Fast and incremental loop closure detection with deep features and proximity graphs
JOURNAL OF FIELD ROBOTICS(2022)
摘要
In recent years, the robotics community has extensively examined methods concerning the place recognition task within the scope of simultaneous localization and mapping applications. This article proposes an appearance-based loop closure detection pipeline named "Fast and Incremental Loop closure Detection (FILD++). First, the system is fed by consecutive images and, via passing them twice through a single convolutional neural network, global and local deep features are extracted. Subsequently, a hierarchical navigable small-world graph incrementally constructs a visual database representing the robot's traversed path based on the computed global features. Finally, a query image, grabbed each time step, is set to retrieve similar locations on the traversed route. An image-to-image pairing follows, which exploits local features to evaluate the spatial information. Thus, in the proposed article, we propose a single network for global and local feature extraction in contrast to our previous work (FILD), while an exhaustive search for the verification process is adopted over the generated deep local features avoiding the utilization of hash codes. Exhaustive experiments on eleven publicly available data sets exhibit the system's high performance (achieving the highest recall score on eight of them) and low execution times (22.05 ms on average in New College, which is the largest one containing 52,480 images) compared to other state-of-the-art approaches.
更多查看译文
关键词
learned-based features, loop closure detection, mapping, navigable small-world graph indexing, visual-based navigation
AI 理解论文
溯源树
样例
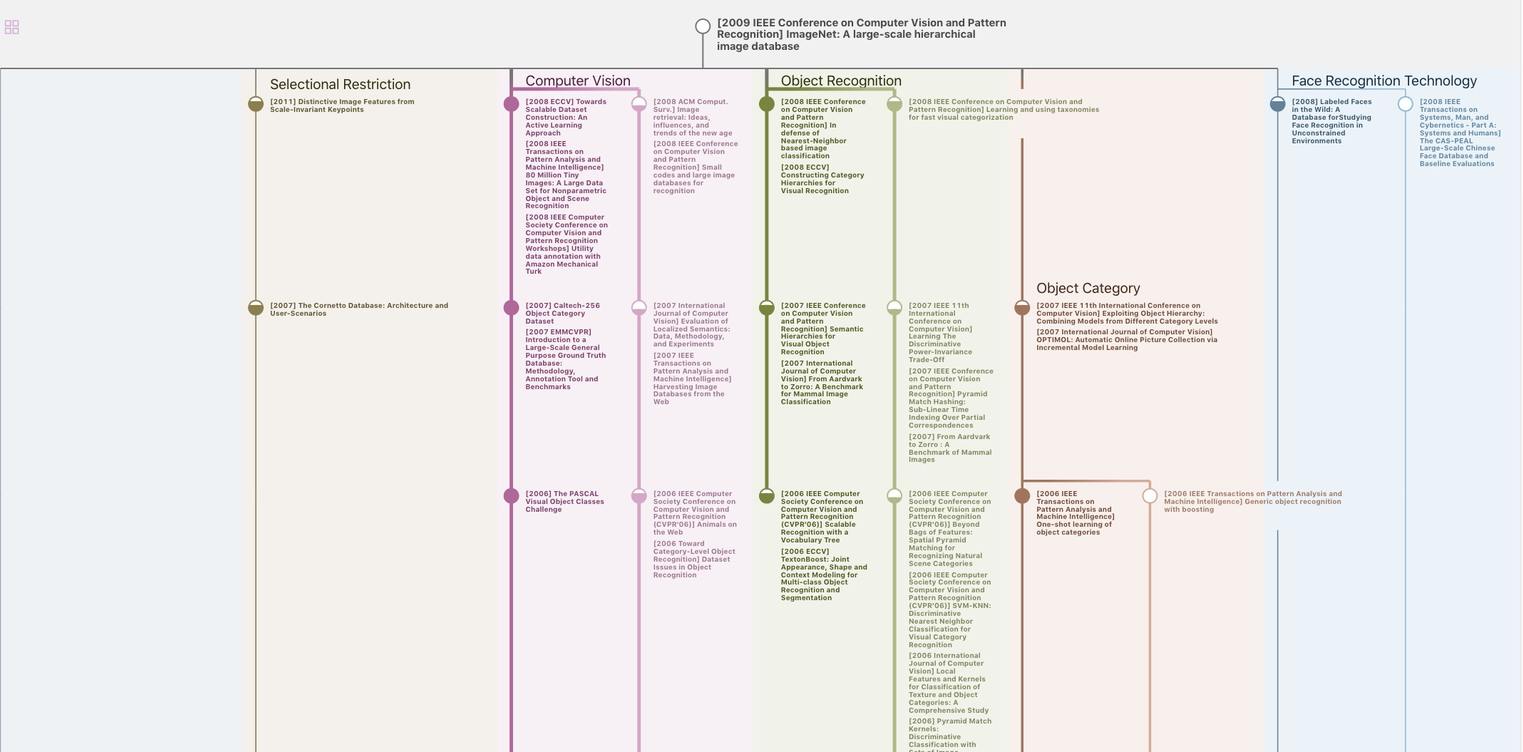
生成溯源树,研究论文发展脉络
Chat Paper
正在生成论文摘要