ConVEx: Data-Efficient and Few-Shot Slot Labeling
NAACL-HLT(2020)
摘要
We propose ConVEx (Conversational Value Extractor), an efficient pretraining and fine-tuning neural approach for slot-labeling dialog tasks. Instead of relying on more general pretraining objectives from prior work (e.g., language modeling, response selection), ConVEx's pretraining objective, a novel pairwise cloze task using Reddit data, is well aligned with its intended usage on sequence labeling tasks. This enables learning domain-specific slot labelers by simply fine-tuning decoding layers of the pretrained general-purpose sequence labeling model, while the majority of the pretrained model's parameters are kept frozen. We report state-of-the-art performance of ConVEx across a range of diverse domains and data sets for dialog slot-labeling, with the largest gains in the most challenging, few-shot setups. We believe that ConVEx's reduced pretraining times (i.e., only 18 hours on 12 GPUs) and cost, along with its efficient fine-tuning and strong performance, promise wider portability and scalability for data-efficient sequence-labeling tasks in general.
更多查看译文
关键词
data-efficient,few-shot
AI 理解论文
溯源树
样例
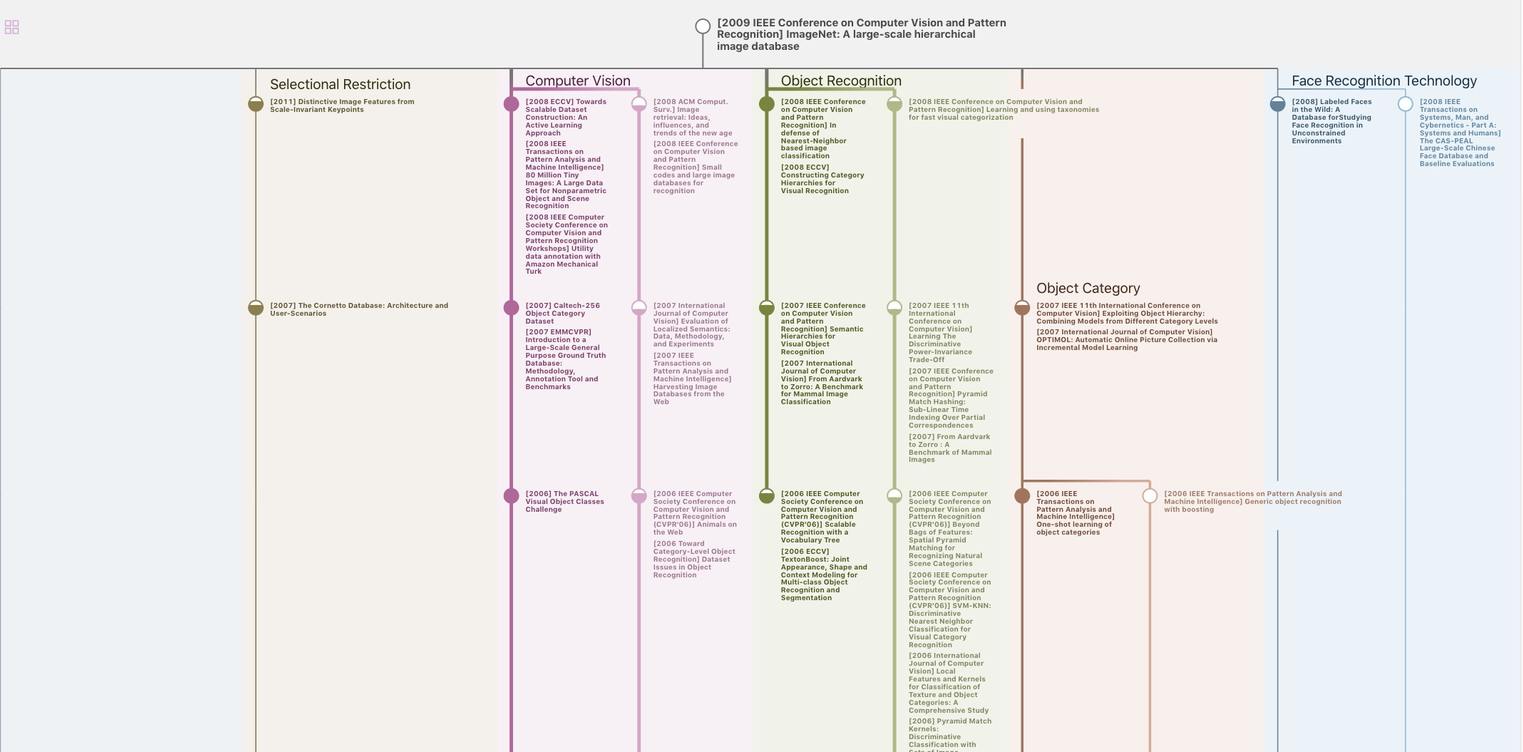
生成溯源树,研究论文发展脉络
Chat Paper
正在生成论文摘要