Weakly Supervised Real-time Image Cropping based on Aesthetic Distributions
MM '20: The 28th ACM International Conference on Multimedia Seattle WA USA October, 2020(2020)
摘要
Image cropping is an effective tool to edit and manipulate images to achieve better aesthetic quality. Most existing cropping approaches rely on the two-step paradigm where multiple candidate cropping areas are proposed initially and the optimal cropping window is determined based on some quality criteria for these candidates afterwards. The obvious disadvantage of this mechanism is its low efficiency due to the huge searching space of candidate crops. In order to tackle this problem, a weakly supervised cropping framework is proposed, where the distribution dissimilarity between high quality images and cropped images is used to guide the coordinate predictor's training and the ground truths of cropping windows are not required by the proposed method. Meanwhile, to improve the cropping performance, a saliency loss is also designed in the proposed framework to force the neural network to focus more on the interested objects in the image. Under this framework, the images can be cropped effectively by the trained coordinate predictor in a one-pass favor without multiple candidates proposals, which ensures the high efficiency of the proposed system . Also, based on the proposed framework, many existing distribution dissimilarity measurements can be applied to train the image cropping system with high flexibility, such as likelihood based and divergence based distribution dissimilarity measure proposed in this work. The experiments on the public databases show that the proposed cropping method achieves the state-of-the-art accuracy, and the high computation efficiency as fast as 285 FPS is also obtained.
更多查看译文
关键词
Image understanding, Divergence, Cropping
AI 理解论文
溯源树
样例
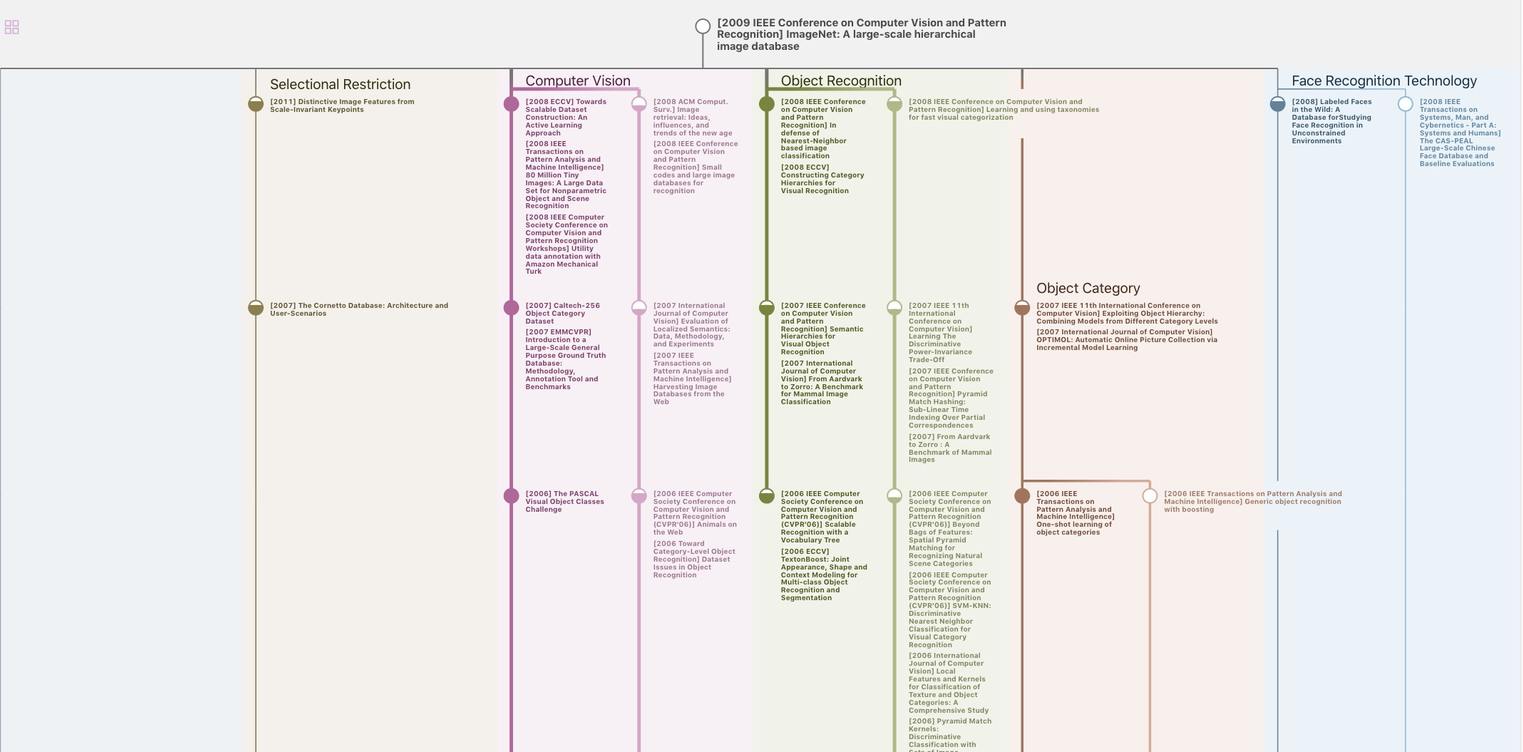
生成溯源树,研究论文发展脉络
Chat Paper
正在生成论文摘要