Learning General Transformations of Data for Out-of-Sample Extensions
2020 IEEE 30th International Workshop on Machine Learning for Signal Processing (MLSP)(2020)
摘要
While generative models such as GANs have been successful at mapping from noise to specific distributions of data, or more generally from one distribution of data to another, they cannot isolate the transformation that is occurring and apply it to a new distribution not seen in training. Thus, they memorize the domain of the transformation, and cannot generalize the transformation out of sample. To address this, we propose a new neural network called a Neuron Transformation Network (NT-Net) that isolates the signal representing the transformation itself from the other signals representing internal distribution variation. This signal can then be removed from a new dataset distributed differently from the original one trained on. We demonstrate the effectiveness of our NTNet on more than a dozen synthetic and biomedical single-cell RNA sequencing datasets, where the NTNet is able to learn the data transformation performed by genetic and drug perturbations on one sample of cells and successfully apply it to another sample of cells to predict treatment outcome.
更多查看译文
关键词
neural network,NT-Net,internal distribution variation,data transformation,out-of-sample extensions,generative models,data general transformation learning,GANs,biomedical single-cell RNA sequencing datasets,neuron transformation network,drug perturbations,genetic perturbations,treatment outcome
AI 理解论文
溯源树
样例
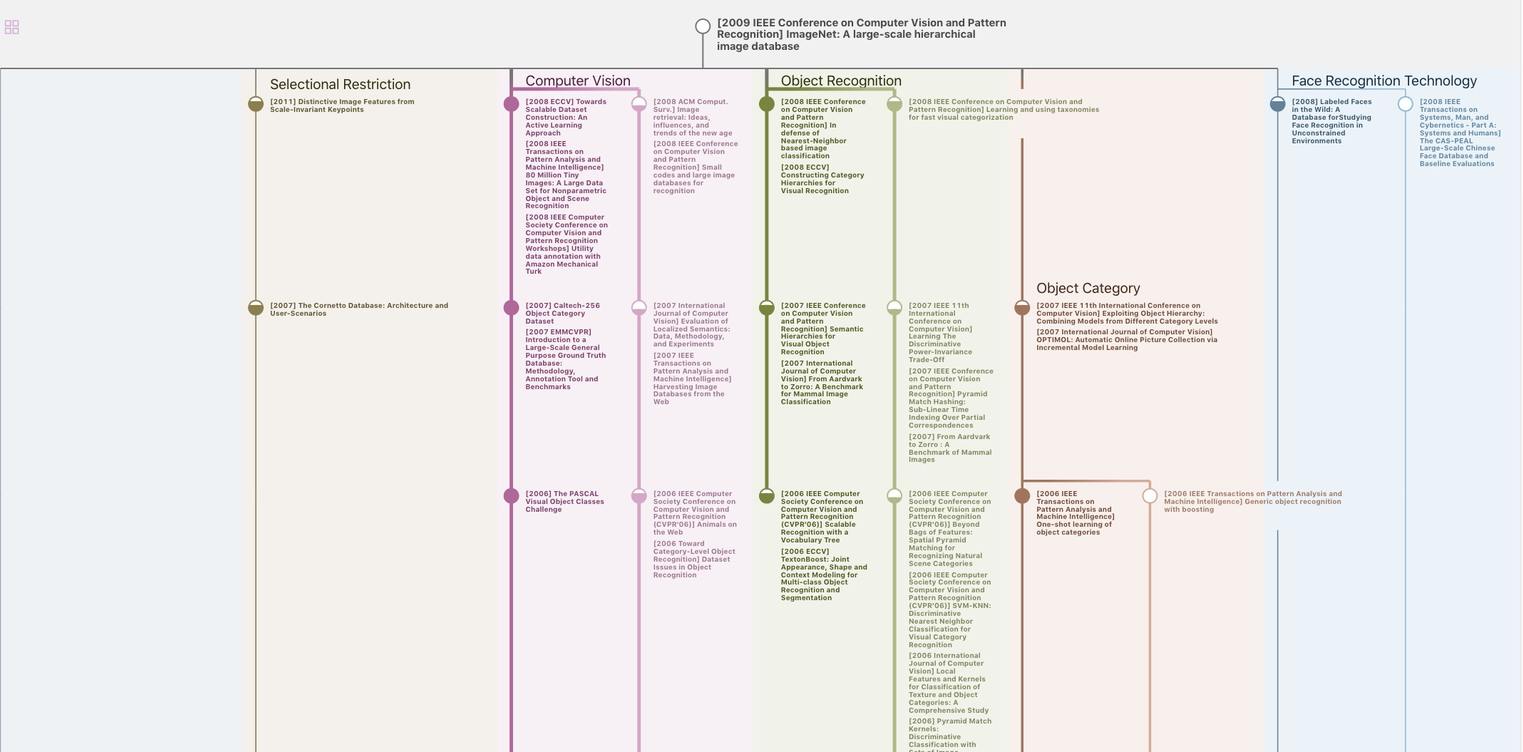
生成溯源树,研究论文发展脉络
Chat Paper
正在生成论文摘要