Simple Neighborhood Representative Pre-processing Boosts Outlier Detectors
arxiv(2022)
摘要
Over the decades, traditional outlier detectors have ignored the group-level factor when calculating outlier scores for objects in data by evaluating only the object-level factor, failing to capture the collective outliers. To mitigate this issue, we present a method called neighborhood representative (NR), which empowers all the existing outlier detectors to efficiently detect outliers, including collective outliers, while maintaining their computational integrity. It achieves this by selecting representative objects, scoring these objects, then applies the score of the representative objects to its collective objects. Without altering existing detectors, NR is compatible with existing detectors, while improving performance on real world datasets with +8% (0.72 to 0.78 AUC) relative to state-of-the-art outlier detectors.
更多查看译文
关键词
boosts outlier
AI 理解论文
溯源树
样例
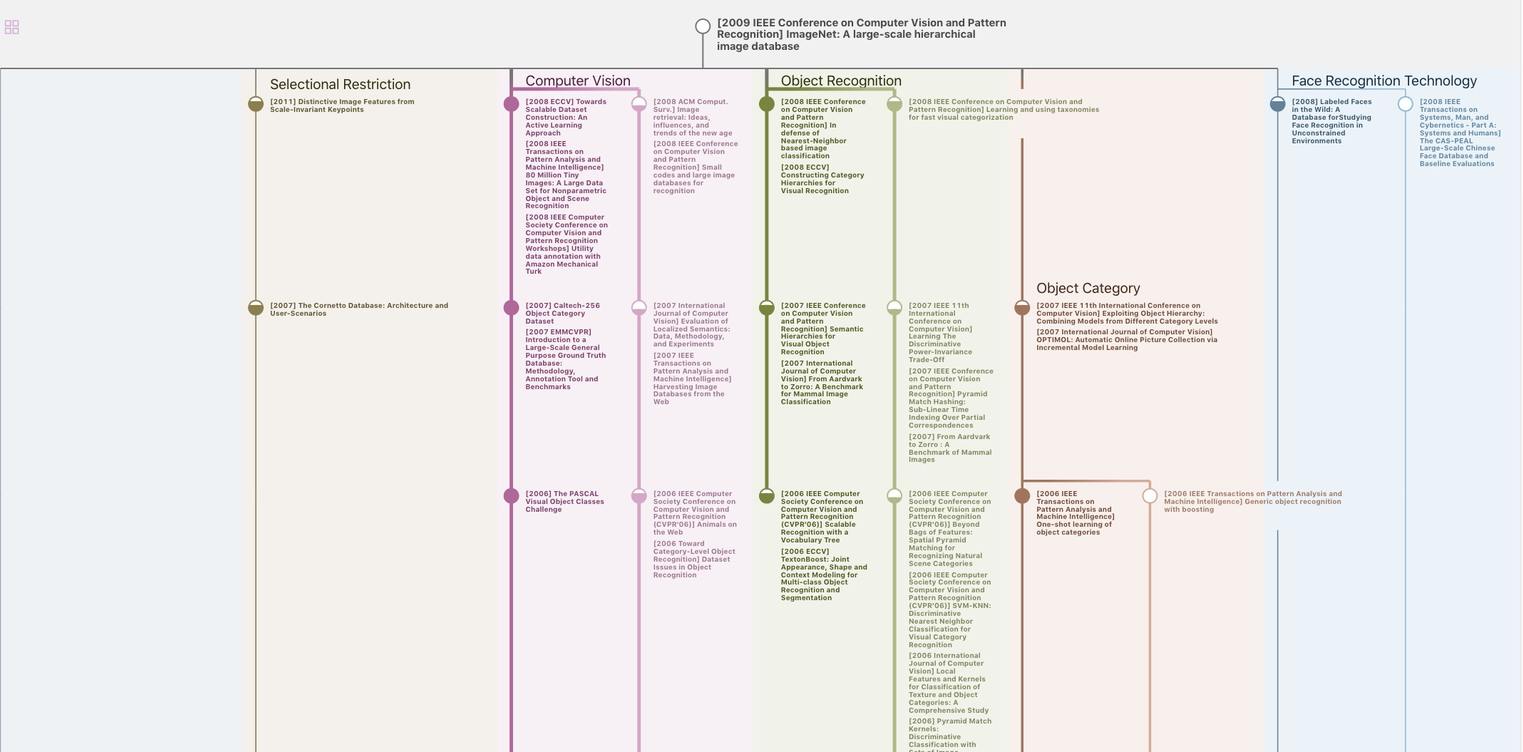
生成溯源树,研究论文发展脉络
Chat Paper
正在生成论文摘要