Provenance Graph Kernel
CoRR(2021)
摘要
Provenance is a record that describes how entities, activities, and agents have influenced a piece of data. Such provenance information is commonly represented in graphs with relevant labels on both their nodes and edges. With the growing adoption of provenance in a wide range of application domains, increasingly, users are confronted with an abundance of graph data, which may prove challenging to analyse. Graph kernels, on the other hand, have been consistently and successfully used to efficiently classify graphs. In this paper, we introduce a novel graph kernel called \emph{provenance kernel}, which is inspired by and tailored for provenance data. It decomposes a provenance graph into tree-patterns rooted at a given node and considers the labels of edges and nodes up to a certain distance from the root. We employ provenance kernels to classify provenance graphs from three application domains. Our evaluation shows that they perform well in terms of classification accuracy and yield competitive results when compared against standard graph kernel methods and the provenance network analytics method while taking significantly less time.Moreover, we illustrate how the provenance types used in provenance kernels help improve the explainability of predictive models.
更多查看译文
关键词
kernel
AI 理解论文
溯源树
样例
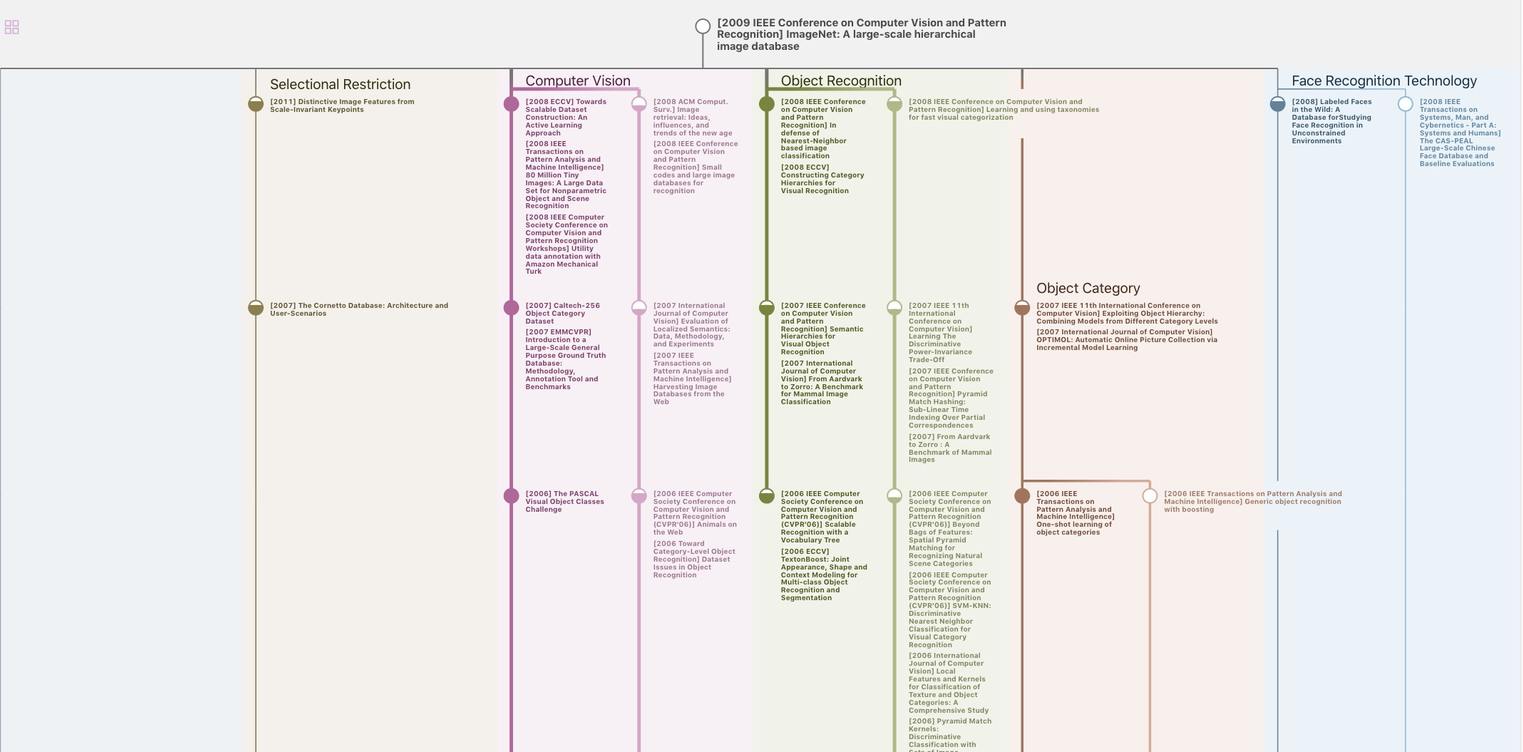
生成溯源树,研究论文发展脉络
Chat Paper
正在生成论文摘要