ST-BERT: CROSS-MODAL LANGUAGE MODEL PRE-TRAINING FOR END-TO-END SPOKEN LANGUAGE UNDERSTANDING
2021 IEEE INTERNATIONAL CONFERENCE ON ACOUSTICS, SPEECH AND SIGNAL PROCESSING (ICASSP 2021)(2021)
摘要
Language model pre-training has shown promising results in various downstream tasks. In this context, we introduce a cross-modal pre-trained language model, called Speech-Text BERT (ST-BERT), to tackle end-to-end spoken language understanding (E2E SLU) tasks. Taking phoneme posterior and subword-level text as an input, ST-BERT learns a contextualized cross-modal alignment via our two proposed pre-training tasks: Cross-modal Masked Language Modeling (CM-MLM) and Cross-modal Conditioned Language Modeling (CMCLM). Experimental results on three benchmarks present that our approach is effective for various SLU datasets and shows a surprisingly marginal performance degradation even when 1% of the training data are available. Also, our method shows further SLU performance gain via domain-adaptive pre-training with domain-specific speech-text pair data.
更多查看译文
关键词
Cross-modal language model, Cross-modal pre-training, Spoken language understanding
AI 理解论文
溯源树
样例
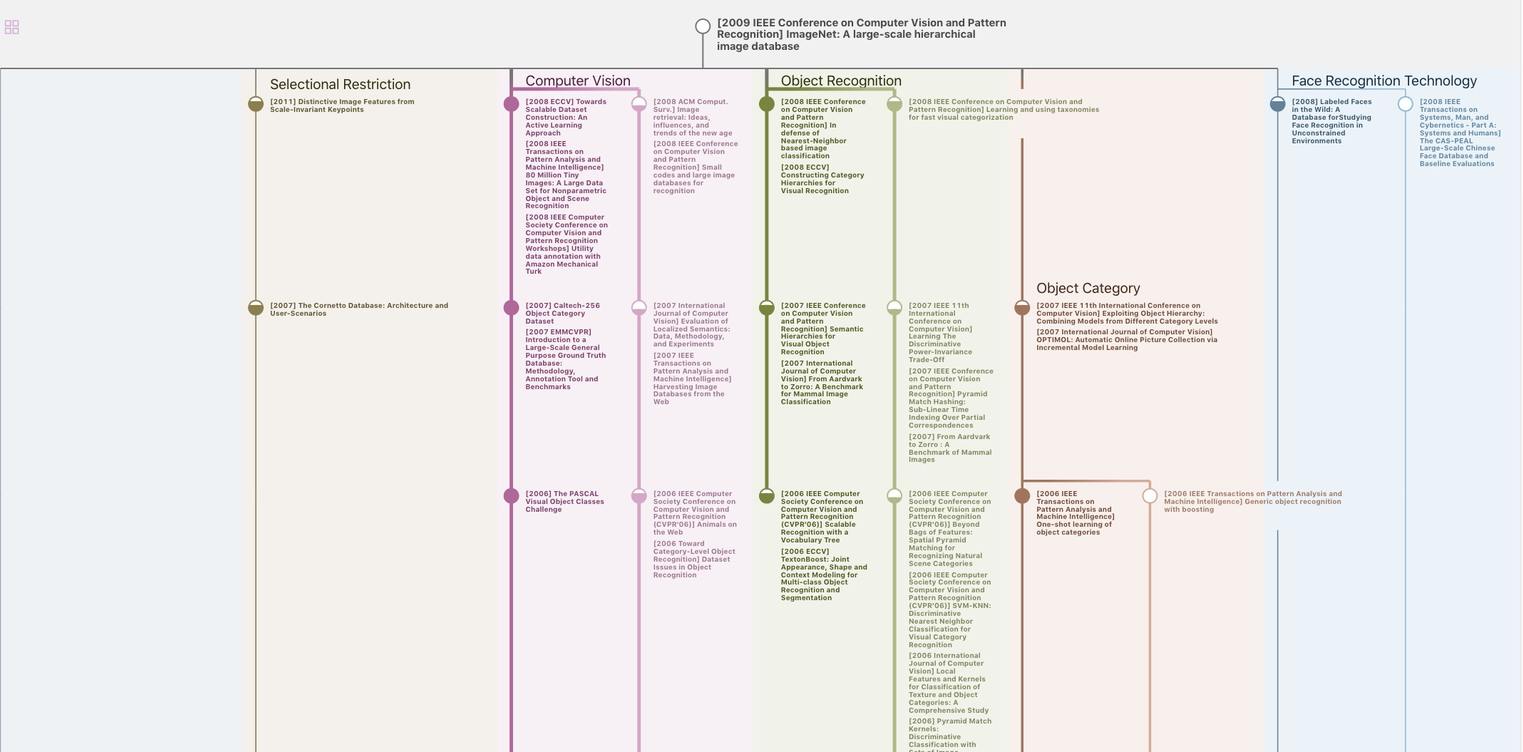
生成溯源树,研究论文发展脉络
Chat Paper
正在生成论文摘要