Non-Bayesian Social Learning on Random Digraphs With Aperiodically Varying Network Connectivity
IEEE Transactions on Control of Network Systems(2022)
Abstract
In this article, we study non-Bayesian social learning on random directed graphs and show that under mild connectivity assumptions, all the agents almost surely learn the true state of the world asymptotically in time if the sequence of the associated weighted adjacency matrices belongs to Class
$\mathcal {P}^*$
(a broad class of stochastic chains that subsumes uniformly strongly connected chains). We show that uniform strong connectivity, while being unnecessary for asymptotic learning, ensures that all the agents’ beliefs converge to a consensus almost surely, even when the true state is not identifiable. We then provide a few corollaries of our main results, some of which apply to the variants of the original update rule, such as inertial non-Bayesian learning and learning via diffusion and adaptation. Others include the extensions of known results on social learning. We also show that if the network of influences is balanced in a certain sense, then asymptotic learning occurs almost surely even in the absence of uniform strong connectivity.
MoreTranslated text
Key words
Distributed algorithms,multiagent systems,network theory (graphs),networked control systems,stochastic systems
AI Read Science
Must-Reading Tree
Example
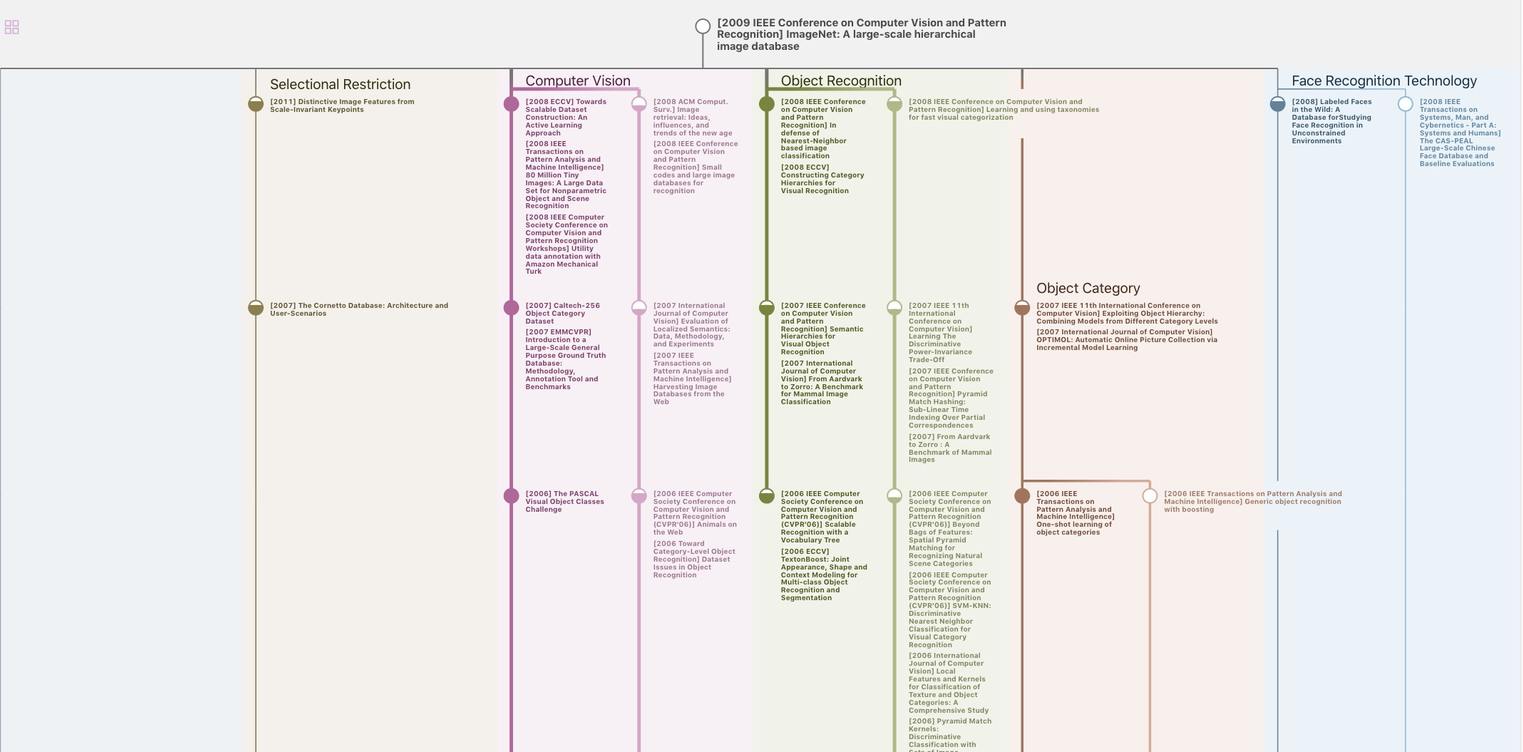
Generate MRT to find the research sequence of this paper
Chat Paper
Summary is being generated by the instructions you defined