Multislice Left Ventricular Ejection Fraction Prediction From Cardiac Mris Without Segmentation Using Shared Sptdennet
COMPUTERIZED MEDICAL IMAGING AND GRAPHICS(2020)
摘要
We propose a spatiotemporal model for cardiac magnetic resonance images (MRI) named SptDenNet. The proposed model is based on DenseNet and extracts spatial and temporal features simultaneously to exploit threedimensional information on the heart over the cardiac loop cycle. To balance the model performance and efficiency, we construct a shared end-to-end framework, in which all frames of each selected short-axis (SAX) view slices are input to SptDenNet individually to extract spatiotemporal features. Then, the extracted features of all selected SAX view slices of a patient are concatenated and input to the subsequent fully connected layer and then a softmax layer to predict the left ventricular ejection fraction directly. To address the problem of class imbalance, we use FocalLoss function by reshaping the standard cross-entropy loss such that it down-weights the loss assigned to well-classified samples. We validate our proposed framework on the Second Annual Data Science Bowl dataset. Our prediction for the left ventricular ejection fraction obtains results comparable with state-ofthe-art end-to-end approaches but without segmentation. The average mean absolute error of the ejection fraction is 6.84. To further verify the effectiveness of the proposed framework, we use 4-chamber view images from the same dataset to predict the cardiac function; we obtain an accuracy of 86.07%. Our approach constructs an end-to-end model to predict the ejection fraction automatically without using image segmentation, which helps reduce manual work. Moreover, the proposed approach is computationally efficient.
更多查看译文
关键词
LV segmentation, LV Quantification, Ejection fraction, Spatiotemporal features, Cardiac MRIs
AI 理解论文
溯源树
样例
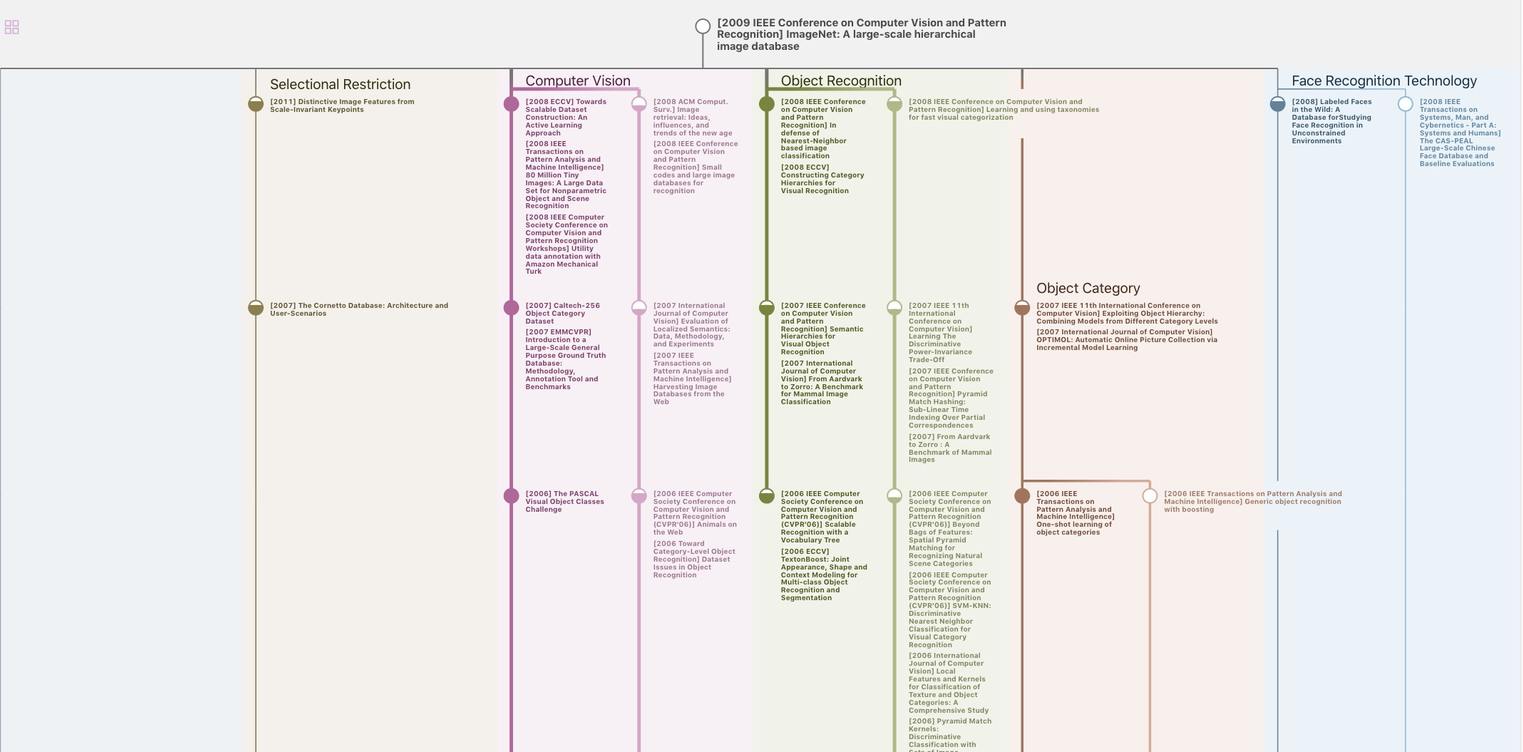
生成溯源树,研究论文发展脉络
Chat Paper
正在生成论文摘要