Fast Uncertainty Estimation for Deep Learning Based Optical Flow
IROS(2020)
摘要
We present a novel approach to reduce the processing time required to derive the estimation uncertainty map in deep learning-based optical flow determination methods. Without uncertainty aware reasoning, the optical flow model, especially when it is used for mission critical fields such as robotics and aerospace, can cause catastrophic failures. Although several approaches such as the ones based on Bayesian neural networks have been proposed to handle this issue, they are computationally expensive. Thus, to speed up the processing time, our approach applies a generative model, which is trained by input images and an uncertainty map derived through a Bayesian approach. By using synthetically generated images of spacecraft, we demonstrate that the trained generative model can produce the uncertainty map 100~700 times faster than the conventional uncertainty estimation method used for training the generative model itself. We also show that the quality of uncertainty map derived by the generative model is close to that of the original uncertainty map. By applying the proposed approach, the deep learning model operated in real-time can avoid disastrous failures by considering the uncertainty as well as achieving better performance removing uncertain portions of the prediction result.
更多查看译文
关键词
fast uncertainty estimation,processing time,estimation uncertainty map,deep learning-based optical flow determination methods,uncertainty aware reasoning,optical flow model,mission critical fields,Bayesian neural networks,Bayesian approach,trained generative model,conventional uncertainty estimation method,original uncertainty map,deep learning model
AI 理解论文
溯源树
样例
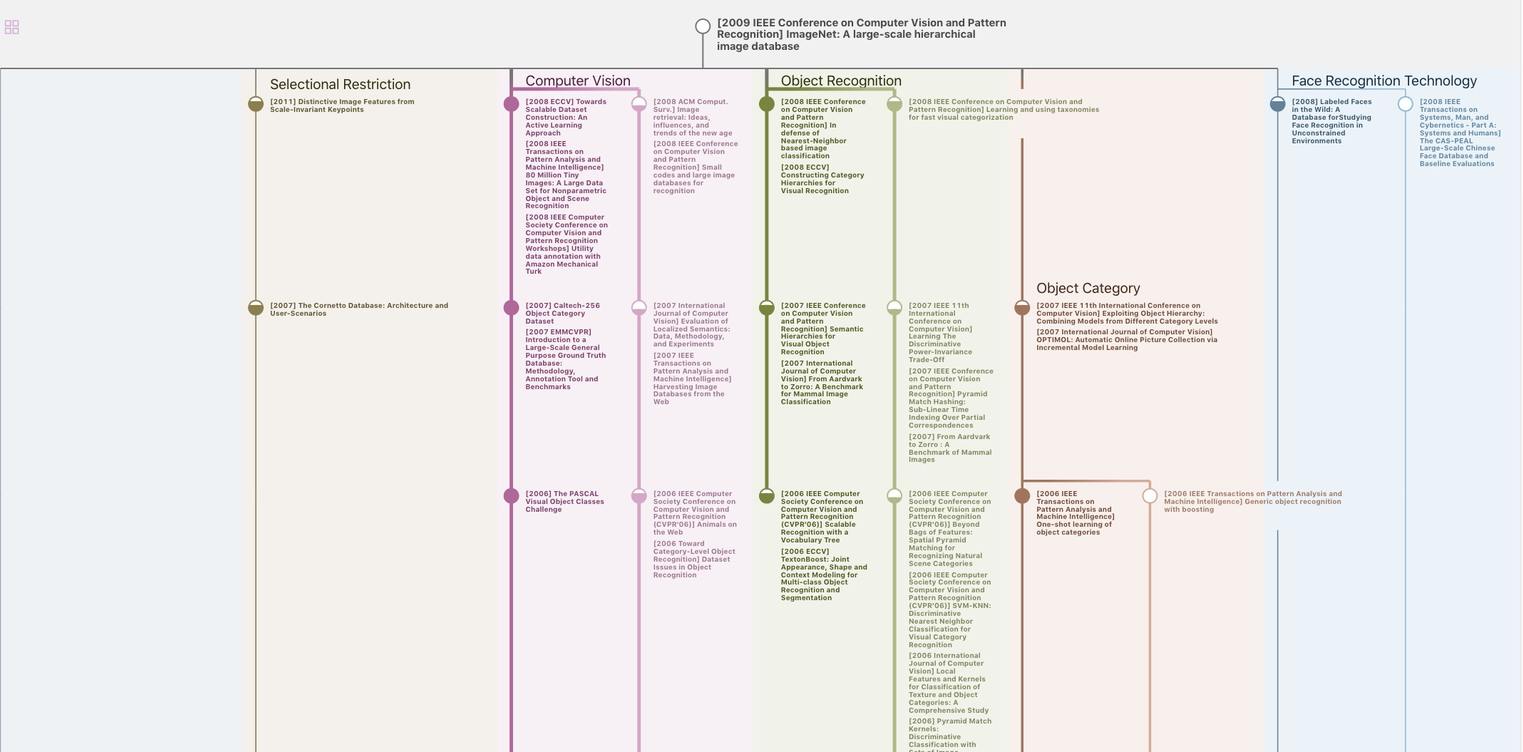
生成溯源树,研究论文发展脉络
Chat Paper
正在生成论文摘要