SpeakerNet: 1D Depth-wise Separable Convolutional Network for Text-Independent Speaker Recognition and Verification
arxiv(2020)
摘要
We propose SpeakerNet - a new neural architecture for speaker recognition and speaker verification tasks. It is composed of residual blocks with 1D depth-wise separable convolutions, batch-normalization, and ReLU layers. This architecture uses x-vector based statistics pooling layer to map variable-length utterances to a fixed-length embedding (q-vector). SpeakerNet-M is a simple lightweight model with just 5M parameters. It doesn't use voice activity detection (VAD) and achieves close to state-of-the-art performance scoring an Equal Error Rate (EER) of 2.10% on the VoxCeleb1 cleaned and 2.29% on the VoxCeleb1 trial files.
更多查看译文
关键词
speakernet,recognition,depth-wise,text-independent
AI 理解论文
溯源树
样例
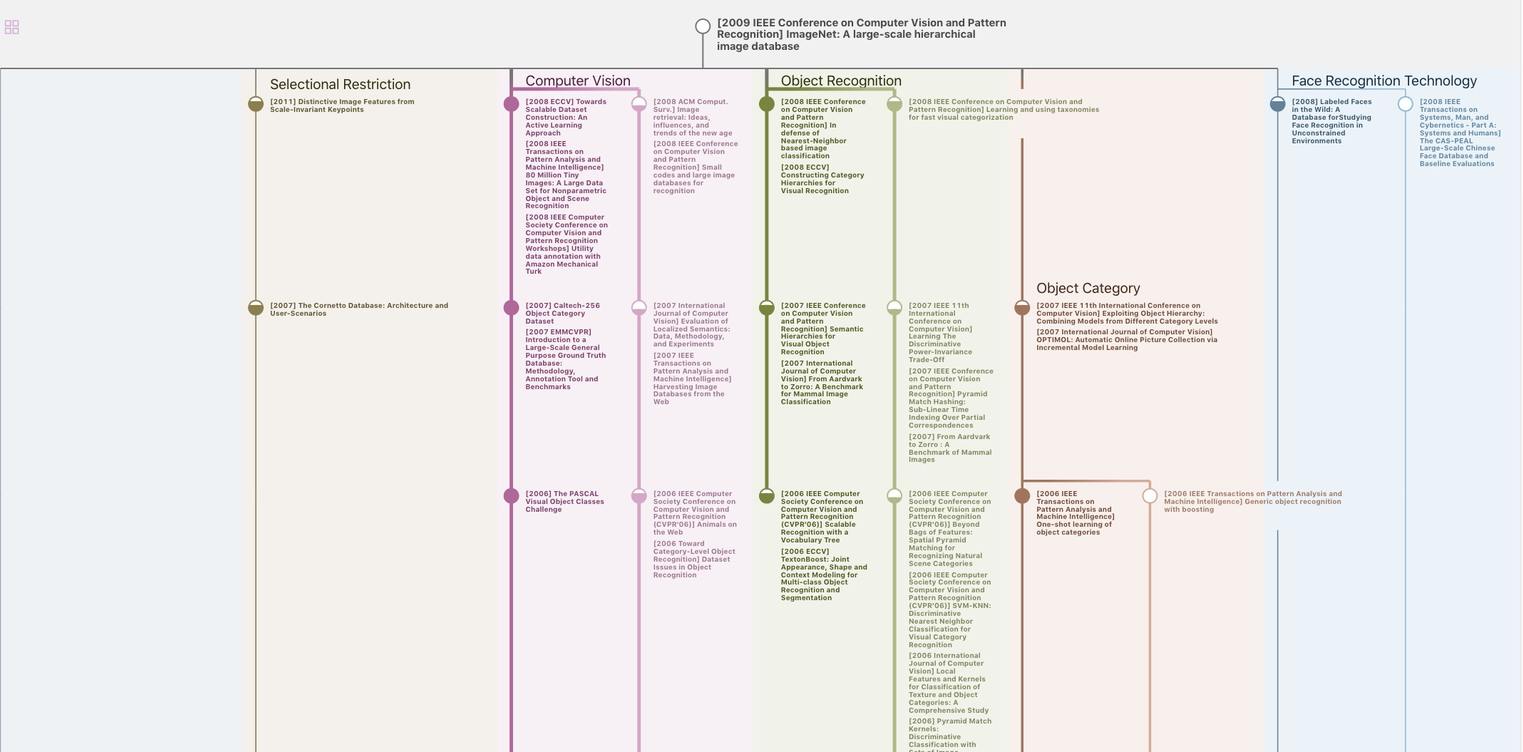
生成溯源树,研究论文发展脉络
Chat Paper
正在生成论文摘要