Sequential Knockoffs For Continuous And Categorical Predictors: With Application To A Large Psoriatic Arthritis Clinical Trial Pool
STATISTICS IN MEDICINE(2021)
摘要
Knockoffs provide a general framework for controlling the false discovery rate when performing variable selection. Much of the Knockoffs literature focuses on theoretical challenges and we recognize a need for bringing some of the current ideas into practice. In this paper we propose a sequential algorithm for generating knockoffs when underlying data consists of both continuous and categorical (factor) variables. Further, we present a heuristic multiple knockoffs approach that offers a practical assessment of how robust the knockoff selection process is for a given dataset. We conduct extensive simulations to validate performance of the proposed methodology. Finally, we demonstrate the utility of the methods on a large clinical data pool of more than 2000 patients with psoriatic arthritis evaluated in four clinical trials with an IL-17A inhibitor, secukinumab (Cosentyx), where we determine prognostic factors of a well established clinical outcome. The analyses presented in this paper could provide a wide range of applications to commonly encountered datasets in medical practice and other fields where variable selection is of particular interest.
更多查看译文
关键词
false discovery rate, knockoff filter, psoriatic arthritis, sequential knockoffs, variable selection
AI 理解论文
溯源树
样例
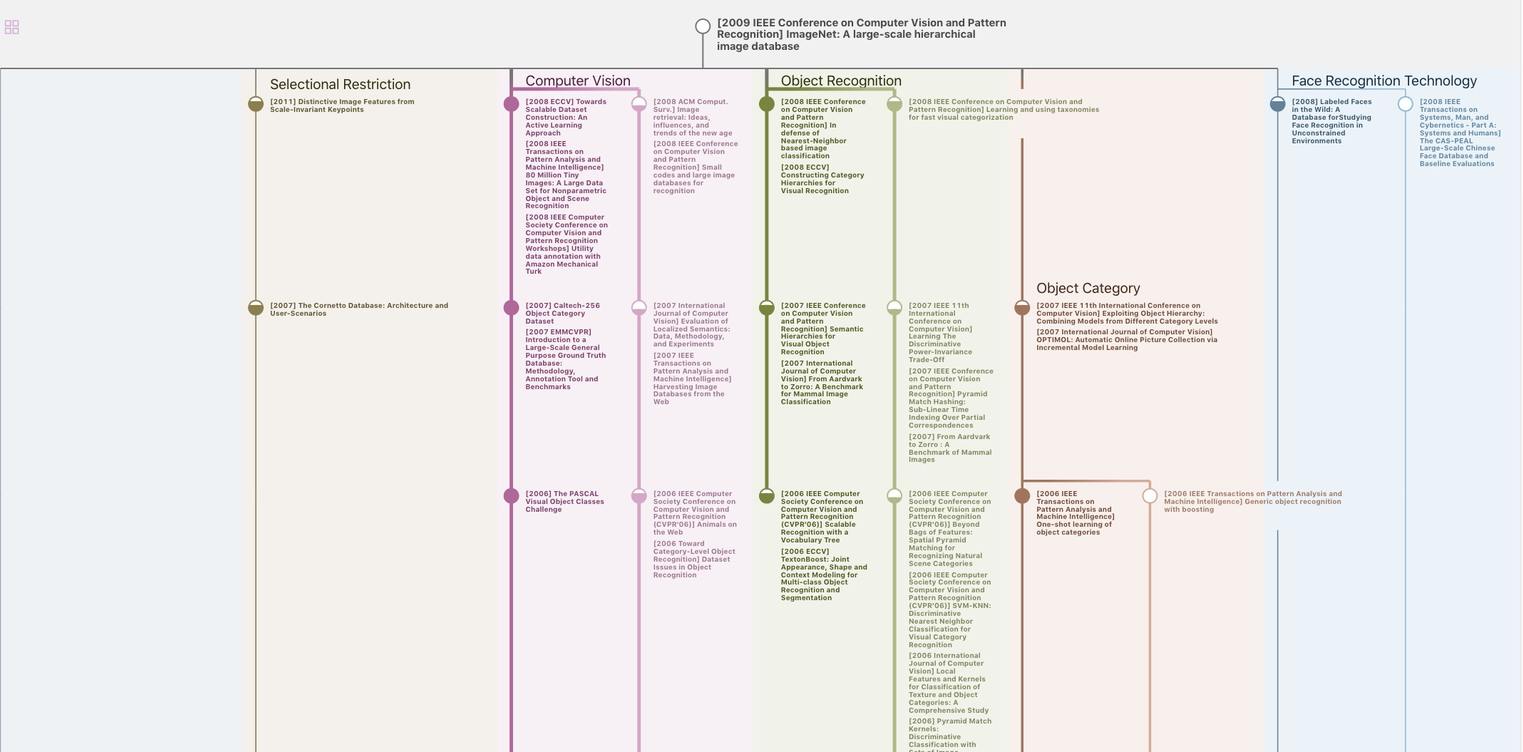
生成溯源树,研究论文发展脉络
Chat Paper
正在生成论文摘要