An empowerment-based solution to robotic manipulation tasks with sparse rewards
ROBOTICS: SCIENCE AND SYSTEM XVII(2023)
摘要
In order to provide adaptive and user-friendly solutions to robotic manipulation, it is important that the agent can learn to accomplish tasks even if they are only provided with very sparse instruction signals. To address the issues reinforcement learning algorithms face when task rewards are sparse, this paper proposes an intrinsic motivation approach that can be easily integrated into any standard reinforcement learning algorithm and can allow robotic manipulators to learn useful manipulation skills with only sparse extrinsic rewards. Through integrating and balancing empowerment and curiosity, this approach shows superior performance compared to other state-of-the-art intrinsic exploration approaches during extensive empirical testing. When combined with other strategies for tackling the exploration challenge, e.g. curriculum learning, our approach is able to further improve the exploration efficiency and task success rate. Qualitative analysis also shows that when combined with diversity-driven intrinsic motivations, this approach can help manipulators learn a set of diverse skills which could potentially be applied to other more complicated manipulation tasks and accelerate their learning process.
更多查看译文
关键词
Reinforcement learning, Intrinsic exploration, Robotic manipulation, Empowerment
AI 理解论文
溯源树
样例
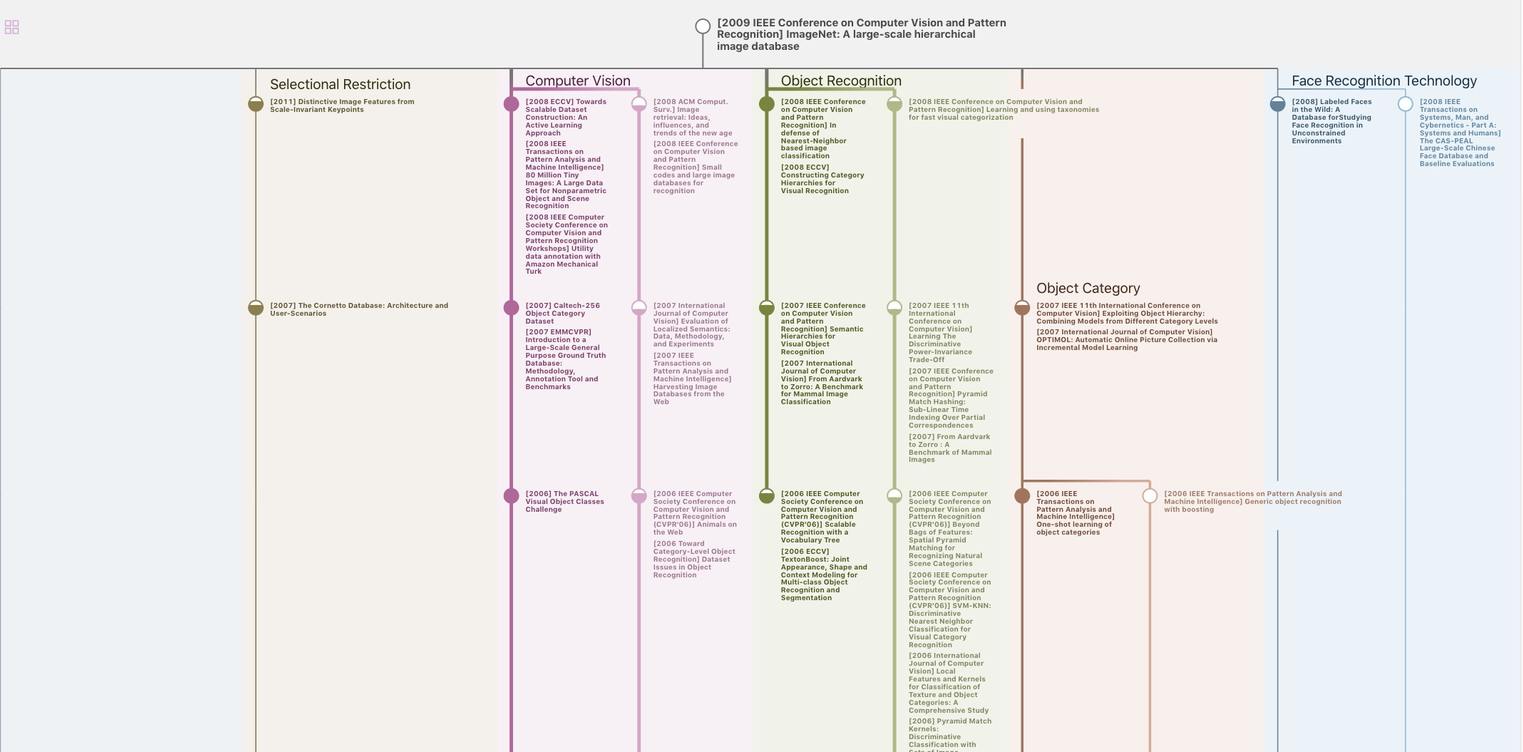
生成溯源树,研究论文发展脉络
Chat Paper
正在生成论文摘要