Fully Trainable and Interpretable Non Local Sparse Models for Image Restoration
European Conference on Computer Vision (ECCV)(2020)
摘要
Non-local self-similarity and sparsity principles have proven to be powerful priors for natural image modeling. We propose a novel differentiable relaxation of joint sparsity that exploits both principles and leads to a general framework for image restoration which is (1) trainable end to end,(2) fully interpretable, and (3) much more compact than competing deep learning architectures. We apply this approach to denoising, jpeg deblocking, and demosaicking, and show that, with as few as 100K parameters, its performance on several standard benchmarks is on par or better than state-of-the-art methods that may have an order of magnitude or more parameters.
更多查看译文
关键词
Sparse coding,Image processing,Structured sparsity
AI 理解论文
溯源树
样例
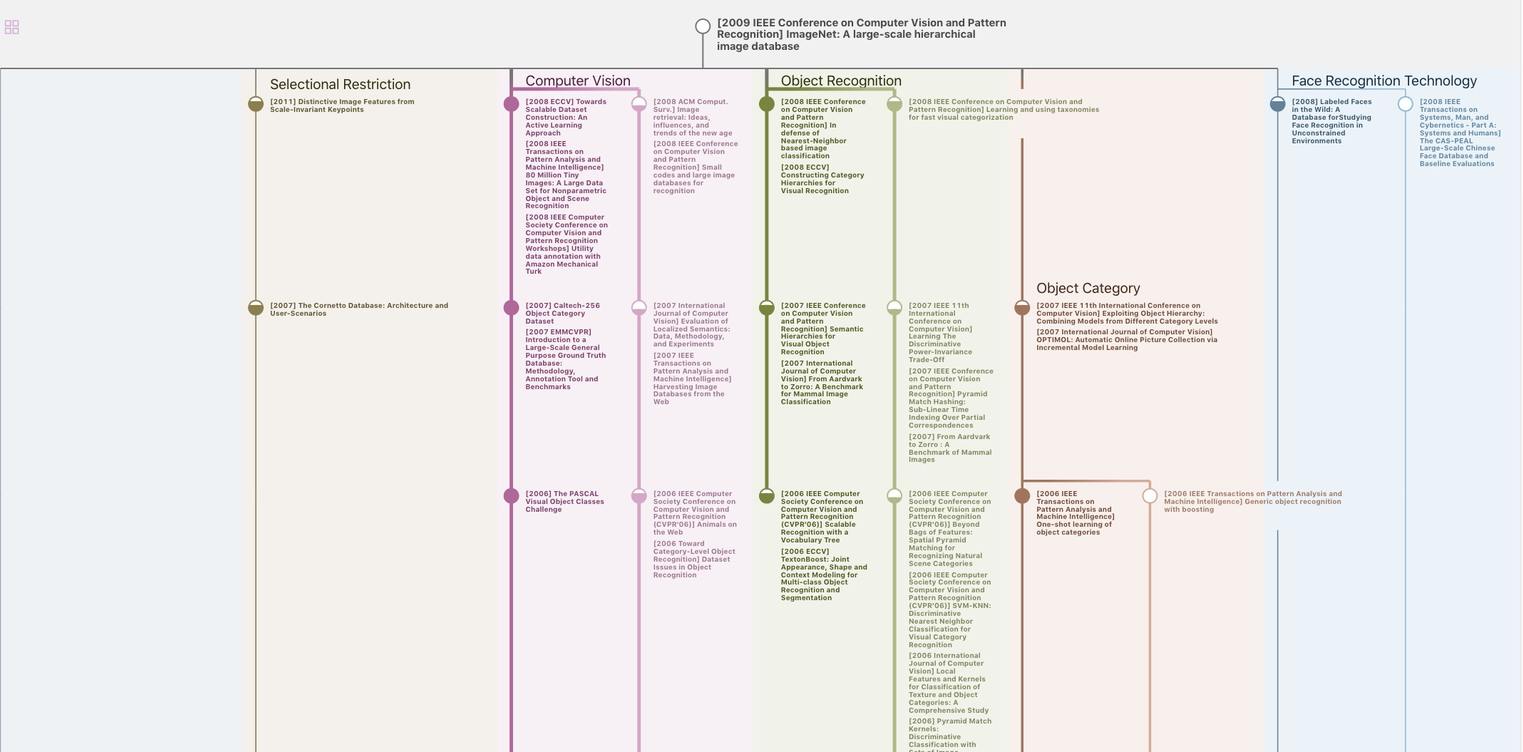
生成溯源树,研究论文发展脉络
Chat Paper
正在生成论文摘要