Evolving Bipartite Model Reveals the Bounded Weights in Mobile Social Networks
IEEE Transactions on Mobile Computing(2022)
摘要
Many realistic mobile social networks can be characterized by evolving bipartite graphs, in which dynamically added elements are divided into two entities and connected by links between these two entities, such as users and items in recommendation networks, authors and scientific topics in scholarly networks, male and female in dating social networks, etc. However, given the fact that connections between two entities are often weighted, how to mathematically model such weighted evolving bipartite relationships, along with quantitative characterizations, remains unexplored. Motivated by this, we develop a novel evolving bipartite model (EBM), which, based on empirically validated power-law distribution on multiple realistic mobile social networks, discloses that the distribution of total weights of incoming and outgoing edges in networks is determined by the weighting scale and bounded by certain ceilings and floors. Based on these theoretical results, for evolving bipartite networks whose degree follows power-law distribution, their overall weights of vertices can be predicted by EBM. To illustrate, in recommendation networks, the evaluation of items, i.e., total rating scores, can be estimated through the given bounds; in scholarly networks, the total numbers of publications under specific topics can be anticipated within a certain range; in dating social networks, the favorability of male/female can be roughly measured. Finally, we perform extensive experiments on 10 realistic datasets and a synthetic network with varying weights, i.e., rating scales, to further evaluate the performance of EBM, and experimental results demonstrate that given weighting scales, both the upper bound and the lower bound of total weights of vertices in mobile social networks can be properly predicted by the EBM.
更多查看译文
关键词
Evolving bipartite model,mobile social network,theoretical bound
AI 理解论文
溯源树
样例
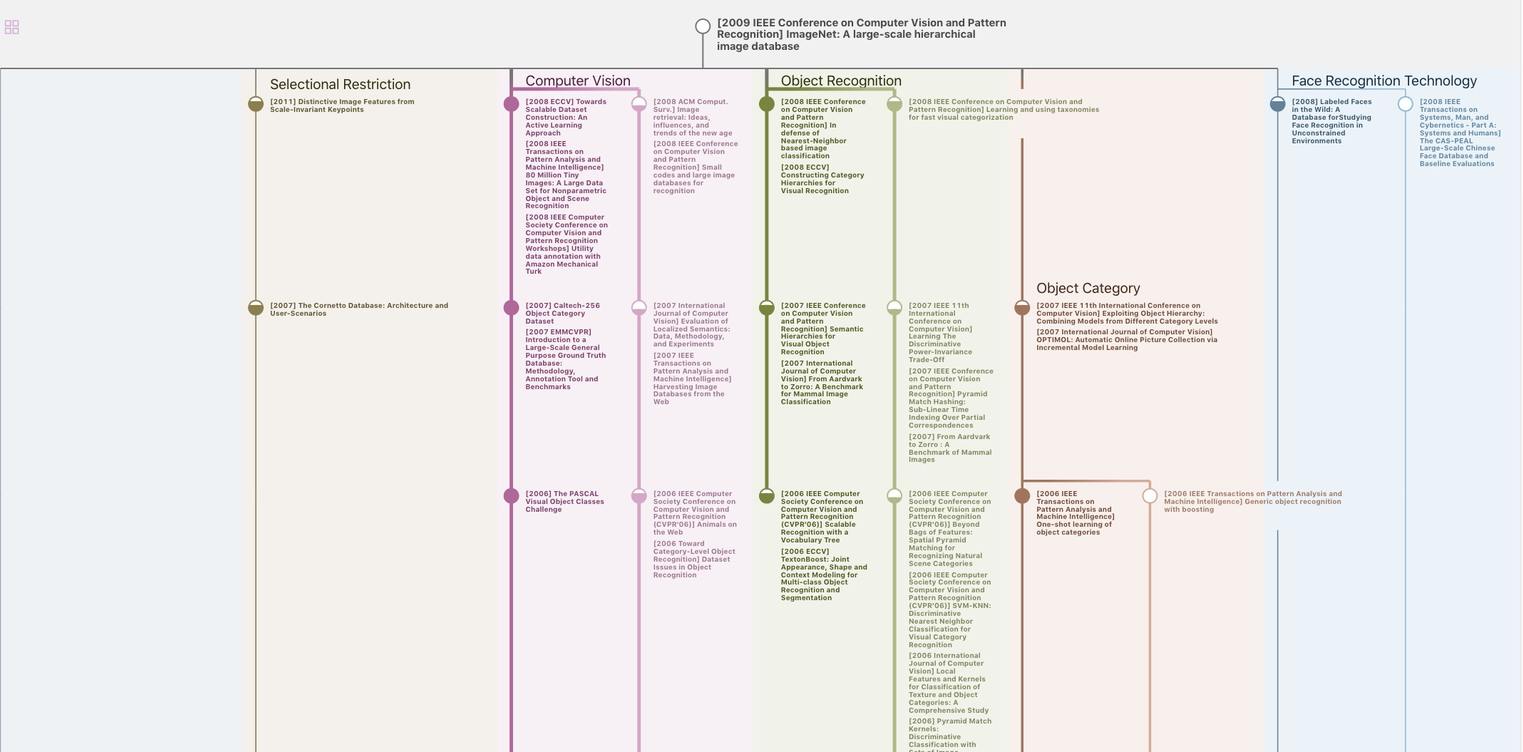
生成溯源树,研究论文发展脉络
Chat Paper
正在生成论文摘要