Learning to encode spatial relations from natural language
user-5ebe3c75d0b15254d6c50b36(2018)
摘要
Natural language processing has made significant inroads into learning the semantics of words through distributional approaches, however representations learnt via these methods fail to capture certain kinds of information implicit in the real world. In particular, spatial relations are encoded in a way that is inconsistent with human spatial reasoning and lacking invariance to viewpoint changes. We present a system capable of capturing the semantics of spatial relations such as behind, left of, etc from natural language. Our key contributions are a novel multi-modal objective based on generating images of scenes from their textual descriptions, and a new dataset on which to train it. We demonstrate that internal representations are robust to meaning preserving transformations of descriptions (paraphrase invariance), while viewpoint invariance is an emergent property of the system.
更多查看译文
AI 理解论文
溯源树
样例
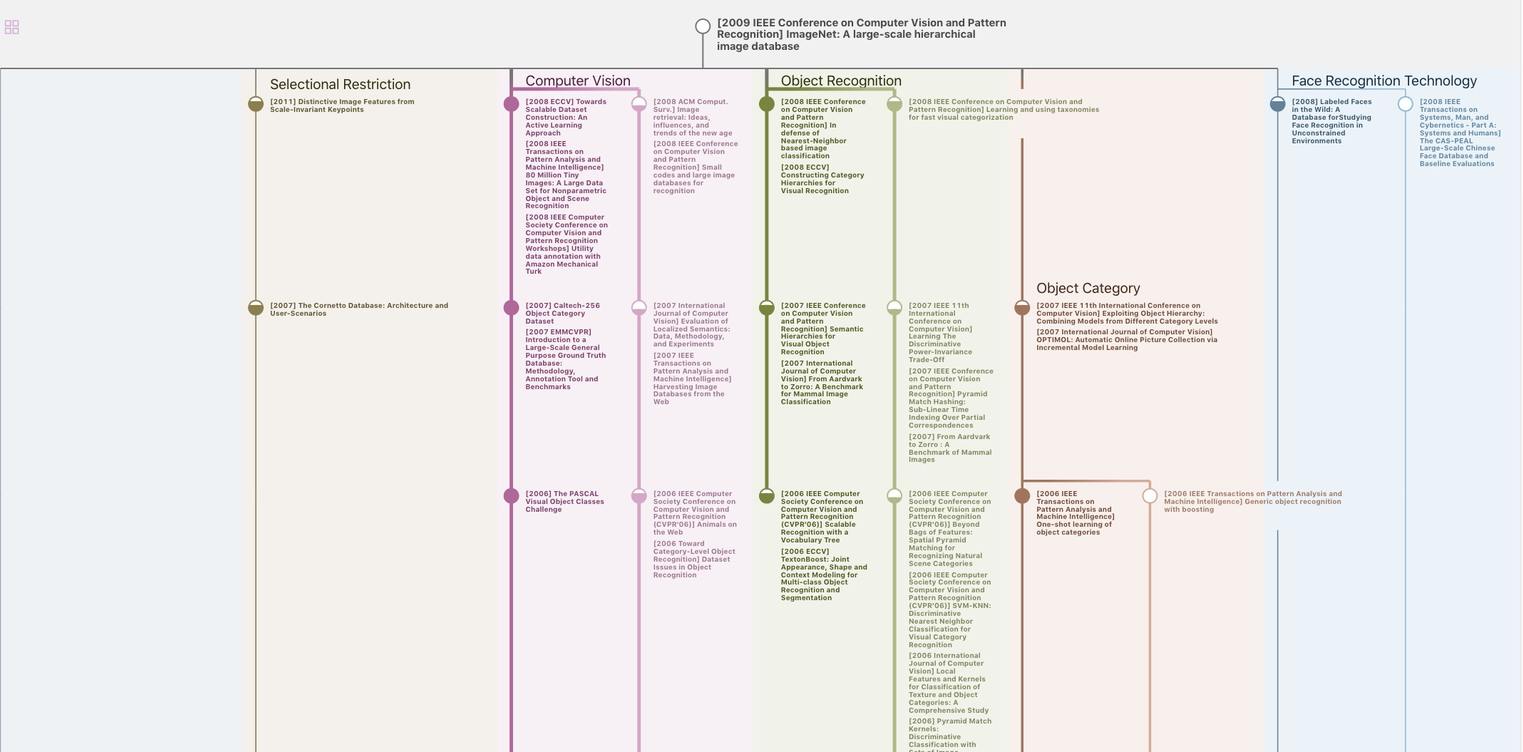
生成溯源树,研究论文发展脉络
Chat Paper
正在生成论文摘要