Node-Adaptive Regularization for Graph Signal Reconstruction
IEEE Open Journal of Signal Processing(2021)
摘要
A critical task in graph signal processing is to estimate the true signal from noisy observations over a subset of nodes, also known as the reconstruction problem. In this paper, we propose a node-adaptive regularization for graph signal reconstruction, which surmounts the conventional Tikhonov regularization, giving rise to more degrees of freedom; hence, an improved performance. We formulate the node-adaptive graph signal denoising problem, study its bias-variance trade-off, and identify conditions under which a lower mean squared error and variance can be obtained with respect to Tikhonov regularization. Compared with existing approaches, the node-adaptive regularization enjoys more general priors on the local signal variation, which can be obtained by optimally designing the regularization weights based on Prony's method or semidefinite programming. As these approaches require additional prior knowledge, we also propose a minimax (worst-case) strategy to address instances where this extra information is unavailable. Numerical experiments with synthetic and real data corroborate the proposed regularization strategy for graph signal denoising and interpolation, and show its improved performance compared with competing alternatives.
更多查看译文
关键词
Bias-variance trade-off,graph regularization,graph signal denoising,graph signal processing
AI 理解论文
溯源树
样例
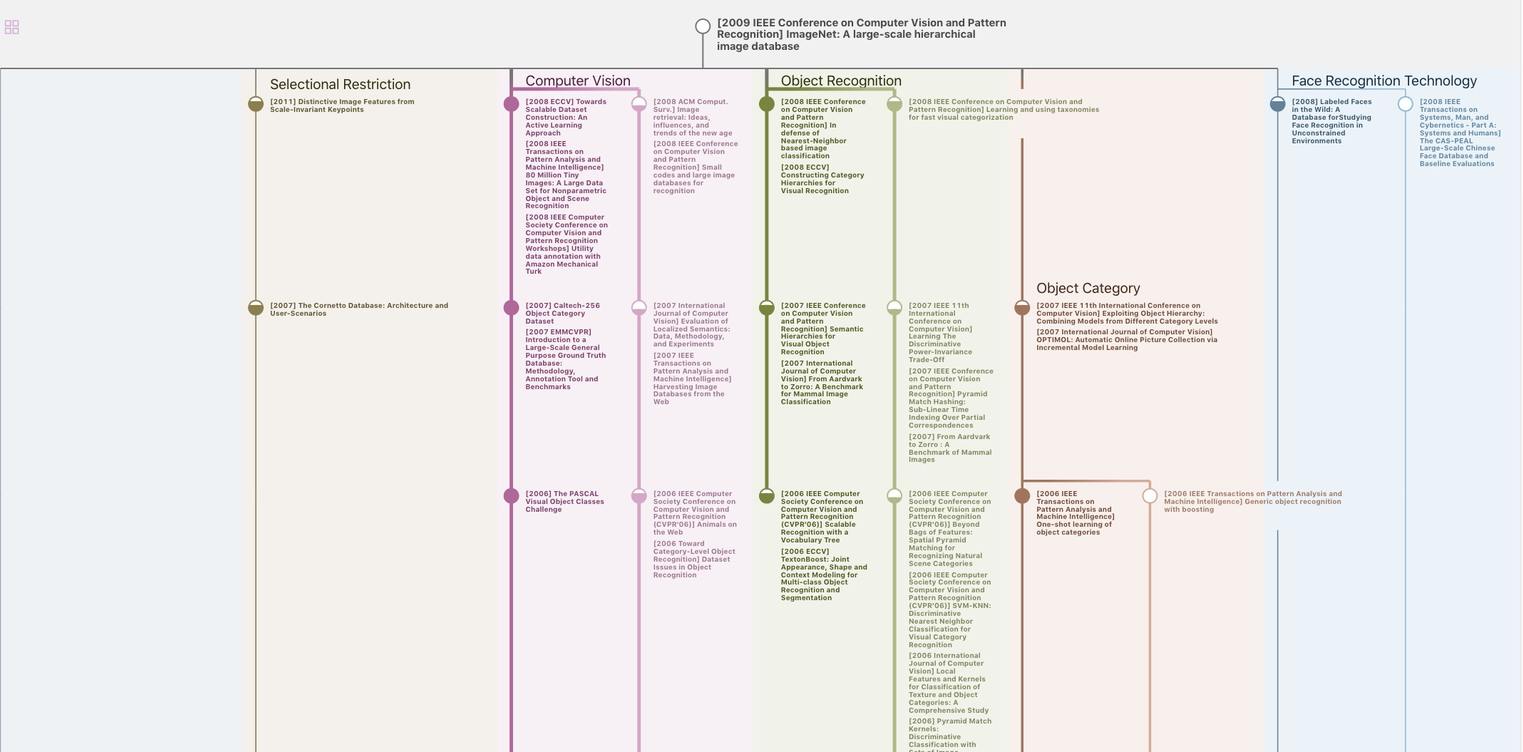
生成溯源树,研究论文发展脉络
Chat Paper
正在生成论文摘要