A Centroid Loss for Weakly Supervised Semantic Segmentation in Quality Control and Inspection Application
arxiv(2020)
摘要
Process automation has enabled a level of accuracy and productivity that goes beyond human ability, and one critical area where automation is making a huge difference is the machine vision system. In this paper, a semantic segmentation solution is proposed for two scenes. One is the inspection intended for vessel corrosion detection, and the other is a detection system used to assist quality control on the surgery toolboxes prepared by the sterilization unit of a hospital. In order to reduce the time required to prepare pixel-level ground truth, this work focuses on the use of weakly supervised annotations (scribbles). Moreover, our solution integrates a clustering approach into a semantic segmentation network, thus reducing the negative effects caused by weakly supervised annotations. To evaluate the performance of our approach, two datasets are collected from the real world (vessels' structure and hospital surgery toolboxes) for both training and validation. According to the result of analysis, the approach proposed in this paper produce a satisfactory performance on two datasets through the use of weak annotations.
更多查看译文
关键词
semantic segmentation,quality-control quality-control,weakly-supervised
AI 理解论文
溯源树
样例
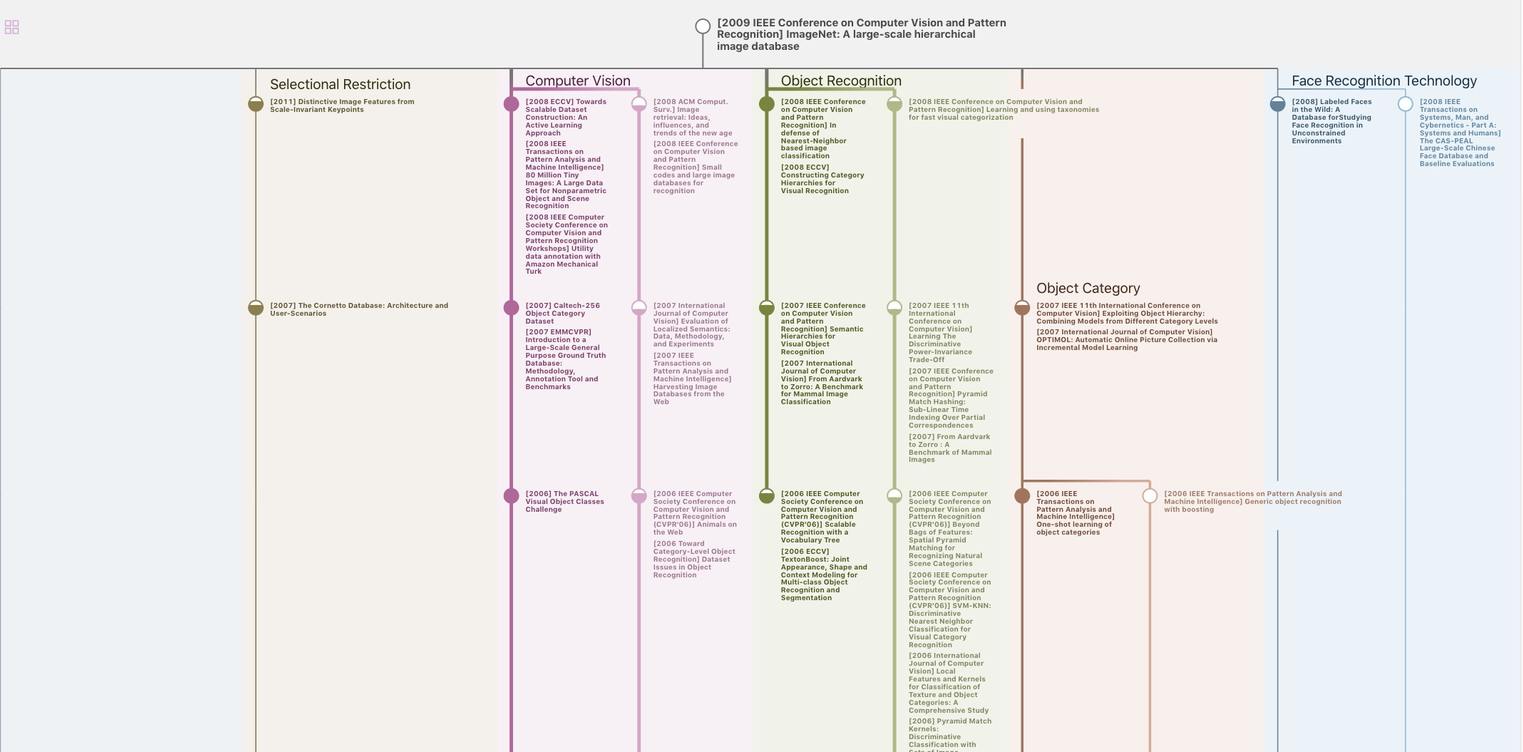
生成溯源树,研究论文发展脉络
Chat Paper
正在生成论文摘要