Temporal Hierarchical Dictionary Guided Decoding For Online Gesture Segmentation And Recognition
IEEE TRANSACTIONS ON IMAGE PROCESSING(2020)
摘要
Online segmentation and recognition of skeletonbased gestures are challenging. Compared with offline cases, the inference of online settings can only rely on the current few frames and always completes before whole temporal movements are performed. However, incompletely performed gestures are ambiguous and their early recognition is easy to fall into local optimum. In this work, we address the problem with a temporal hierarchical dictionary to guide the hidden Markov model (HMM) decoding procedure. The intuition is that, gestures are ambiguous with high uncertainty at early performing phases, and only become discriminate after certain phases. This uncertainty naturally can be measured by entropy. Thus, we propose a measurement called "relative entropy map" (REM) to encode this temporal context to guide HMM decoding. Furthermore, we introduce a progressive learning strategy with which neural networks could learn a robust recognition of HMM states in an iterative manner. The performance of our method is intensively evaluated on three challenging databases and achieves state-of-the-art results. Our method shows the abilities of both extracting the discriminate connotations and reducing large redundancy in the HMM transition process. It is verified that our framework can achieve online recognition of continuous gesture streams even when they are halfway performed.
更多查看译文
关键词
Hidden Markov models, Dictionaries, Entropy, Decoding, Skeleton, Three-dimensional displays, Neural networks, Temporal context, hidden Markov model, hierarchical structure, deep neural network, relative entropy, skeleton-based recognition
AI 理解论文
溯源树
样例
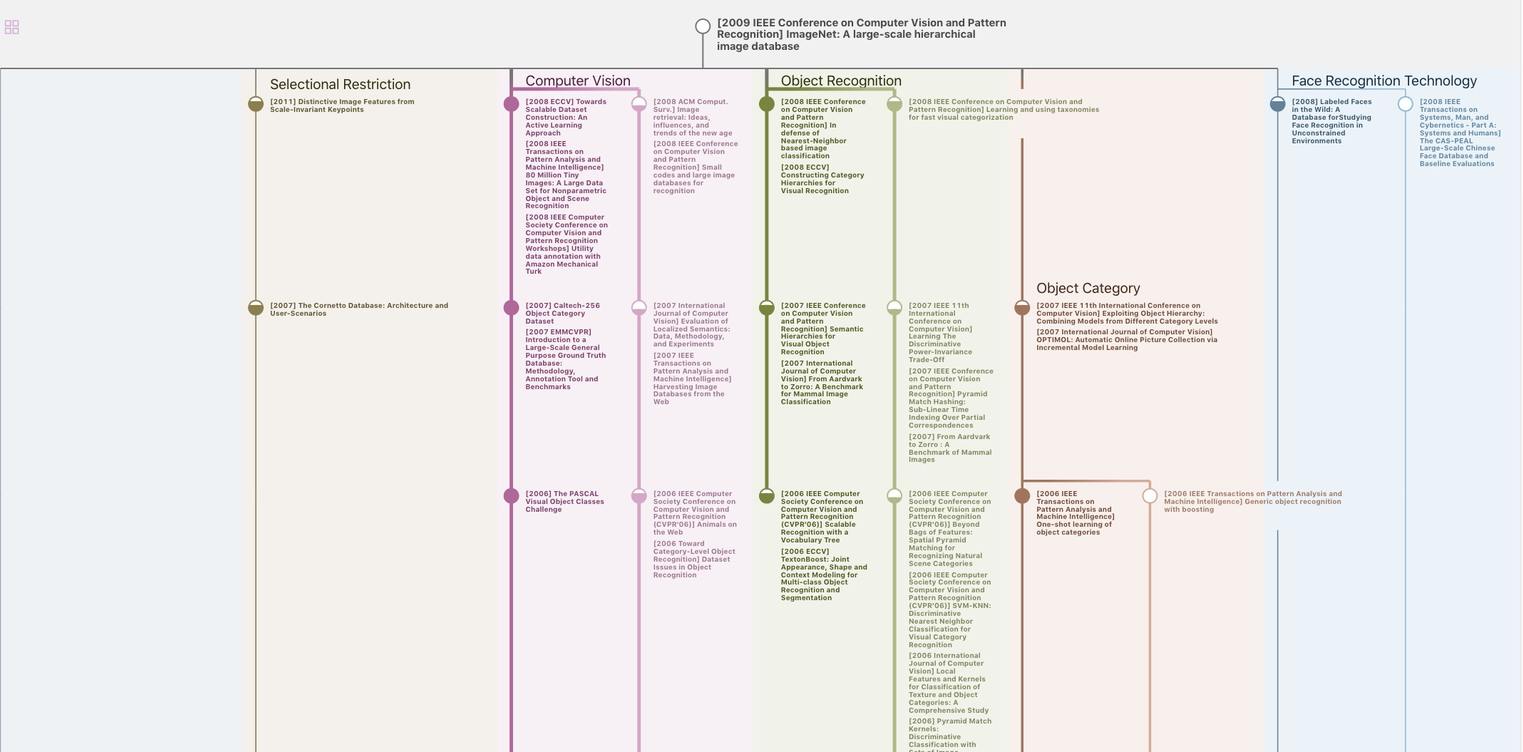
生成溯源树,研究论文发展脉络
Chat Paper
正在生成论文摘要