Estimating Wildfire Smoke Concentrations During The October 2017 California Fires Through Bme Space/Time Data Fusion Of Observed, Modeled, And Satellite-Derived Pm2.5
ENVIRONMENTAL SCIENCE & TECHNOLOGY(2020)
Abstract
Exposure to wildfire smoke causes adverse health outcomes, suggesting the importance of accurately estimating smoke concentrations. Geostatistical methods can combine observed, modeled, and satellite-derived concentrations to produce accurate estimates. Here, we estimate daily average ground-level PM2.5 concentrations at a 1 km resolution during the October 2017 California wildfires, using the Constant Air Quality Model Performance (CAMP) and Bayesian Maximum Entropy (BME) methods to bias-correct and fuse three concentration datasets: permanent and temporary monitoring stations, a chemical transport model (CTM), and satellite-derived estimates. Four BME space/time kriging and data fusion methods were evaluated. All BME methods produce more accurate estimates than the standalone CTM and satellite products. Adding temporary station data increases the R-2 by 36%. The data fusion of observations with the CAMP-corrected CTM and satellite-derived concentrations provides the best estimate (R-2 = 0.713) in fire-impacted regions, emphasizing the importance of combining multiple datasets. We estimate that approximately 65,000 people were exposed to very unhealthy air (daily average PM2.5 >= 150.5 mu g/m(3)).
MoreTranslated text
Key words
wildfire smoke concentrations,california fires,space/time data fusion,bme space/time,satellite-derived
AI Read Science
Must-Reading Tree
Example
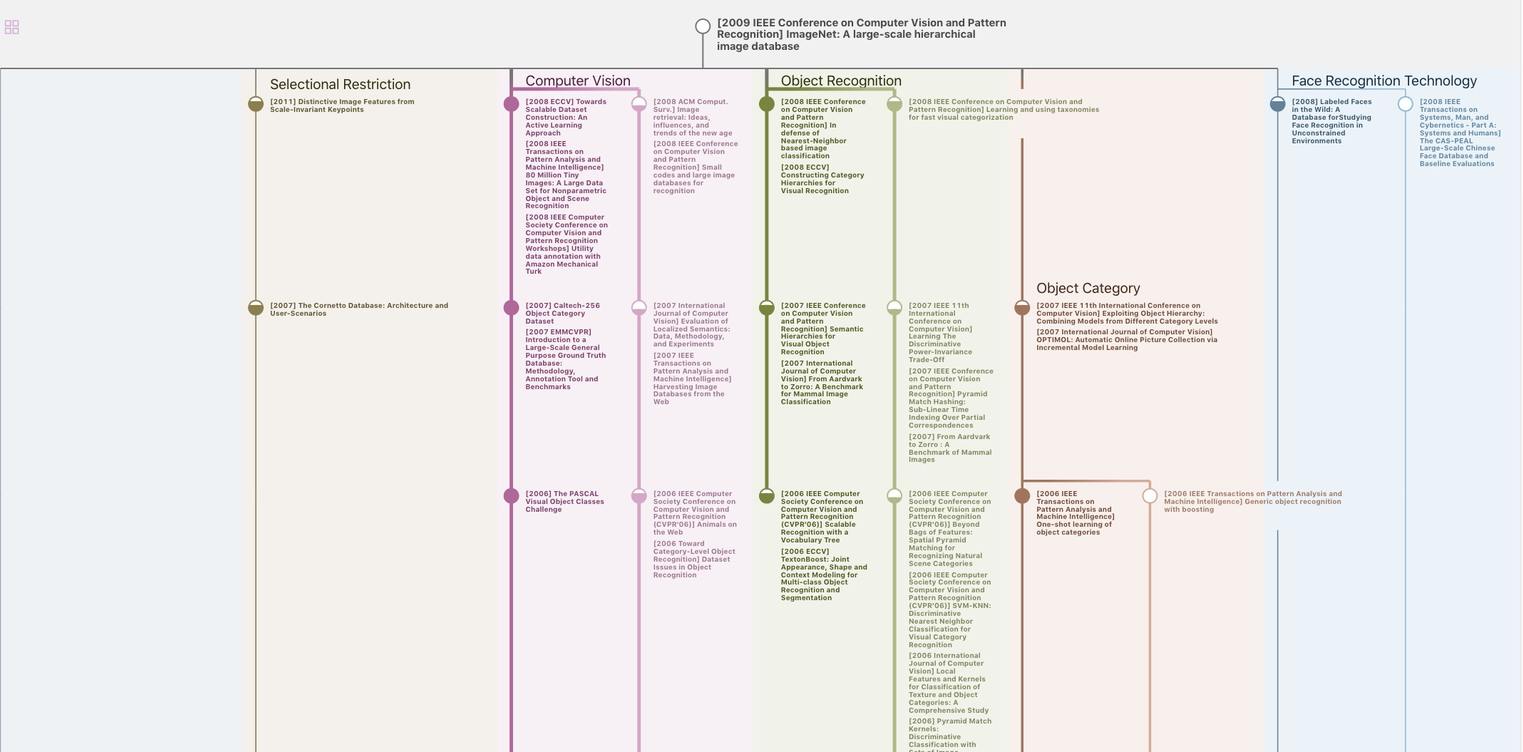
Generate MRT to find the research sequence of this paper
Chat Paper
Summary is being generated by the instructions you defined