Reinforcement Learning for Efficient and Tuning-Free Link Adaptation
IEEE Transactions on Wireless Communications(2022)
摘要
Wireless links adapt the data transmission parameters to the dynamic channel state – this is called
link adaptation
. Classical link adaptation relies on tuning parameters that are challenging to configure for optimal link performance. Recently, reinforcement learning has been proposed to automate link adaptation, where the transmission parameters are modeled as discrete arms of a multi-armed bandit. In this context, we propose a latent learning model for link adaptation that exploits the correlation between data transmission parameters. Further, motivated by the recent success of Thompson sampling for multi-armed bandit problems, we propose a latent Thompson sampling (LTS) algorithm that quickly learns the optimal parameters for a given channel state. We extend LTS to fading wireless channels through a tuning-free mechanism that automatically tracks the channel dynamics. In numerical evaluations with fading wireless channels, LTS improves the link throughout by up to 100% compared to the state-of-the-art link adaptation algorithms.
更多查看译文
关键词
Wireless networks,adaptive modulation and coding,reinforcement learning,thompson sampling,outer loop link adaptation
AI 理解论文
溯源树
样例
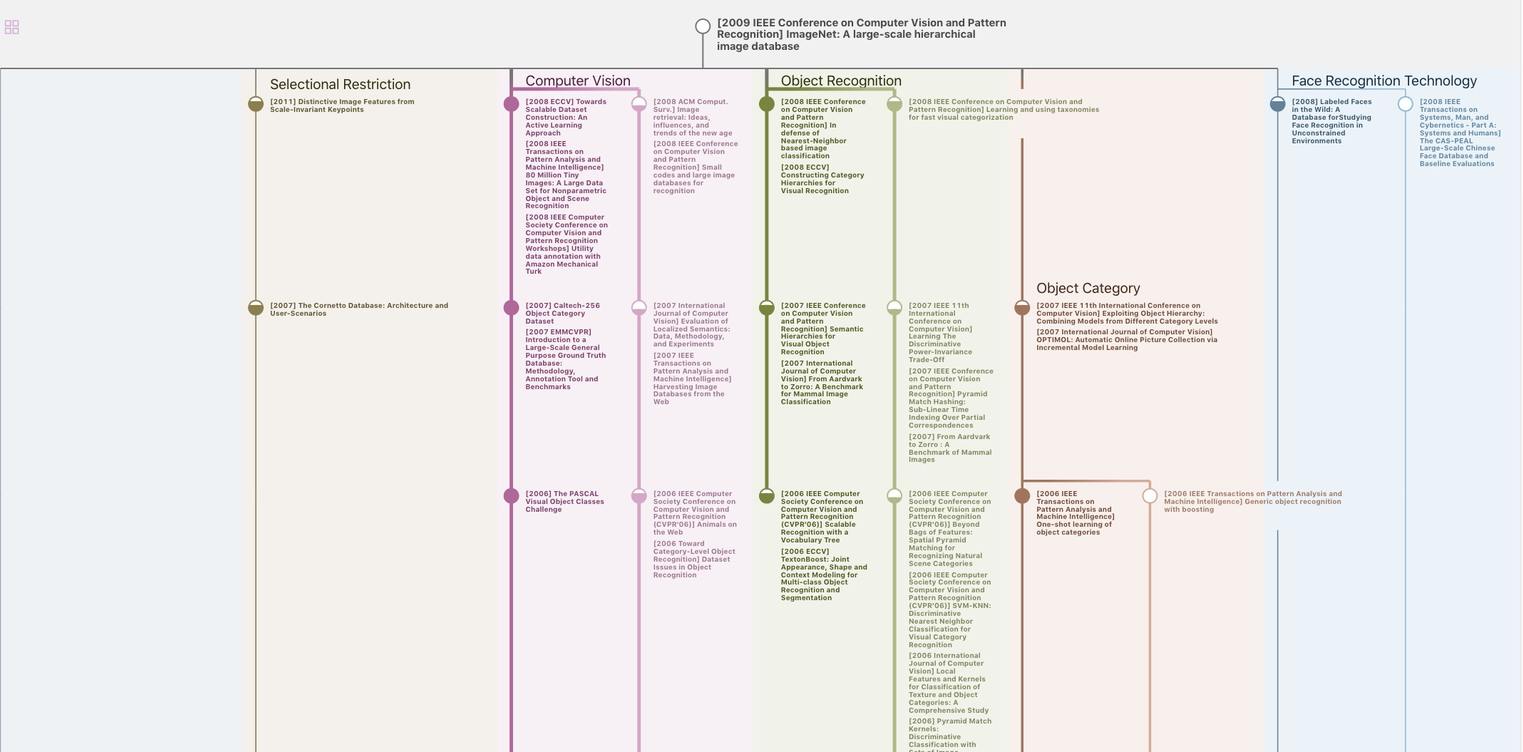
生成溯源树,研究论文发展脉络
Chat Paper
正在生成论文摘要