PSTIE: Time Information Enhanced Personalized Search
CIKM '20: The 29th ACM International Conference on Information and Knowledge Management Virtual Event Ireland October, 2020(2020)
摘要
Personalized search aims to improve the search quality by re-ranking the candidate document list based on user's historical behavior. Existing approaches focus on modeling the order information of user's search history by sequential methods such as Recurrent Neural Network (RNN). However, these methods usually ignore the fine-grained time information associated with user actions. In fact, the time intervals between queries can help to capture the evolution of query intent and document interest of users. Besides, the time intervals between past actions and current query can reflect the re-finding tendency more accurately than discrete steps in RNN. In this paper, we propose PSTIE, a fine-grained Time Information Enhanced model to construct more accurate user interest representations for Personalized Search. To capture the short-term interest of users, we design time-aware LSTM architectures for modeling the subtle interest evolution of users in continuous time. We further leverage time in calculating the re-finding possibility of users to capture the long-term user interest. We propose two methods to utilize the time-enhanced user interest into personalized ranking. Experiments on two datasets show that PSTIE can effectively improve the ranking quality over state-of-the-art models.
更多查看译文
关键词
Personalized Search, Time Information, User Interest, Re-finding
AI 理解论文
溯源树
样例
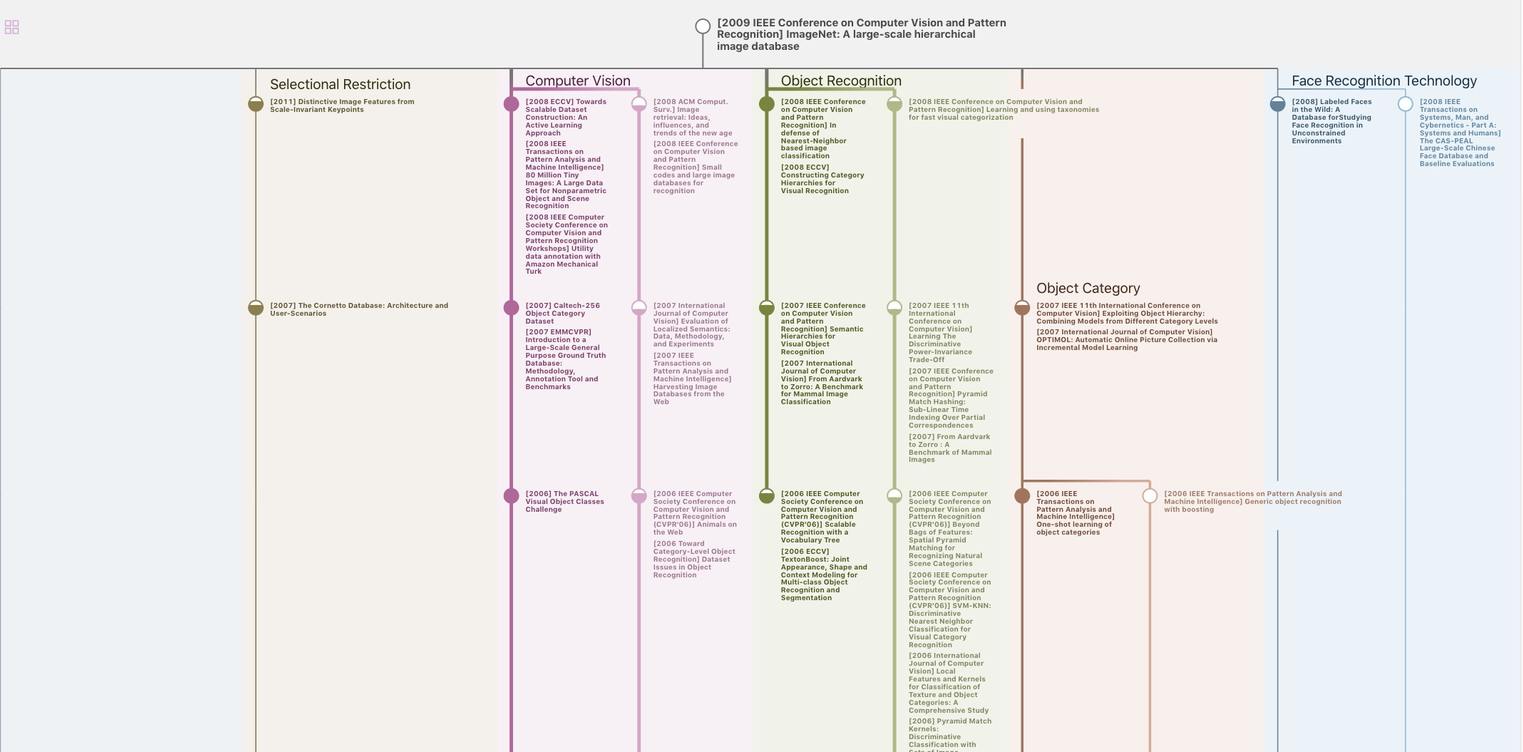
生成溯源树,研究论文发展脉络
Chat Paper
正在生成论文摘要