A clustering-based biased Monte Carlo approach to protein titration curve prediction
2020 19th IEEE International Conference on Machine Learning and Applications (ICMLA)(2020)
摘要
In this work, we developed an efficient approach to compute ensemble averages in systems with pairwise-additive energetic interactions between the entities. Methods involving full enumeration of the configuration space result in exponential complexity. Sampling methods such as Markov Chain Monte Carlo (MCMC) algorithms have been proposed to tackle the exponential complexity of these problems; however, in certain scenarios where significant energetic coupling exists between the entities, the efficiency of the such algorithms can be diminished. We used a strategy to improve the efficiency of MCMC by taking advantage of the cluster structure in the interaction energy matrix to bias the sampling. We pursued two different schemes for the biased MCMC runs and show that they are valid MCMC schemes. We used both synthesized and real-world systems to show the improved performance of our biased MCMC methods when compared to the regular MCMC method. In particular, we applied these algorithms to the problem of estimating protonation ensemble averages and titration curves of residues in a protein.
更多查看译文
关键词
Markov Chain Monte Carlo (MCMC),Ensemble Averages,Energy Minimization,Discrete Optimization,Protein Titration
AI 理解论文
溯源树
样例
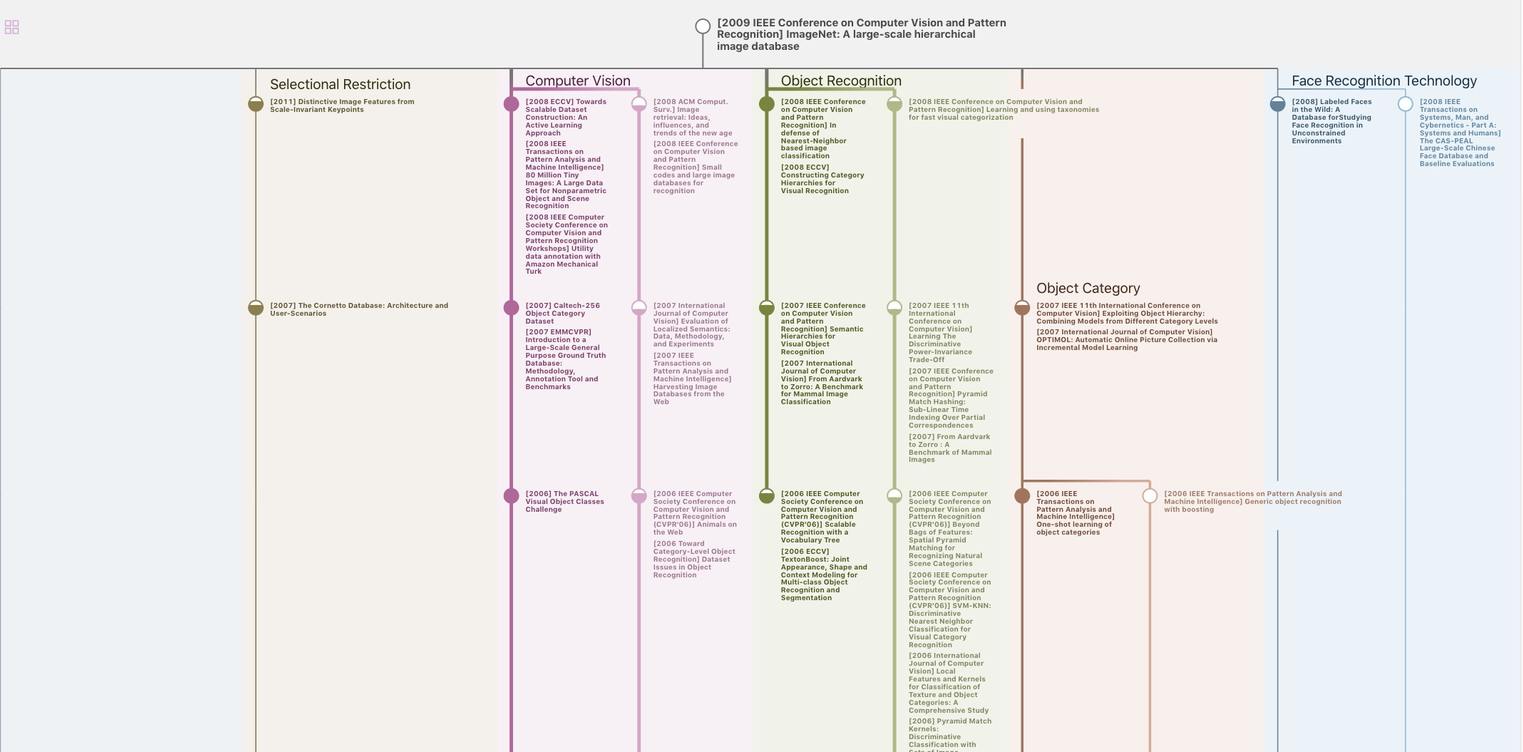
生成溯源树,研究论文发展脉络
Chat Paper
正在生成论文摘要