Unsupervised variable selection for kernel methods in systems biology
Journée Régionale de Bioinformatique et Biostatistique, Génopole Toulouse(2019)
摘要
Kernel methods have proven to be useful and successful to analyse large-scale multi-omics datasets [Schölkopf et al., 2004]. However, as stated in [Hofmann et al., 2015, Mariette et al., 2017], these methods usually suffer from a lack of interpretability as the information of thousands descriptors is summarized in a few similarity measures, that can be strongly in uenced by a large number of irrelevant descriptors. To address this issue, feature selection is a widely used strategy: it consist in selecting the most promising features during or prior the analysis. However, most existing methods are proposed in a supervised framework [Tibshirani, 1996, Robnik-Sikonja and Kononenko, 2003, Lin and Tang, 2006]. In the unsupervised framework, the number of proposals is much less important, because there is no objective criterion or value on which to tune the quality of a given feature. Proposals thus aim at preserving at best the similarities between individuals like the SPEC approach [Zhao and Liu, 2007] or at recovering a latent cluster structure, like MCFS [Cai et al., 2010], NDFS [Li et al., 2012] and UDFS [Yang et al., 2011]. In this communication, we will present a feature selection algorithm that explicitly takes advantage of the kernel structure in an unsupervised fashion.
更多查看译文
AI 理解论文
溯源树
样例
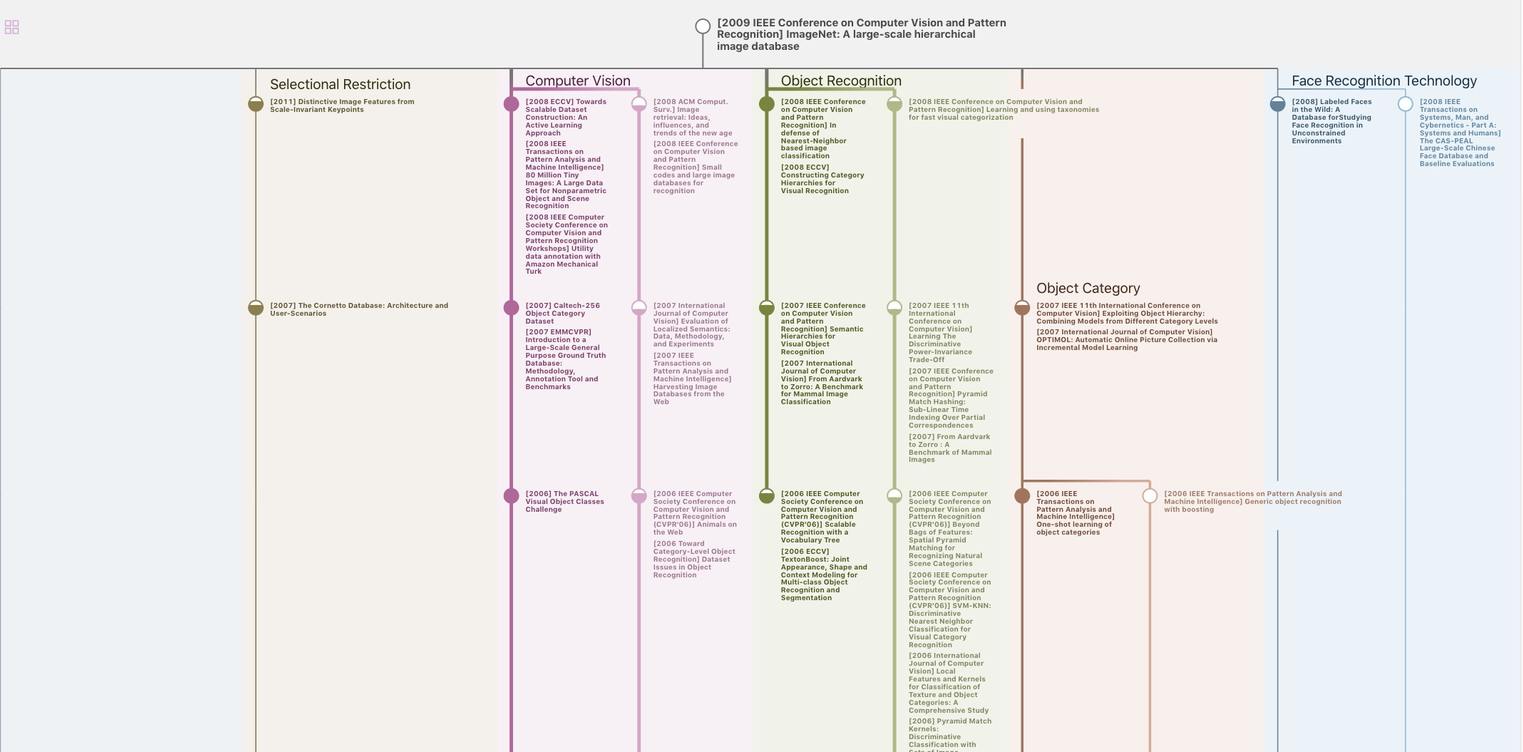
生成溯源树,研究论文发展脉络
Chat Paper
正在生成论文摘要