Approximate Gaussian Process Regression and Performance Analysis Using Composite Likelihood
2020 IEEE 30th International Workshop on Machine Learning for Signal Processing (MLSP)(2020)
摘要
Nonparametric regression using Gaussian Process (GP) models is a powerful but computationally demanding method. While various approximation methods have been developed to mitigate its computation complexity, few works have addressed the quality of the resulting approximations of the target posterior. In this paper we start from a general belief updating framework that can generate various approximations. We show that applying using composite likelihoods yields computationally scalable approximations for both GP learning and prediction. We then analyze the quality of the approximation in terms of averaged prediction errors as well as Kullback-Leibler (KL) divergences.
更多查看译文
关键词
target posterior,performance analysis,composite likelihood,nonparametric regression,GP learning,computation complexity,Gaussian process regression approximation methods,belief updating framework,Kullback-Leibler divergence,computationally scalable approximations
AI 理解论文
溯源树
样例
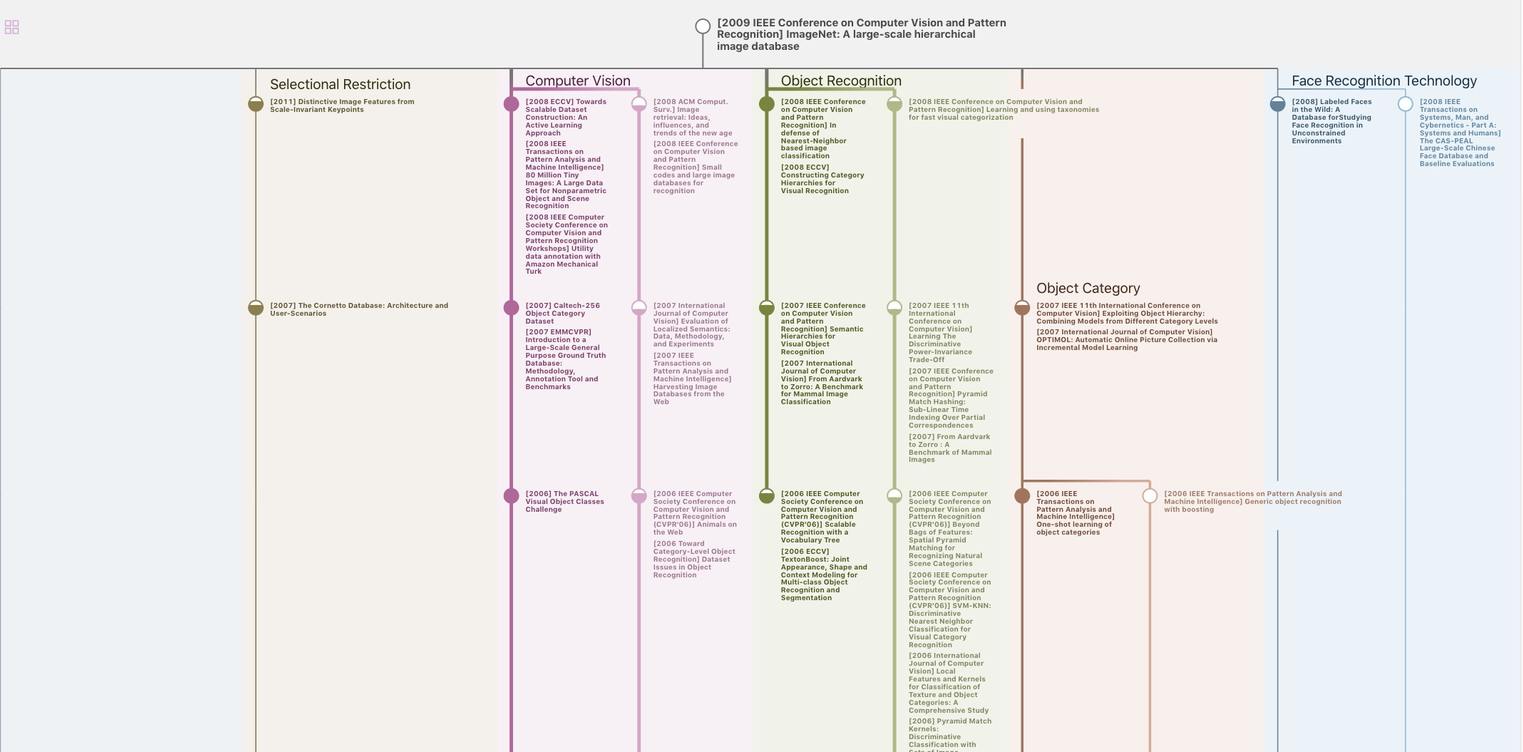
生成溯源树,研究论文发展脉络
Chat Paper
正在生成论文摘要