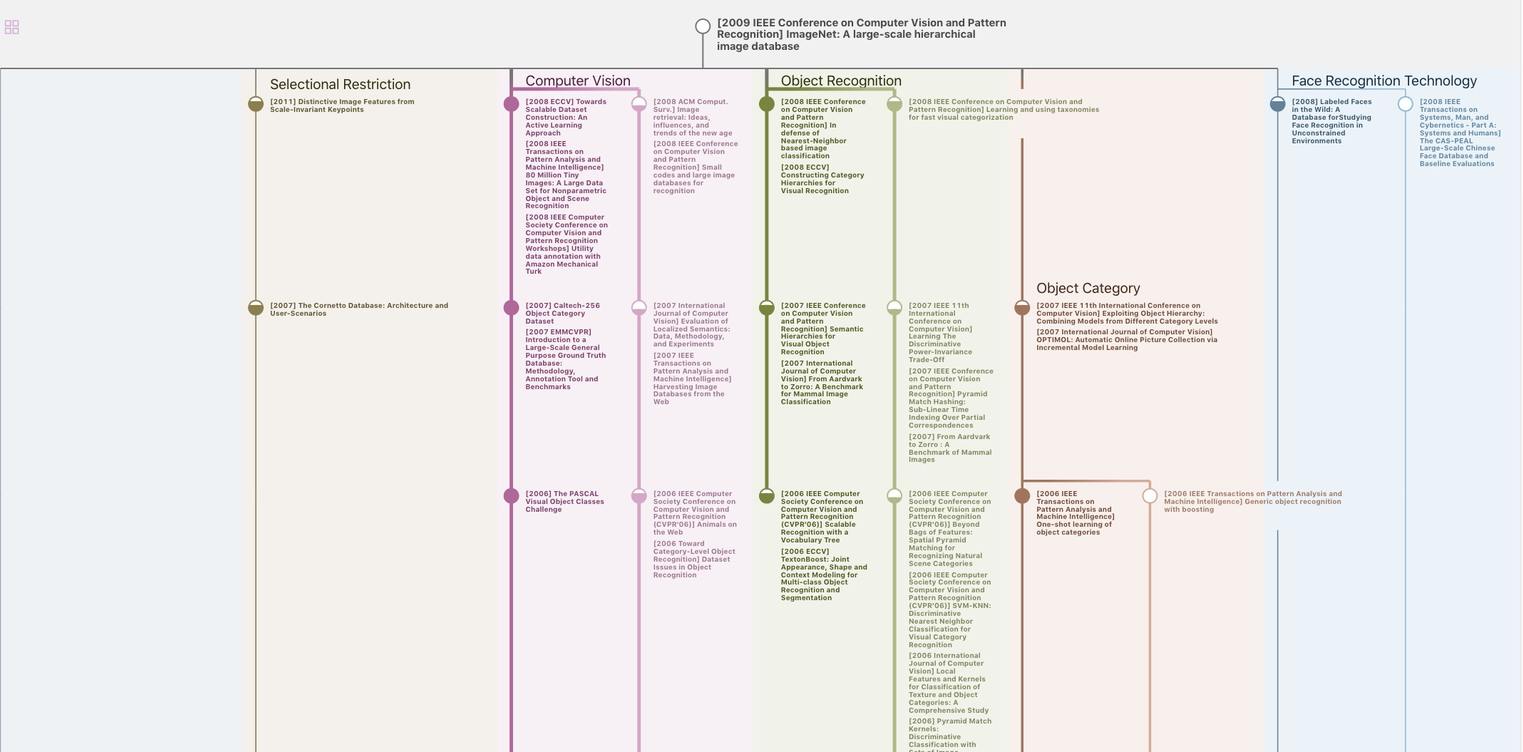
生成溯源树,研究论文发展脉络
Chat Paper
正在生成论文摘要