Discover novel disease-associated genes based on regulatory networks of long-range chromatin interactions
METHODS(2021)
Abstract
Identifying genes and non-coding genetic variants that are genetically associated with complex diseases and the underlying mechanisms is one of the most important questions in functional genomics. Due to the limited statistical power and the lack of mechanistic modeling, traditional genome-wide association studies (GWAS) is restricted to fully address this question. Based on multi-omics data integration, cell-type specific regulatory networks can be built to improve GWAS analysis. In this study, we developed a new computational infrastructure, APRIL, to incorporate 3D chromatin interactions into regulatory network construction, which can extend the networks to include long-range cis-regulatory links between non-coding GWAS SNPs and target genes. Combinatorial transcription factors that co-regulate groups of genes are also inferred to further expand the networks with trans-regulation. A suite of machine learning predictions and statistical tests are incorporated in APRIL to predict novel disease-associated genes based on the expanded regulatory networks. Important features of non-coding regulatory elements and genetic variants are prioritized in network-based predictions, providing systems-level insights on the mechanisms of transcriptional dysregulation associated with complex diseases.
MoreTranslated text
Key words
GWAS,Regulatory network,Long-range chromatin interaction,Disease-associated genetics,Epigenetics,Transcription factors
AI Read Science
Must-Reading Tree
Example
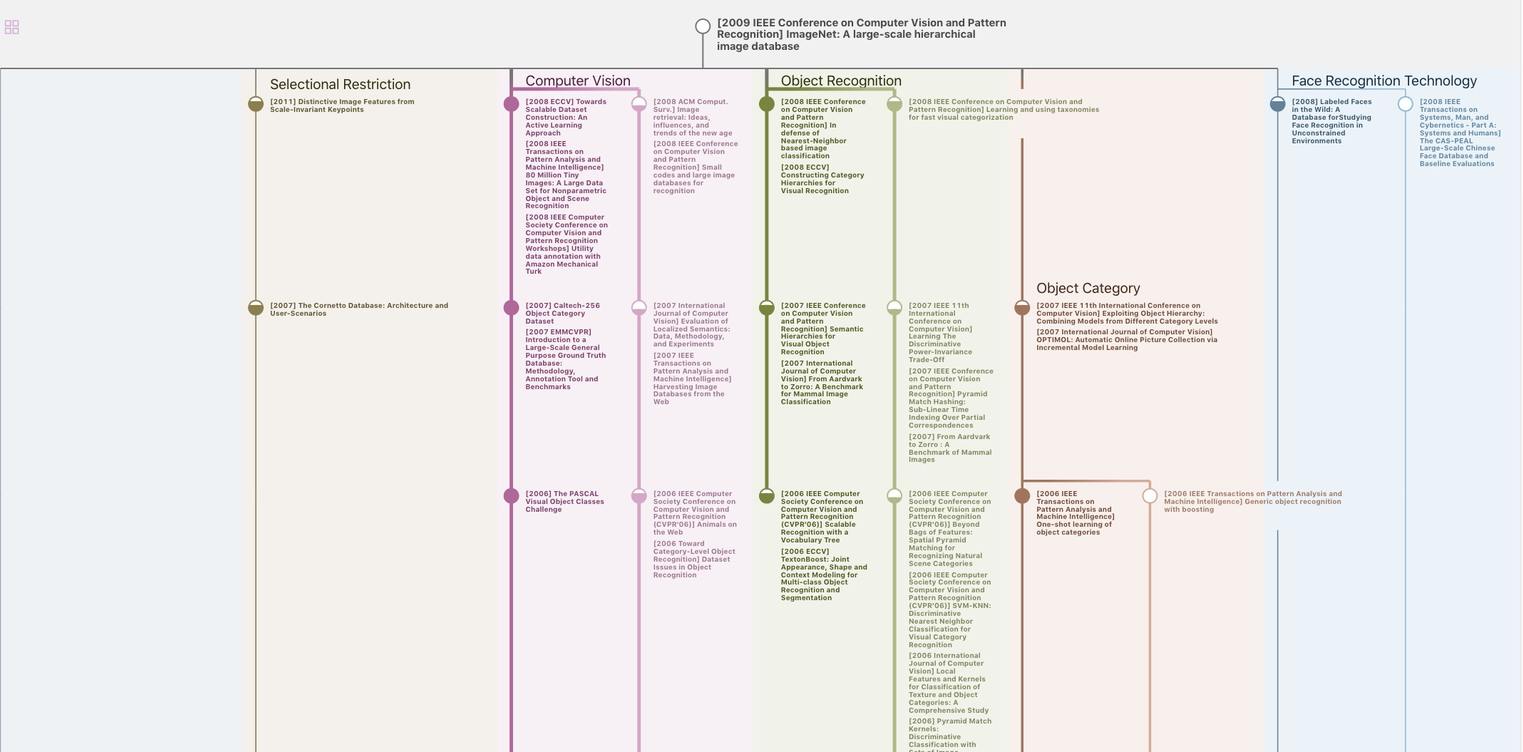
Generate MRT to find the research sequence of this paper
Chat Paper
Summary is being generated by the instructions you defined