Non-saturating GAN training as divergence minimization
arxiv(2020)
摘要
Non-saturating generative adversarial network (GAN) training is widely used and has continued to obtain groundbreaking results. However so far this approach has lacked strong theoretical justification, in contrast to alternatives such as f-GANs and Wasserstein GANs which are motivated in terms of approximate divergence minimization. In this paper we show that non-saturating GAN training does in fact approximately minimize a particular f-divergence. We develop general theoretical tools to compare and classify f-divergences and use these to show that the new f-divergence is qualitatively similar to reverse KL. These results help to explain the high sample quality but poor diversity often observed empirically when using this scheme.
更多查看译文
关键词
divergence minimization,training,non-saturating
AI 理解论文
溯源树
样例
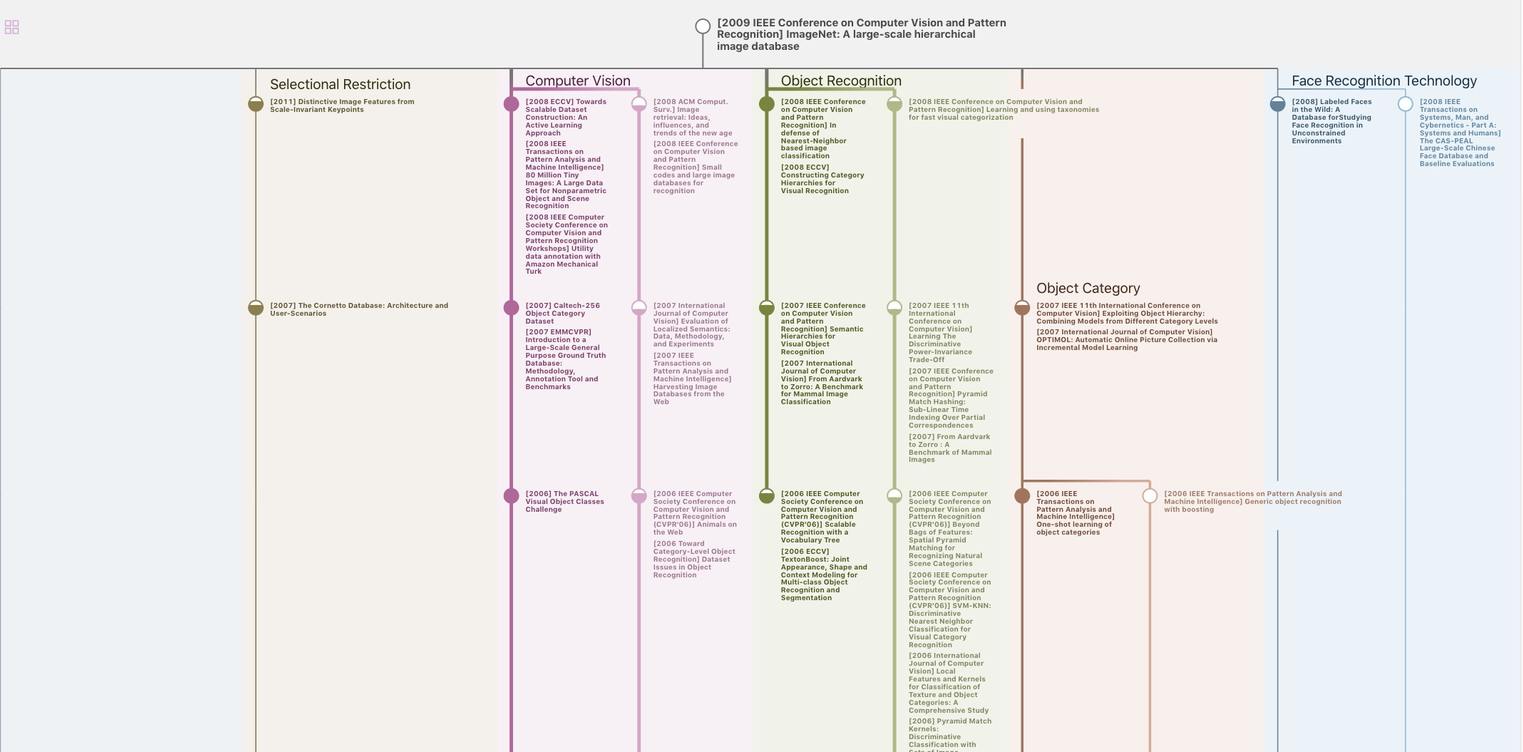
生成溯源树,研究论文发展脉络
Chat Paper
正在生成论文摘要