A Two-Stage Unsupervised Approach For Low Light Image Enhancement
IEEE ROBOTICS AND AUTOMATION LETTERS(2021)
摘要
As vision based perception methods are usually built on the normal light assumption, there will be a serious safety issue when deploying them into low light environments. Recently, deep learning based methods have been proposed to enhance low light images by penalizing the pixel-wise loss of low light and normal light images. However, most of them suffer from the following problems: 1) the need of pairs of low light and normal light images for training, 2) the poor performance for dark images, 3) the amplification of noise. To alleviate these problems, in this letter, we propose a two-stage unsupervised method that decomposes the low light image enhancement into a pre-enhancement and a post-refinement problem. In the first stage, we pre-enhance a low light image with a conventional Retinex based method. In the second stage, we use a refinement network learned with adversarial training for further improvement of the image quality. The experimental results show that our method outperforms previous methods on four benchmark datasets. In addition, we show that our method can significantly improve feature points matching and simultaneous localization and mapping in low light conditions.
更多查看译文
关键词
Low light image enhancement, robot's perception, SLAM, unsupervised method
AI 理解论文
溯源树
样例
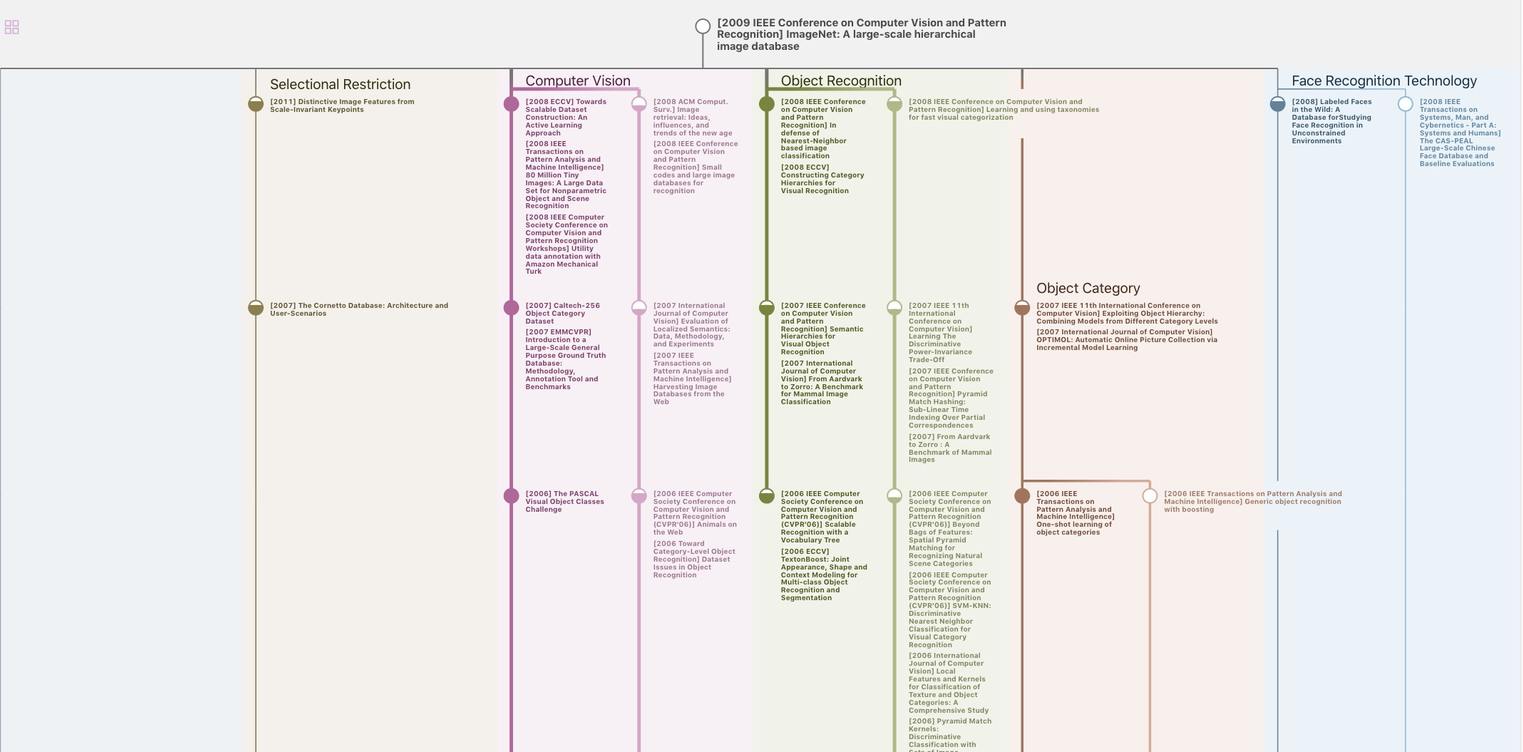
生成溯源树,研究论文发展脉络
Chat Paper
正在生成论文摘要