Early Prediction Of Sepsis From Clinical Data Using Ratio And Power-Based Features
CRITICAL CARE MEDICINE(2020)
摘要
Objectives:Early prediction of sepsis is of utmost importance to provide optimal care at an early stage. This work aims to deploy soft-computing and machine learning techniques for early prediction of sepsis.Design:An algorithm for early identification of sepsis using ratio and power-based feature transformation of easily obtainable clinical data.Setting:PhysioNet Challenge 2019 provided ICU data from three separate hospital systems. Publicly shared data from two hospital systems are used for training and validation purposes, whereas sequestered data from all the three systems is used for testing.Patients:Over 60,000 ICU patients with up to 40 clinical variables are sourced for each hour of their ICU stay. The Sepsis-3 criterion is applied for annotation.Interventions:None.Measurements and Main Results:The clinical feature exploration for early prediction of sepsis is achieved using the proposed framework named genetic algorithm optimized ratio and power-based expert algorithm. An optimal feature set containing 46 ratio and power-based features is computed from the given patient covariates using genetic algorithm optimized ratio and power-based expert and grouped with identified 17 raw features and 55 statistical features to form a final feature set of 118 clinical features to predict the onset of sepsis in the proceeding 6 hours. The obtained features are fed to a hybrid Random Under-Sampling-Boosting algorithm, called RUSBoost for alleviating the involved class imbalance. The optimal RUSBoost model has achieved a normalized utility score of 0.318 on full test data.Conclusions:The proposed study supports the realization of a hospital-specific customized solution in the form of an early-warning system for sepsis. However, an extended analysis is necessary to apply this framework for hospital-independent diagnosis of sepsis in general. Nevertheless, the clinical utility of hospital-specific customized solutions based on the proposed method across a wide range of hospital systems needs to be studied.
更多查看译文
关键词
early prediction, feature extraction, genetic algorithm, machine learning, epsis
AI 理解论文
溯源树
样例
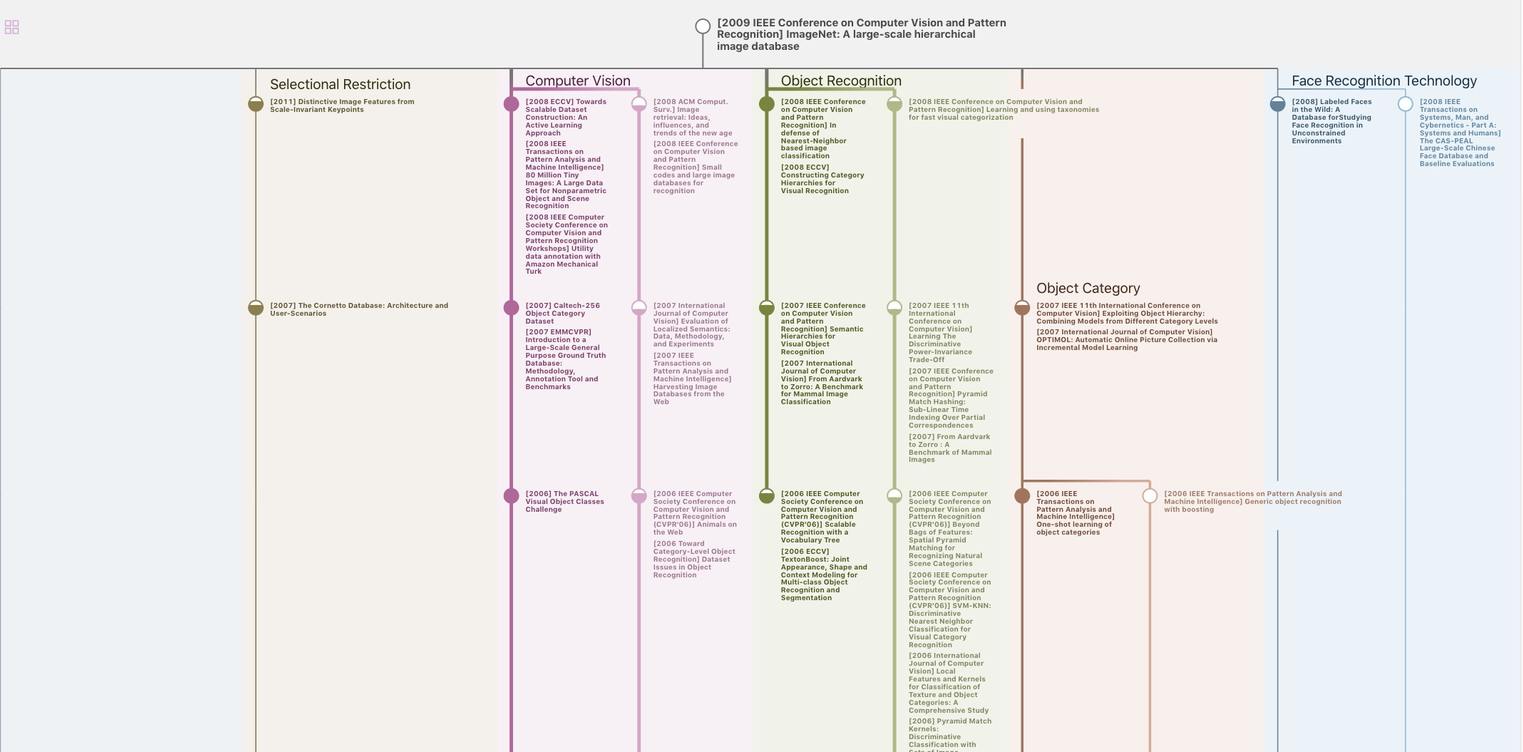
生成溯源树,研究论文发展脉络
Chat Paper
正在生成论文摘要