One-vs.-One Mitigation of Intersectional Bias: A General Method for Extending Fairness-Aware Binary Classification
NEW TRENDS IN DISRUPTIVE TECHNOLOGIES, TECH ETHICS AND ARTIFICIAL INTELLIGENCE: THE DITTET COLLECTION(2022)
摘要
With the widespread adoption of machine learning, the impact of discriminatory biases on outputs has received attention, and various methods for mitigating discriminatory biases have been proposed. Among the discriminatory bias, it is difficult to remove intersectional bias, which brings unfair situations when there are subgroups treated worse in the same protected group. Despite the development of some conventional methods for mitigating intersectional bias, applicable use-case scenarios are limited. To broaden the use-case scenarios, where intersectional bias can be mitigated, in this study, we propose a method called One-vs.-One Mitigation. This method applies a process of comparison between each pair of subgroups based on sensitive attributes to the fairness-aware machine learning for binary classification. We compare our method with conventional fairness-aware binary classification methods in comprehensive scenarios using three approaches (pre-, in-, and post-processing), three metrics (demographic parity, equalized odds, and equal opportunity), and a real-world dataset. Experimental results show that our method mitigates intersectional bias much better than conventional methods in all scenarios. Based on these findings, we have opened up a potential path of fairness-aware binary classification for solving more realistic problems with multiple sensitive attributes.
更多查看译文
关键词
Fairness, Machine learning, Intersectional bias
AI 理解论文
溯源树
样例
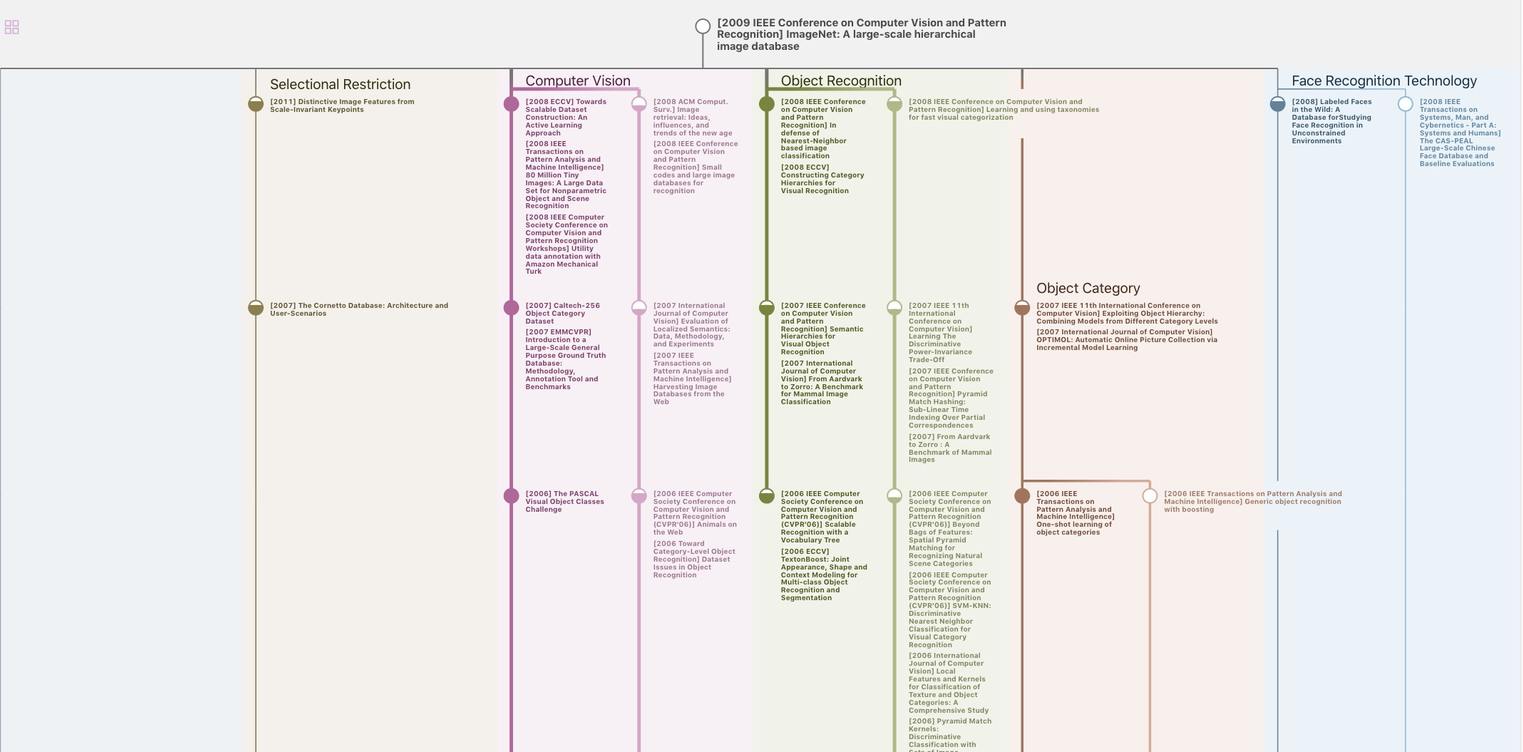
生成溯源树,研究论文发展脉络
Chat Paper
正在生成论文摘要