Predicting enhancer-promoter interactions by deep learning and matching heuristic.
BRIEFINGS IN BIOINFORMATICS(2021)
摘要
Enhancer-promoter interactions (EPIs) play an important role in transcriptional regulation. Recently, machine learning-based methods have been widely used in the genome-scale identification of EPIs due to their promising predictive performance. In this paper, we propose a novel method, termed EPI-DLMH, for predicting EPIs with the use of DNA sequences only. EPI-DLMH consists of three major steps. First, a two-layer convolutional neural network is used to learn local features, and an bidirectional gated recurrent unit network is used to capture long-range dependencies on the sequences of promoters and enhancers. Second, an attention mechanism is used for focusing on relatively important features. Finally, a matching heuristic mechanism is introduced for the exploration of the interaction between enhancers and promoters. We use benchmark datasets in evaluating and comparing the proposed method with existing methods. Comparative results show that our model is superior to currently existing models in multiple cell lines. Specifically, we found that the matching heuristic mechanism introduced into the proposed model mainly contributes to the improvement of performance in terms of overall accuracy. Additionally, compared with existing models, our model is more efficient with regard to computational speed.
更多查看译文
关键词
enhancer-promoter interactions, pretraining, deep learning, matching heuristic, DNA sequence
AI 理解论文
溯源树
样例
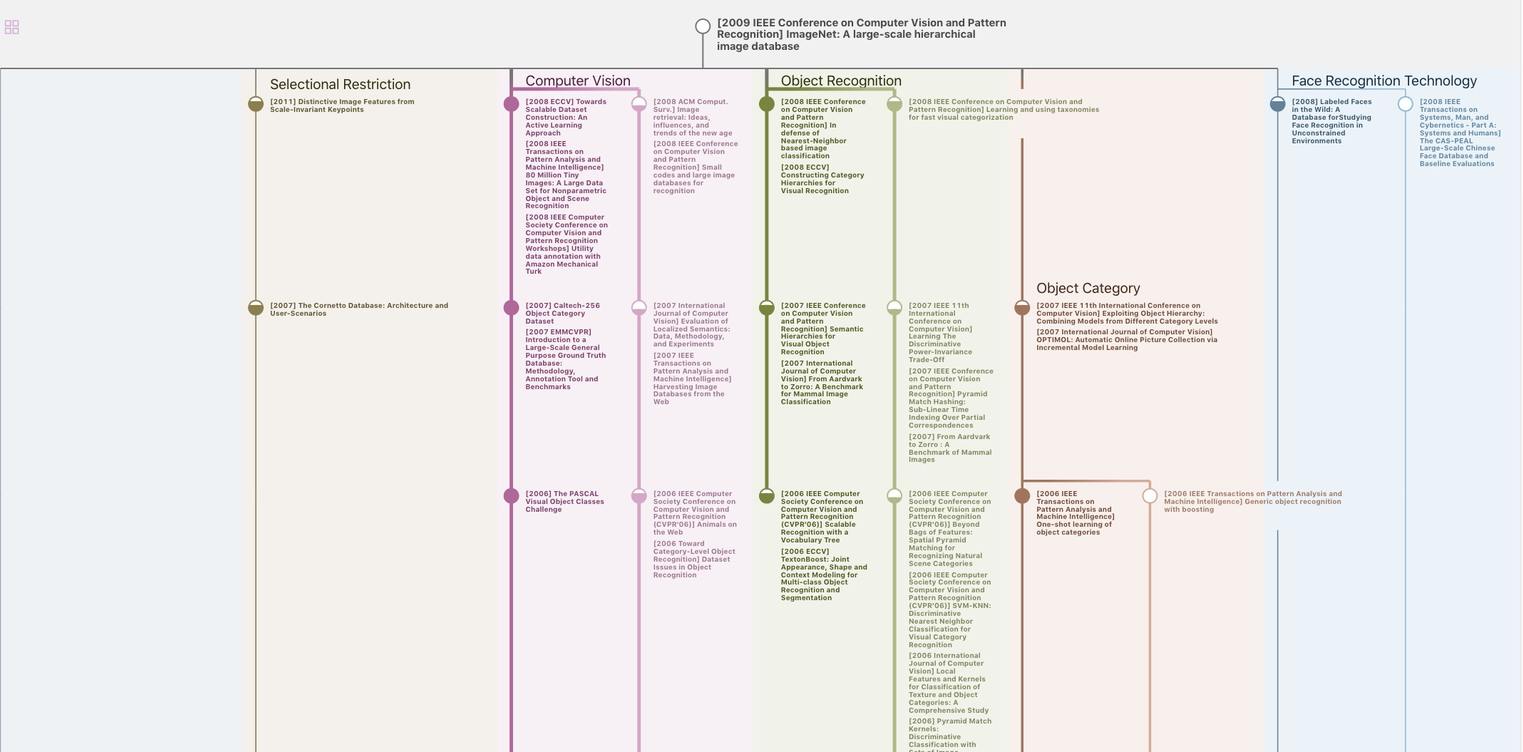
生成溯源树,研究论文发展脉络
Chat Paper
正在生成论文摘要