Automated generation of test scenario models for the system-level safety assurance of autonomous vehicles
MODELS '20: ACM/IEEE 23rd International Conference on Model Driven Engineering Languages and Systems Virtual Event Canada October, 2020(2020)
摘要
Autonomous vehicles controlled by advanced machine learning techniques are significantly gaining in popularity. However, the safety engineering practices currently used in such vehicles are not capable of justifying that AI techniques would prevent unsafe situations with a designated level of confidence and reliability. One related challenge is the perpetually changing environment that autonomous vehicles must interact with, which must be taken into consideration when deriving test suites for their safety assurance. As a result, a common approach for testing autonomous vehicles involves subjecting them to test scenarios and evaluating their system-level quality of service. As it stands, such system-level testing approaches do exist but only at a prototypical and conceptual level: these approaches cannot handle complex system-level traffic scenarios and related coverage criteria. I plan to address this challenge through my PhD studies by (1) defining situation coverage as an abstract coverage criteria for autonomous vehicle testing, (2) evaluating situation coverage of existing test suites obtained by off-the-shelf simulation tools, and (3) proposing a test suite generation approach that provides test scenarios with increasing situation coverage as output.
更多查看译文
AI 理解论文
溯源树
样例
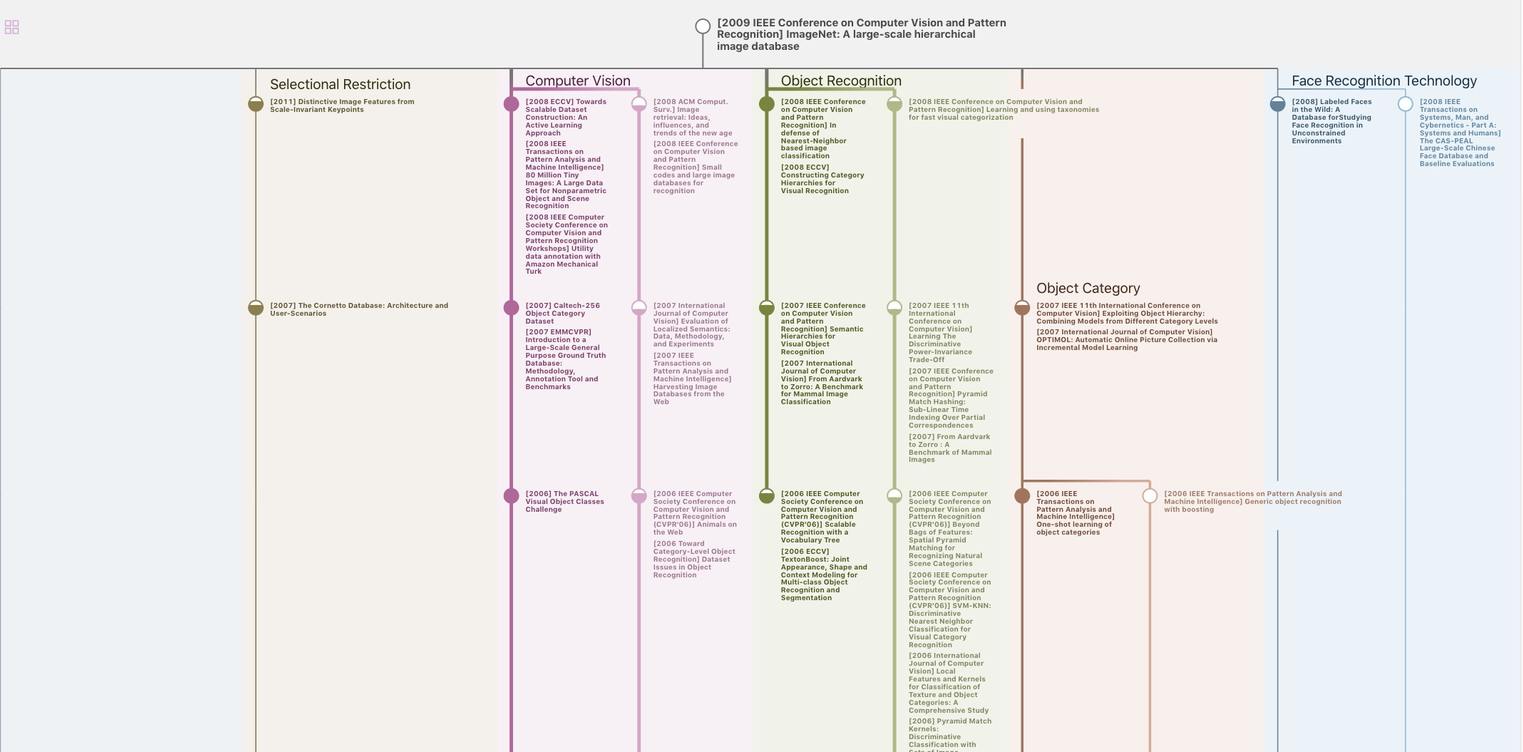
生成溯源树,研究论文发展脉络
Chat Paper
正在生成论文摘要