Very Fast Streaming Submodular Function Maximization
MACHINE LEARNING AND KNOWLEDGE DISCOVERY IN DATABASES, ECML PKDD 2021: RESEARCH TRACK, PT III(2021)
Abstract
Data summarization has become a valuable tool in understanding even terabytes of data. Due to their compelling theoretical properties, submodular functions have been the focus of summarization algorithms. Submodular function maximization is a well-studied problem with a variety of algorithms available. These algorithms usually offer worst-case guarantees to the expense of higher computation and memory requirements. However, many practical applications do not fall under this mathematical worst-case but are usually much more well-behaved. We propose a new submodular function maximization algorithm called ThreeSieves that ignores the worst-case and thus uses fewer resources. Our algorithm selects the most informative items from a data-stream on the fly and maintains a provable performance in most cases on a fixed memory budget. In an extensive evaluation, we compare our method against 6 state-of-the-art algorithms on 8 different datasets including data with and without concept drift. We show that our algorithm outperforms the current state-of-the-art in the majority of cases and, at the same time, uses fewer resources.
MoreTranslated text
Key words
Submodular function maximization,Streaming data,Data summarization
AI Read Science
Must-Reading Tree
Example
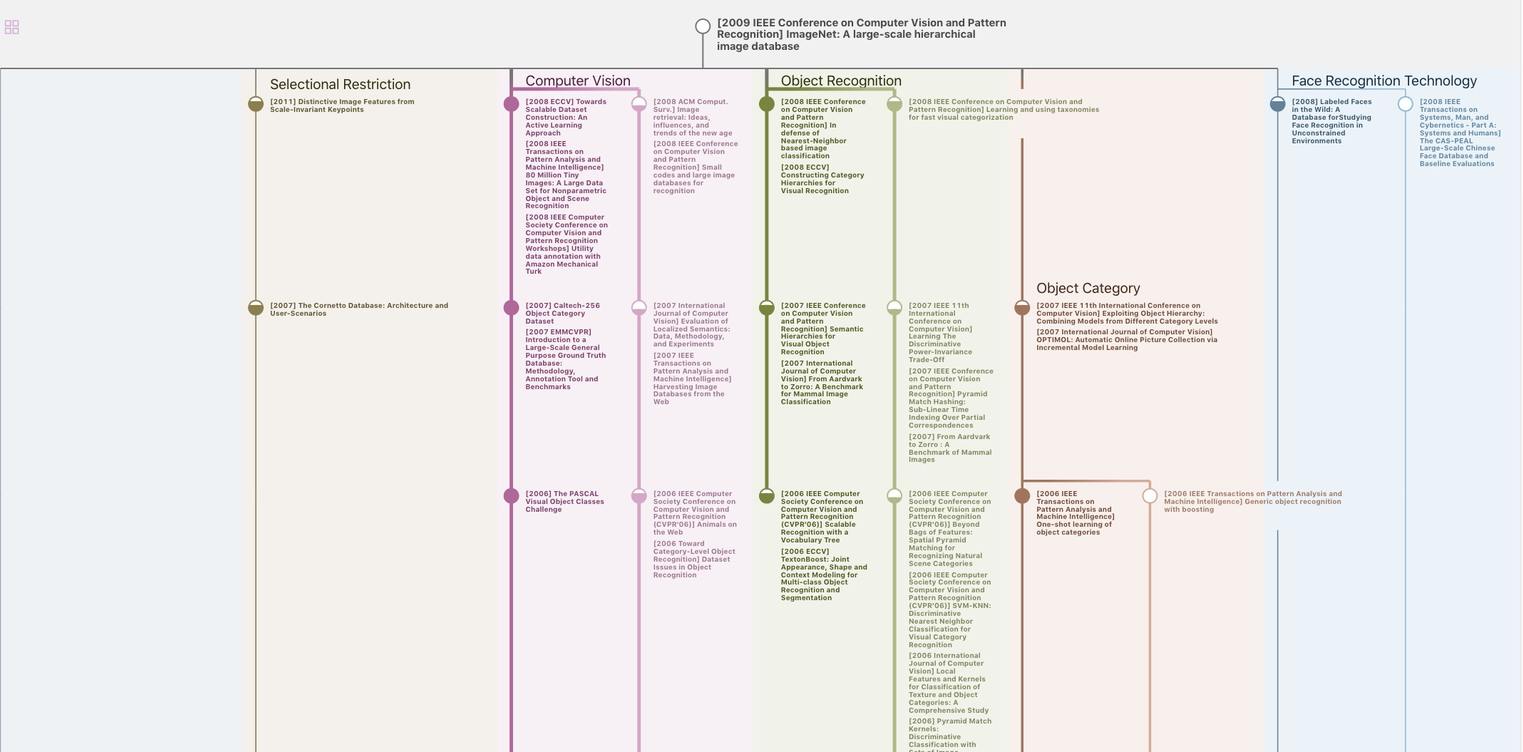
Generate MRT to find the research sequence of this paper
Chat Paper
Summary is being generated by the instructions you defined