Making deep neural networks right for the right scientific reasons by interacting with their explanations
NATURE MACHINE INTELLIGENCE(2020)
摘要
Deep neural networks have demonstrated excellent performances in many real-world applications. Unfortunately, they may show Clever Hans-like behaviour (making use of confounding factors within datasets) to achieve high performance. In this work we introduce the novel learning setting of explanatory interactive learning and illustrate its benefits on a plant phenotyping research task. Explanatory interactive learning adds the scientist into the training loop, who interactively revises the original model by providing feedback on its explanations. Our experimental results demonstrate that explanatory interactive learning can help to avoid Clever Hans moments in machine learning and encourages (or discourages, if appropriate) trust in the underlying model.
更多查看译文
关键词
deep neural networks,neural networks,right scientific reasons
AI 理解论文
溯源树
样例
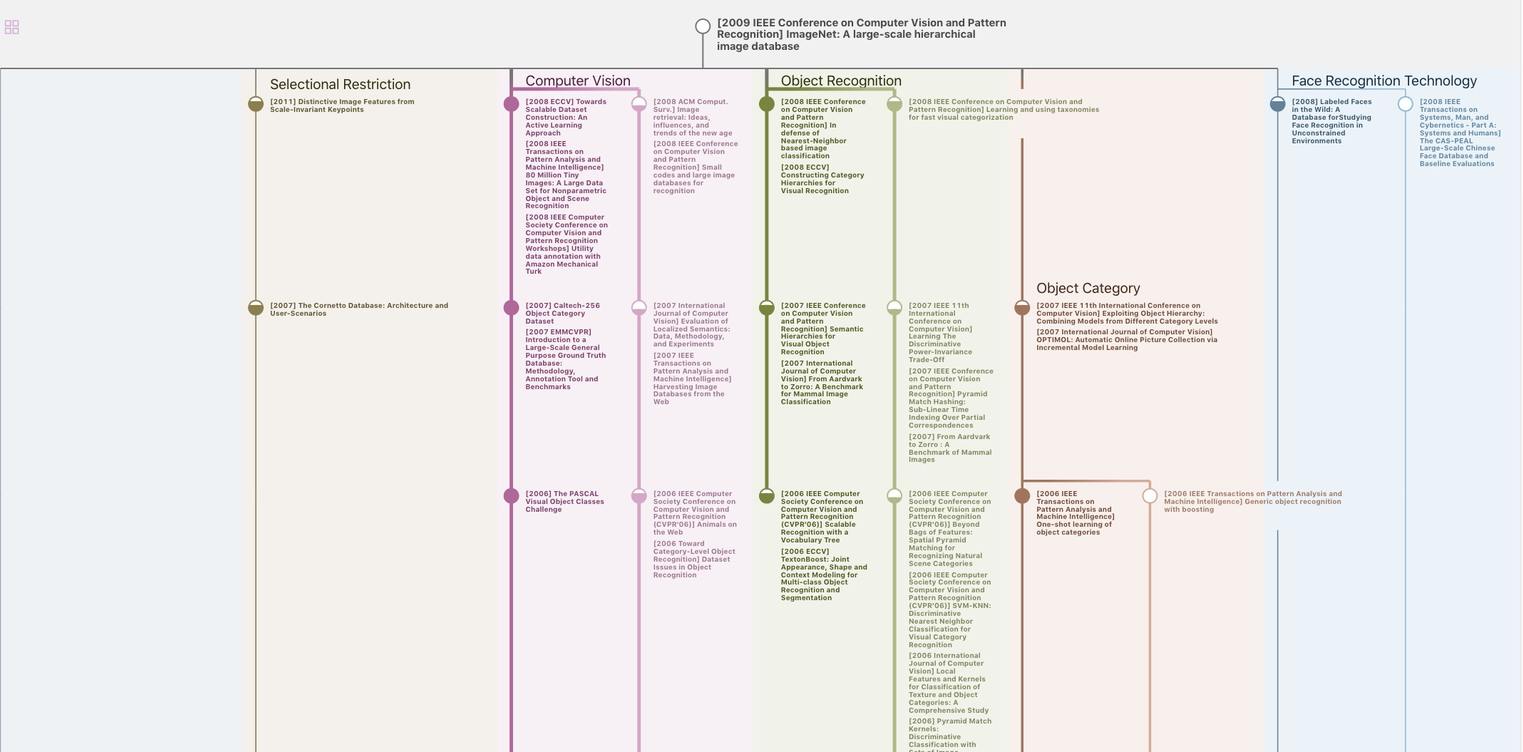
生成溯源树,研究论文发展脉络
Chat Paper
正在生成论文摘要